Knowledge Augmented Bert Mutual Network in Multi-Turn Spoken Dialogues
IEEE International Conference on Acoustics, Speech, and Signal Processing (ICASSP)(2022)
摘要
Modern spoken language understanding (SLU) systems rely on sophisticated semantic notions revealed in single utterances to detect intents and slots. However, they lack the capability of modeling multi-turn dynamics within a dialogue particularly in long-term slot contexts. Without external knowledge, depending on limited linguistic legitimacy within a word sequence may overlook deep semantic information across dialogue turns. In this paper, we propose to equip a BERT-based joint model with a knowledge attention module to mutually leverage dialogue contexts between two SLU tasks. A gating mechanism is further utilized to filter out irrelevant knowledge triples and to circumvent distracting comprehension. Experimental results in two complicated multi-turn dialogue datasets have demonstrate by mutually modeling two SLU tasks with filtered knowledge and dialogue contexts, our approach has considerable improvements compared with several competitive baselines.
更多查看译文
关键词
Multi-turn Dialogues,Slot Filling,Knowledge base,BERT,Context
AI 理解论文
溯源树
样例
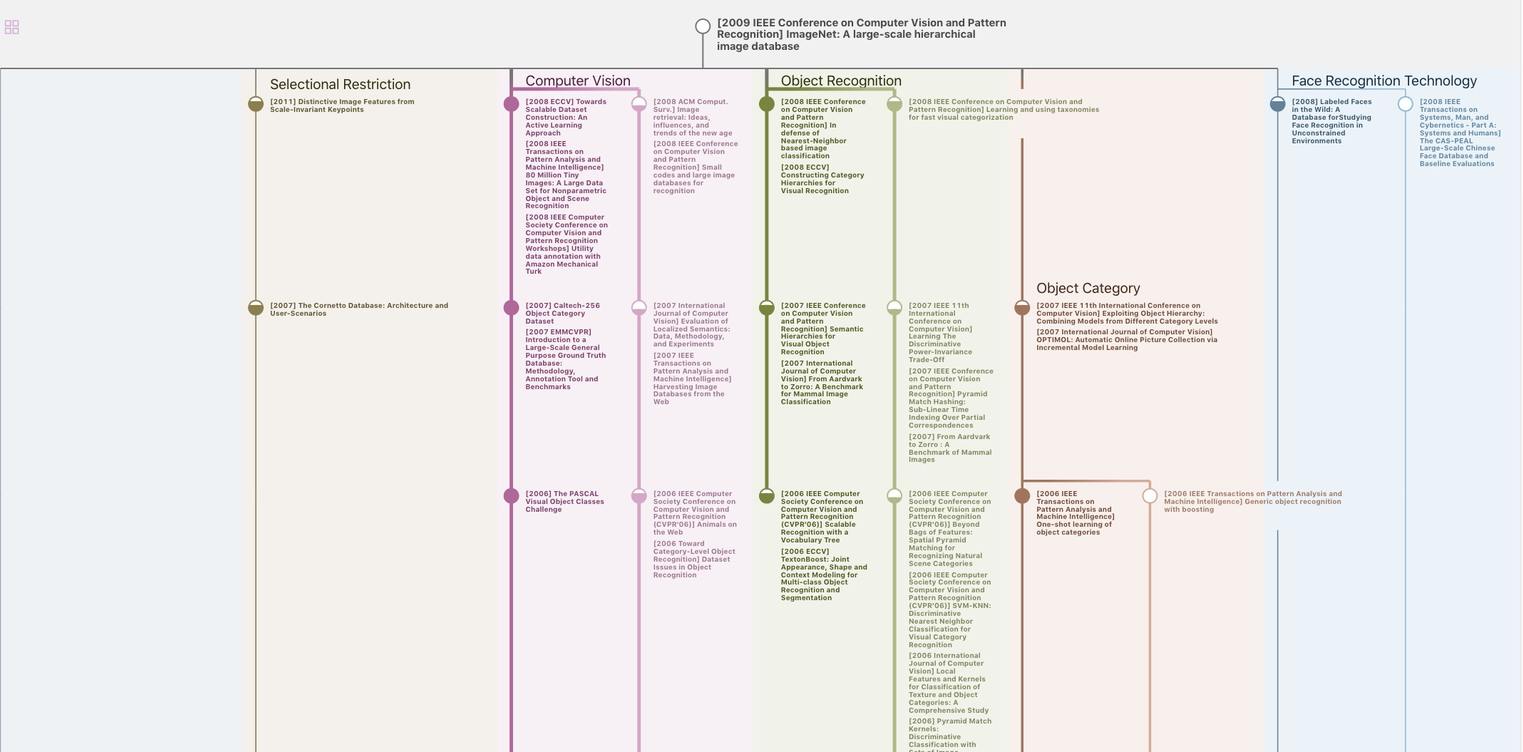
生成溯源树,研究论文发展脉络
Chat Paper
正在生成论文摘要