Iamp-Attenpred: a Novel Antimicrobial Peptide Predictor Based on BERT Feature Extraction Method and CNN-BiLSTM-Attention Combination Model.
Briefings in bioinformatics(2023)
摘要
As a kind of small molecule protein that can fight against various microorganisms in nature, antimicrobial peptides (AMPs) play an indispensable role in maintaining the health of organisms and fortifying defenses against diseases. Nevertheless, experimental approaches for AMP identification still demand substantial allocation of human resources and material inputs. Alternatively, computing approaches can assist researchers effectively and promptly predict AMPs. In this study, we present a novel AMP predictor called iAMP-Attenpred. As far as we know, this is the first work that not only employs the popular BERT model in the field of natural language processing (NLP) for AMPs feature encoding, but also utilizes the idea of combining multiple models to discover AMPs. Firstly, we treat each amino acid from preprocessed AMPs and non-AMP sequences as a word, and then input it into BERT pre-training model for feature extraction. Moreover, the features obtained from BERT method are fed to a composite model composed of one-dimensional CNN, BiLSTM and attention mechanism for better discriminating features. Finally, a flatten layer and various fully connected layers are utilized for the final classification of AMPs. Experimental results reveal that, compared with the existing predictors, our iAMP-Attenpred predictor achieves better performance indicators, such as accuracy, precision and so on. This further demonstrates that using the BERT approach to capture effective feature information of peptide sequences and combining multiple deep learning models are effective and meaningful for predicting AMPs.
更多查看译文
关键词
antimicrobial peptides,BERT,CNN-BiLSTM-Attention,AMP predictor
AI 理解论文
溯源树
样例
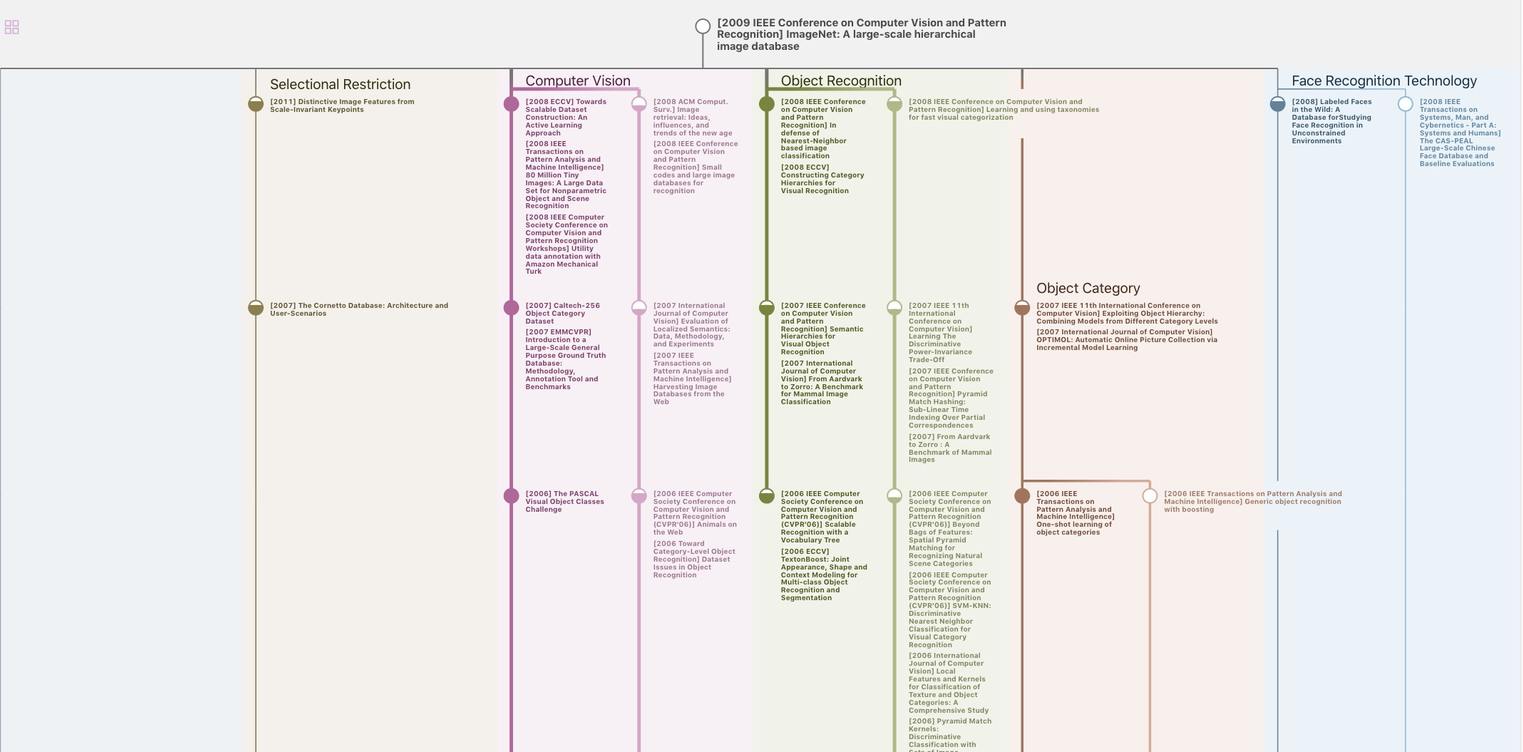
生成溯源树,研究论文发展脉络
Chat Paper
正在生成论文摘要