Approximate Counting of Graphical Models Via MCMC Revisited
International Journal of Intelligent Systems(2014)
AI Read Science
Must-Reading Tree
Example
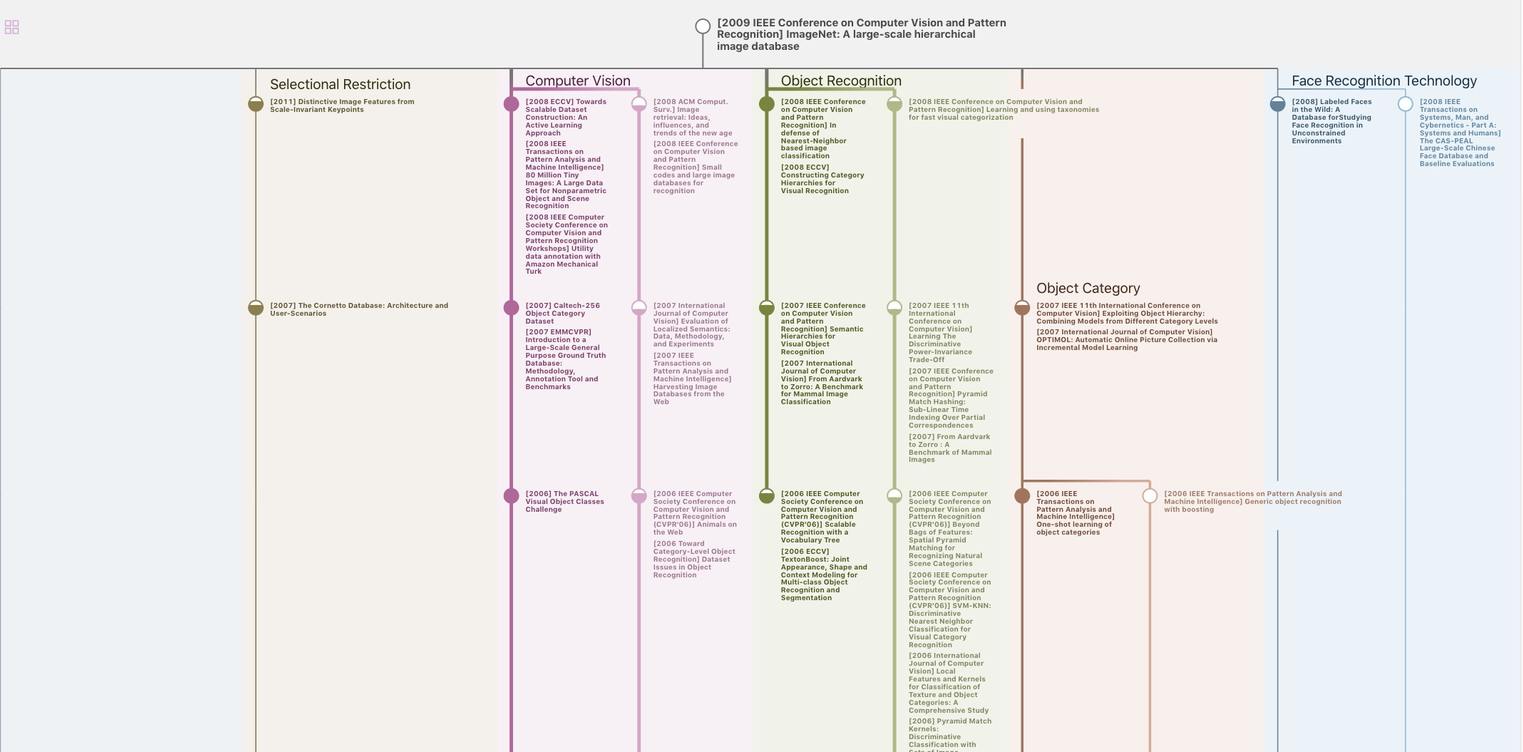
Generate MRT to find the research sequence of this paper
Chat Paper
Summary is being generated by the instructions you defined