Using Deep Reinforcement Learning with Automatic Curriculum earning for Mapless Navigation in Intralogistics
arxiv(2022)
摘要
We propose a deep reinforcement learning approach for solving a mapless navigation problem in warehouse scenarios. The automatic guided vehicle is equipped with LiDAR and frontal RGB sensors and learns to reach underneath the target dolly. The challenges reside in the sparseness of positive samples for learning, multi-modal sensor perception with partial observability, the demand for accurate steering maneuvers together with long training cycles. To address these points, we proposed NavACL-Q as an automatic curriculum learning together with distributed soft actor-critic. The performance of the learning algorithm is evaluated exhaustively in a different warehouse environment to check both robustness and generalizability of the learned policy. Results in NVIDIA Isaac Sim demonstrates that our trained agent significantly outperforms the map-based navigation pipeline provided by NVIDIA Isaac Sim in terms of higher agent-goal distances and relative orientations. The ablation studies also confirmed that NavACL-Q greatly facilitates the whole learning process and a pre-trained feature extractor manifestly boosts the training speed.
更多查看译文
关键词
deep reinforcement learning, automatic curriculum learning, autonomous navigation, multi-modal sensor perception
AI 理解论文
溯源树
样例
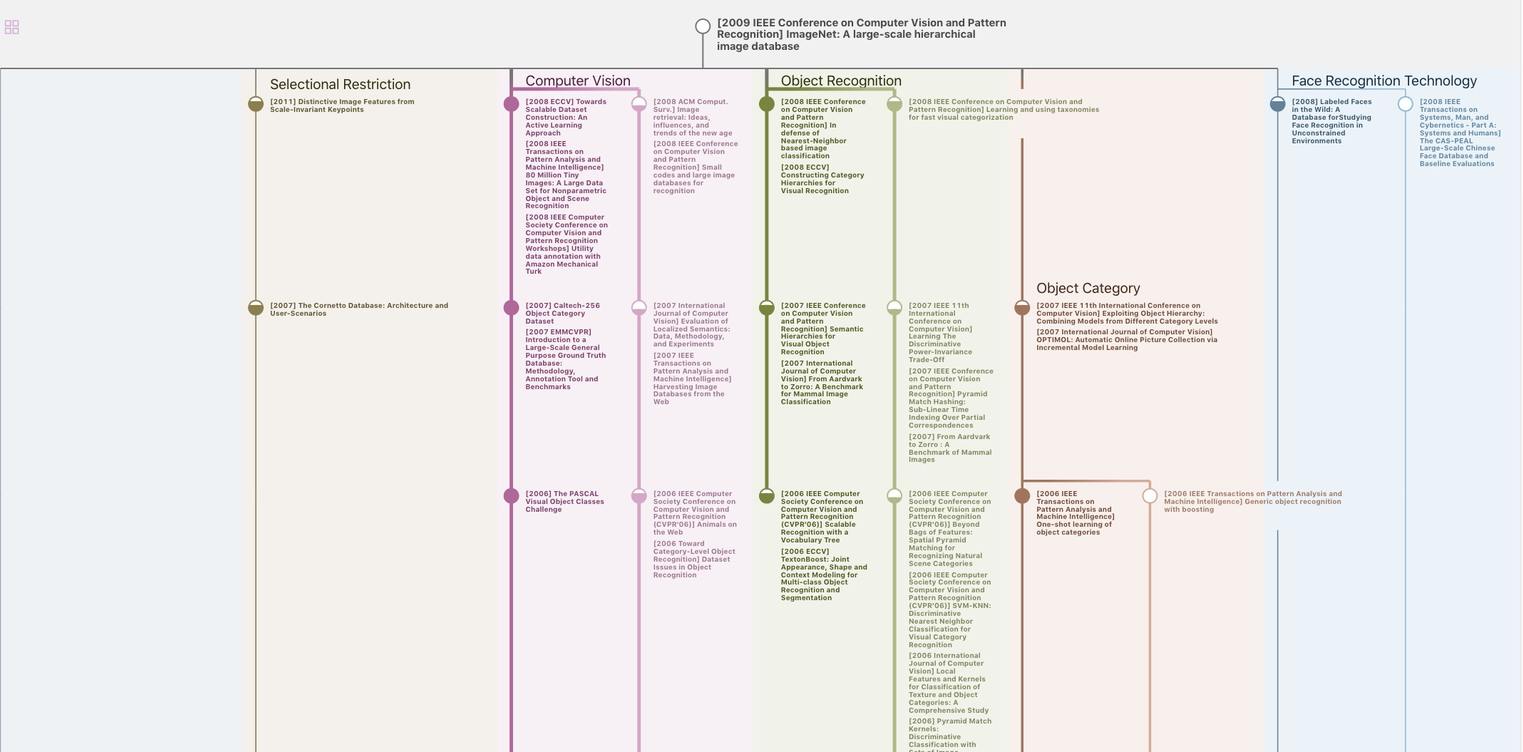
生成溯源树,研究论文发展脉络
Chat Paper
正在生成论文摘要