Scalable Query Answering Under Uncertainty to Neuroscientific Ontological Knowledge: The NeuroLang Approach
Neuroinformatics(2022)
摘要
Researchers in neuroscience have a growing number of datasets available to study the brain, which is made possible by recent technological advances. Given the extent to which the brain has been studied, there is also available ontological knowledge encoding the current state of the art regarding its different areas, activation patterns, keywords associated with studies, etc. Furthermore, there is inherent uncertainty associated with brain scans arising from the mapping between voxels—3D pixels—and actual points in different individual brains. Unfortunately, there is currently no unifying framework for accessing such collections of rich heterogeneous data under uncertainty, making it necessary for researchers to rely on ad hoc tools. In particular, one major weakness of current tools that attempt to address this task is that only very limited propositional query languages have been developed. In this paper we present NeuroLang, a probabilistic language based on first-order logic with existential rules, probabilistic uncertainty, ontologies integration under the open world assumption, and built-in mechanisms to guarantee tractable query answering over very large datasets. NeuroLang’s primary objective is to provide a unified framework to seamlessly integrate heterogeneous data, such as ontologies, and map fine-grained cognitive domains to brain regions through a set of formal criteria, promoting shareable and highly reproducible research. After presenting the language and its general query answering architecture, we discuss real-world use cases showing how NeuroLang can be applied to practical scenarios.
更多查看译文
关键词
Datalog,Meta-Analysis,Neuroimaging,Open-world Assumption,Probabilistic Programming,Query Answering
AI 理解论文
溯源树
样例
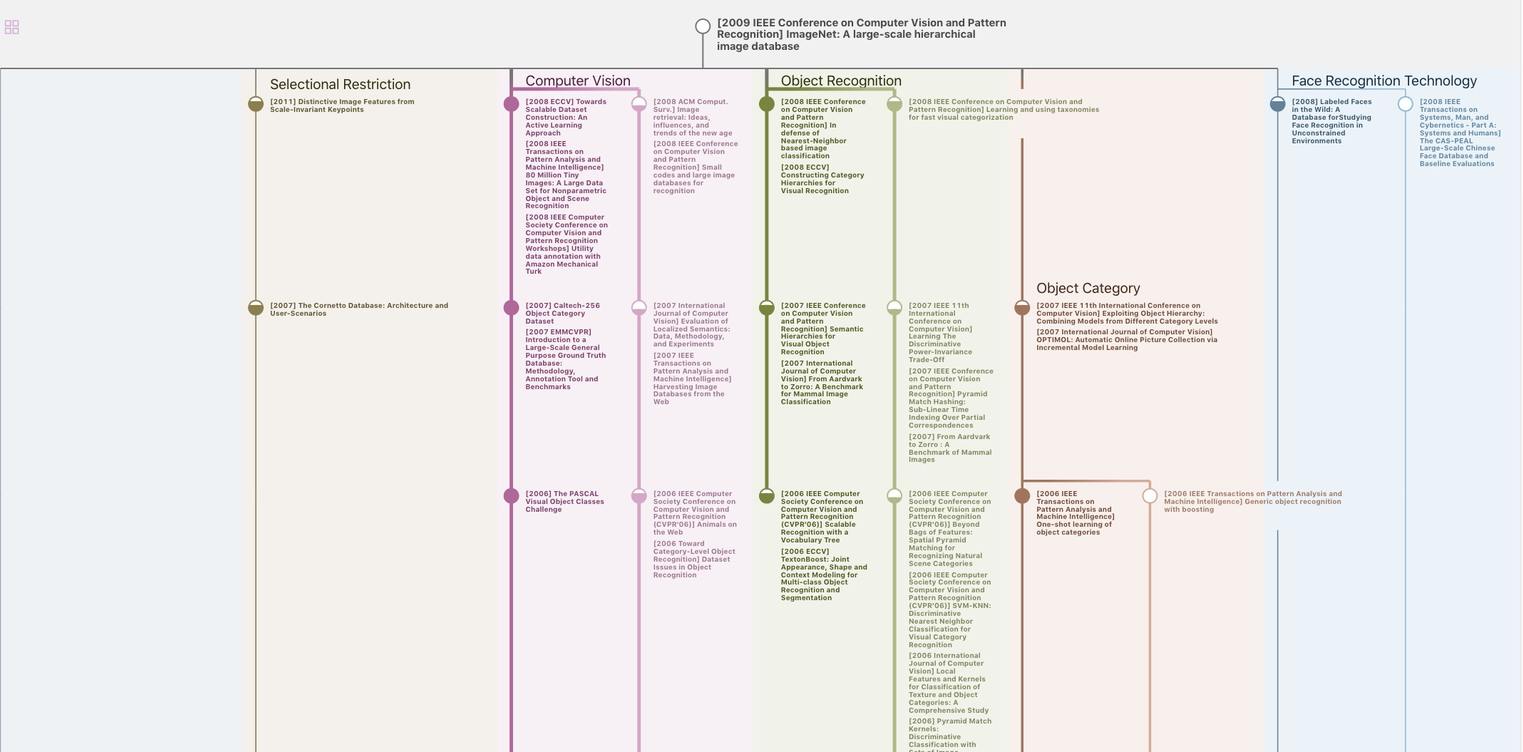
生成溯源树,研究论文发展脉络
Chat Paper
正在生成论文摘要