A bias-adjusted estimator in quantile regression for clustered data
Econometrics and Statistics(2022)
Abstract
Quantile regression models with random effects are useful for studying associations between covariates and quantiles of the response distribution for clustered data. Parameter estimation is examined for a class of mixed-effects quantile regression models, with focus on settings with many but small clusters. The main contributions are the following: (i) documenting that existing methods may lead to severely biased estimators for fixed effects parameters; (ii) proposing a new two-step estimation methodology where predictions of the random effects are first computed by a pseudo likelihood approach (the LQMM method) and then used as offsets in standard quantile regression; (iii) proposing a novel bootstrap sampling procedure in order to reduce bias of the two-step estimator and compute confidence intervals. The proposed estimation and associated inference is assessed numerically through rigorous simulation studies and applied to an AIDS Clinical Trial Group (ACTG) study.
MoreTranslated text
Key words
Linear quantile regression,Clustered data,Random effects,Bias-adjustment,Wild bootstrap,AIDS clinical trial group study
AI Read Science
Must-Reading Tree
Example
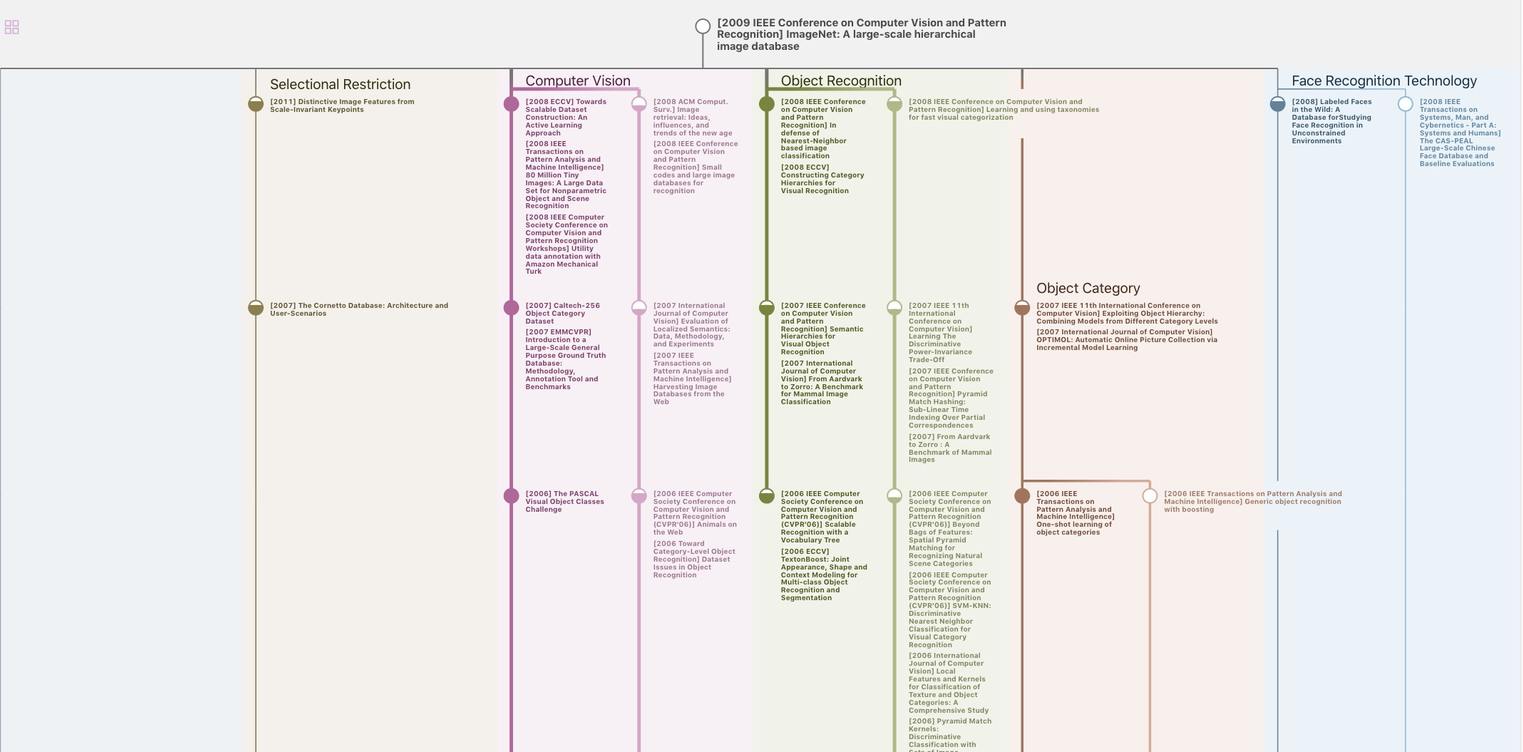
Generate MRT to find the research sequence of this paper
Chat Paper
Summary is being generated by the instructions you defined