Calibrated inference: statistical inference that accounts for both sampling uncertainty and distributional uncertainty
arxiv(2023)
摘要
How can we draw trustworthy scientific conclusions? One criterion is that a study can be replicated by independent teams. While replication is critically important, it is arguably insufficient. If a study is biased for some reason and other studies recapitulate the approach then findings might be consistently incorrect. It has been argued that trustworthy scientific conclusions require disparate sources of evidence. However, different methods might have shared biases, making it difficult to judge the trustworthiness of a result. We formalize this issue by introducing a "distributional uncertainty model", which captures biases in the data collection process. Distributional uncertainty is related to other concepts in statistics, ranging from correlated data to selection bias and confounding. We show that a stability analysis on a single data set allows to construct confidence intervals that account for both sampling uncertainty and distributional uncertainty.
更多查看译文
关键词
distributional uncertainty,statistical inference
AI 理解论文
溯源树
样例
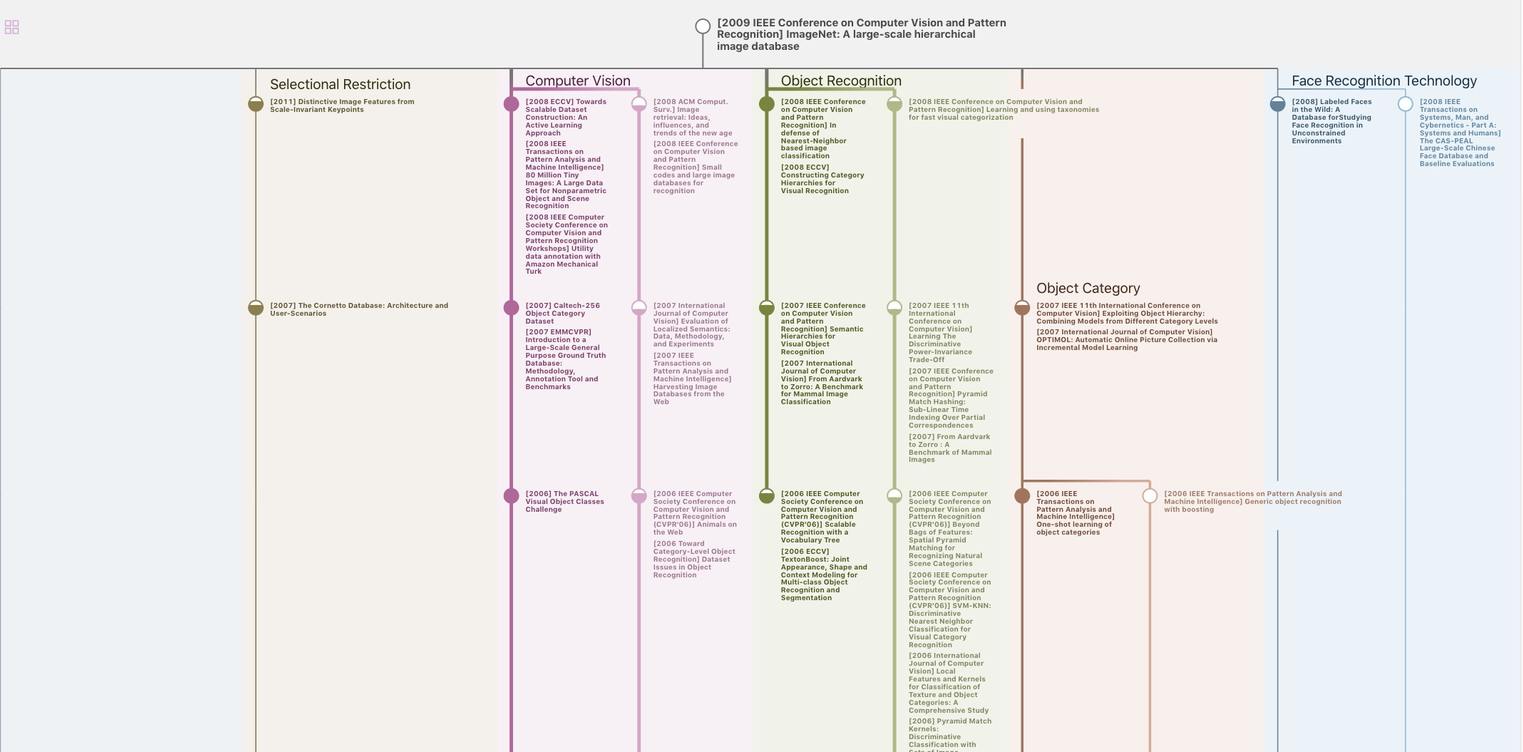
生成溯源树,研究论文发展脉络
Chat Paper
正在生成论文摘要