Evaluating feature attribution methods in the image domain
Machine Learning(2024)
Abstract
Feature attribution maps are a popular approach to highlight the most important pixels in an image for a given prediction of a model. Despite a recent growth in popularity and available methods, the objective evaluation of such attribution maps remains an open problem. Building on previous work in this domain, we investigate existing quality metrics and propose new variants of metrics for the evaluation of attribution maps. We confirm a recent finding that different quality metrics seem to measure different underlying properties of attribution maps, and extend this finding to a larger selection of attribution methods, quality metrics, and datasets. We also find that metric results on one dataset do not necessarily generalize to other datasets, and methods with desirable theoretical properties do not necessarily outperform computationally cheaper alternatives in practice. Based on these findings, we propose a general benchmarking approach to help guide the selection of attribution methods for a given use case. Implementations of attribution metrics and our experiments are available online ( https://github.com/arnegevaert/benchmark-general-imaging ).
MoreTranslated text
Key words
Explainability,Interpretability,Benchmark,Feature attribution,Saliency maps
AI Read Science
Must-Reading Tree
Example
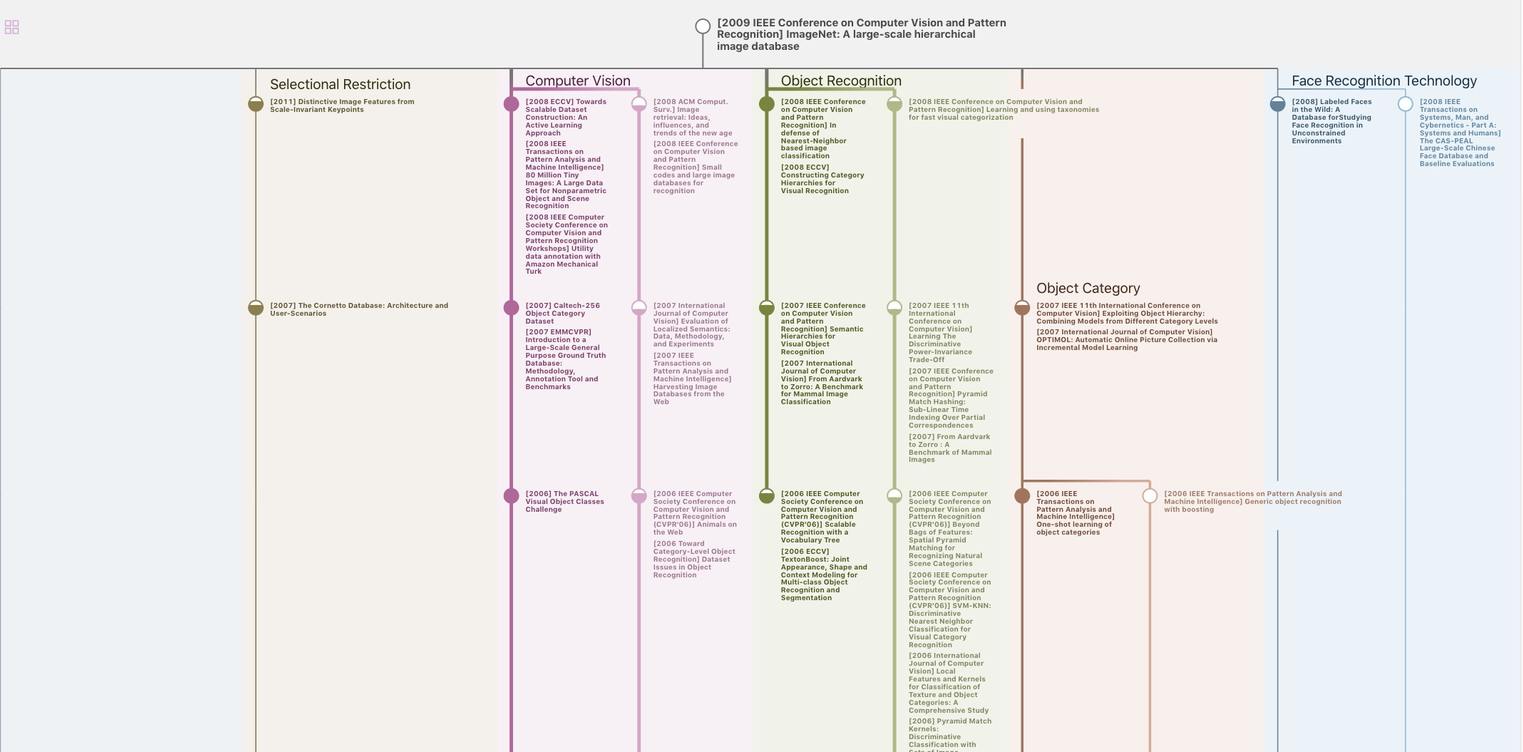
Generate MRT to find the research sequence of this paper
Chat Paper
Summary is being generated by the instructions you defined