A Framework for Eliciting, Incorporating, and Disciplining Identification Beliefs in Linear Models, Third Version
SSRN Electronic Journal(2015)
摘要
The identification of causal effects in linear models relies, explicitly and implicitly, on the imposition of researcher beliefs along several dimensions. Assumptions about measurement error, regressor endogeneity, and instrument validity are three key components of any such empirical exercise. Although in practice researchers reason about these three dimensions independently, we show that measurement error, regressor endogeneity and instrument invalidity are mutually constrained by each other and the data in a manner that is only apparent by writing down the full identified set for the model. The nature of this set makes it clear that researcher beliefs over these objects cannot and indeed should not be independent: there are fewer degrees of freedom than parameters. By failing to take this into account, applied researchers both leave money on the table - by failing to incorporate relevant information in estimation - and more importantly risk reasoning to a contradiction by expressing mutually incompatible beliefs. We propose a Bayesian framework to help researchers elicit their beliefs, explicitly incorporate them into estimation and ensure that they are mutually coherent. We illustrate the practical usefulness of our method by applying it to several well-known papers from the empirical microeconomics literature.
更多查看译文
关键词
disciplining identification beliefs,linear models,eliciting
AI 理解论文
溯源树
样例
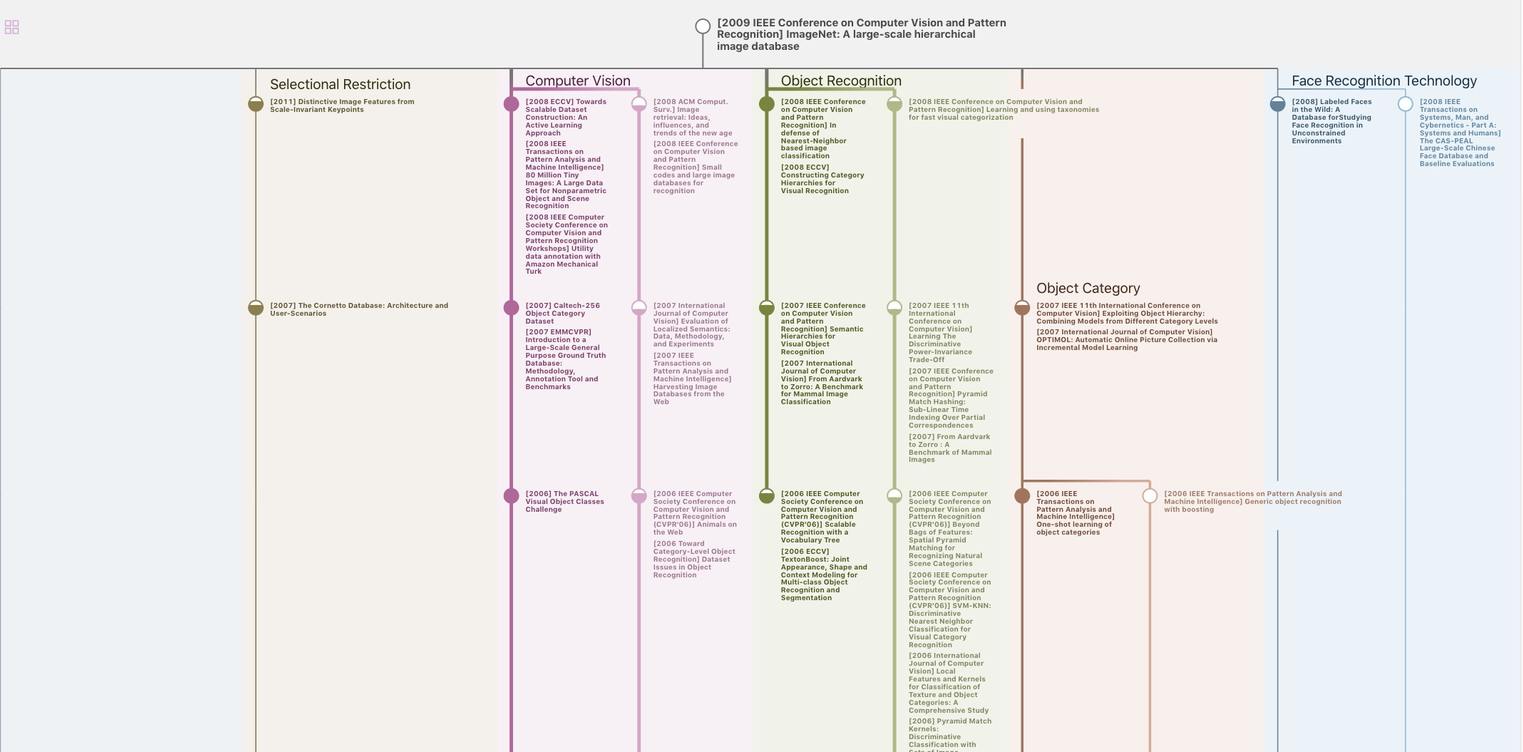
生成溯源树,研究论文发展脉络
Chat Paper
正在生成论文摘要