Physics-informed digital twin for wind turbine main bearing fatigue: Quantifying uncertainty in grease degradation
APPLIED SOFT COMPUTING(2023)
摘要
In the field of prognostics and health management for industrial equipment, digital twins stand out as essential tools. Wind park operators can harness the potential of digital twins to monitor component health, enabling proactive measures to optimize energy production while reducing maintenance costs. This study introduces a novel hybrid digital twin application tailored for monitoring wind turbine main bearing fatigue. It combines physics-based and data-driven kernels to address variable grease quality and biased observations. Our study covers model initialization, cross-validation, fleet management, and the influence of sampled turbines on prediction. Our approach offers two key advantages: (a) It reduces the need for extensive datasets, typical in other machine learning methods, by incorporating physics-based knowledge into the network architecture, and (b) it quantifies output uncertainties using tailored network layers and loss functions. Our findings conclude that even under compound uncertainty scenario, our hybrid model can estimate fleet unreliability only off by 4.7 weeks. However, it is essential to note that computational costs are tied to data processing, similar to other recurrent neural networks. A limitation is that the physics-based kernels must align with common machine learning linear algebra practices.
更多查看译文
关键词
Physics-informed neural network,Wind turbines,Digital twins,Uncertainty quantification
AI 理解论文
溯源树
样例
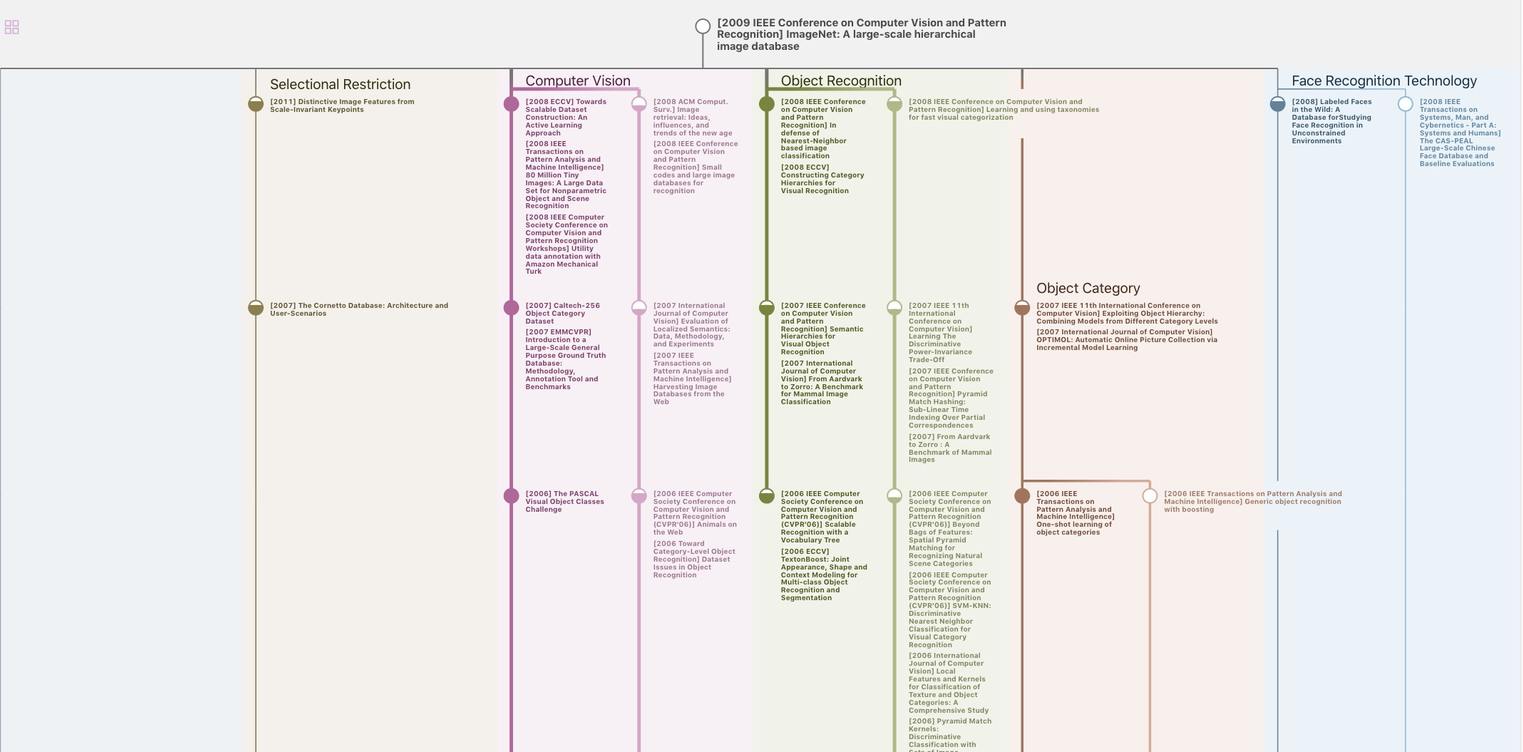
生成溯源树,研究论文发展脉络
Chat Paper
正在生成论文摘要