Convergence of Oja's online principal component flow
arXiv (Cornell University)(2022)
摘要
Online principal component analysis (PCA) has been an efficient tool in practice to reduce dimension. However, convergence properties of the corresponding ODE are still unknown, including global convergence, stable manifolds, and convergence rate. In this paper, we focus on the stochastic gradient ascent (SGA) method proposed by Oja. By regarding the corresponding ODE as a Landau-Lifshitz-Gilbert (LLG) equation on the Stiefel manifold, we proved global convergence of the ODE. Moreover, we developed a new technique to determine stable manifolds. This technique analyzes the rank of the initial datum. Using this technique, we derived the explicit expression of the stable manifolds. As a consequence, exponential convergence to stable equilibrium points was also proved. The success of this new technique should be attributed to the semi-decoupling property of the SGA method: iteration of previous components does not depend on that of later ones. As far as we know, our result is the first complete one on the convergence of an online PCA flow, providing global convergence, explicit characterization of stable manifolds, and closed formula of exponential convergence depending on the spectrum gap.
更多查看译文
关键词
oja,flow
AI 理解论文
溯源树
样例
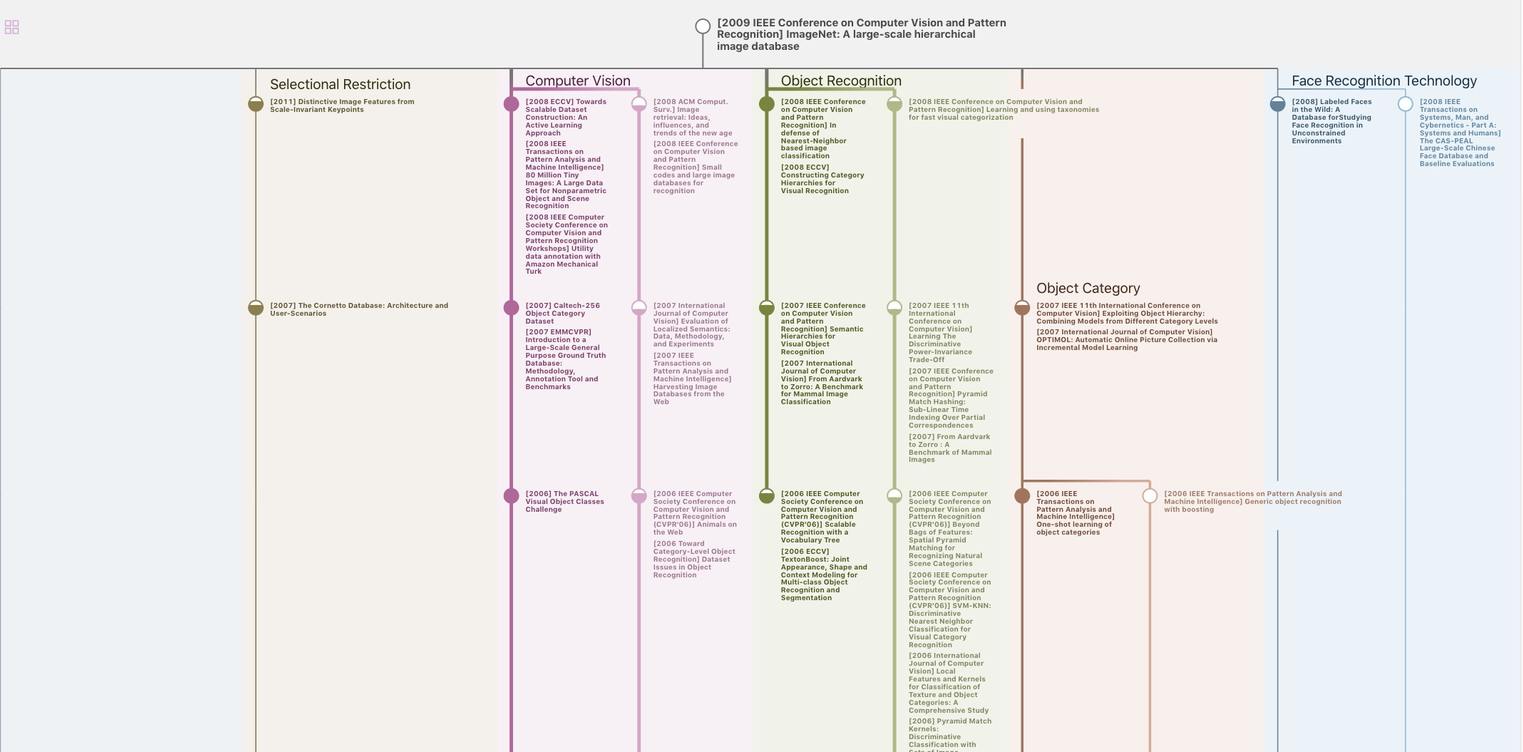
生成溯源树,研究论文发展脉络
Chat Paper
正在生成论文摘要