Using Bayesian Deep Learning to Infer Planet Mass from Gaps in Protoplanetary Disks
ASTROPHYSICAL JOURNAL(2022)
摘要
Planet-induced substructures, like annular gaps, observed in dust emission from protoplanetary disks, provide a unique probe for characterizing unseen young planets. While deep-learning-based models have an edge in characterizing a planet's properties over traditional methods, such as customized simulations and empirical relations, they lacks the ability to quantify the uncertainties associated with their predictions. In this paper, we introduce a Bayesian deep-learning network, "DPNNet-Bayesian," which can predict planet mass from disk gaps and also provides the uncertainties associated with the prediction. A unique feature of our approach is that it is able to distinguish between the uncertainty associated with the deep-learning architecture and the uncertainty inherent in the input data due to measurement noise. The model is trained on a data set generated from disk-planet simulations using the fargo3d hydrodynamics code, with a newly implemented fixed grain size module and improved initial conditions. The Bayesian framework enables the estimation of a gauge/confidence interval over the validity of the prediction, when applied to unknown observations. As a proof of concept, we apply DPNNet-Bayesian to the dust gaps observed in HL Tau. The network predicts masses of 86.0 +/- 5.5 M (circle plus), 43.8 +/- 3.3 M (circle plus), and 92.2 +/- 5.1 M (circle plus), respectively, which are comparable to those from other studies based on specialized simulations.
更多查看译文
关键词
infer planet mass,protoplanetary disks,bayesian deep learning
AI 理解论文
溯源树
样例
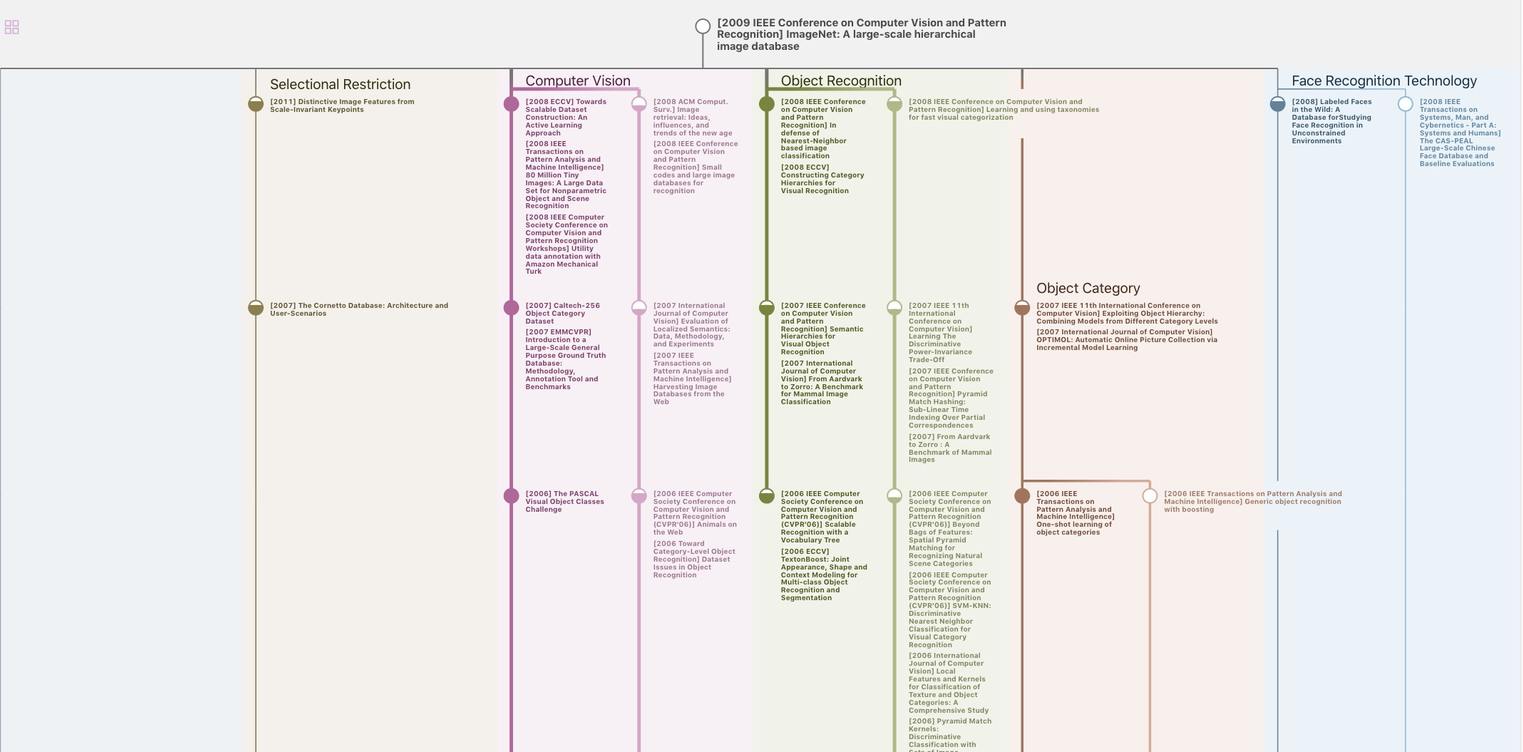
生成溯源树,研究论文发展脉络
Chat Paper
正在生成论文摘要