Robust Hierarchical Patterns for identifying MDD patients: A Multisite Study
arxiv(2022)
摘要
Many supervised machine learning frameworks have been proposed for disease classification using functional magnetic resonance imaging (fMRI) data, producing important biomarkers. More recently, data pooling has flourished, making the result generalizable across a large population. But, this success depends on the population diversity and variability introduced due to the pooling of the data that is not a primary research interest. Here, we look at hierarchical Sparse Connectivity Patterns (hSCPs) as biomarkers for major depressive disorder (MDD). We propose a novel model based on hSCPs to predict MDD patients from functional connectivity matrices extracted from resting-state fMRI data. Our model consists of three coupled terms. The first term decomposes connectivity matrices into hierarchical low-rank sparse components corresponding to synchronous patterns across the human brain. These components are then combined via patient-specific weights capturing heterogeneity in the data. The second term is a classification loss that uses the patient-specific weights to classify MDD patients from healthy ones. Both of these terms are combined with the third term, a robustness loss function to improve the reproducibility of hSCPs. This reduces the variability introduced due to site and population diversity (age and sex) on the predictive accuracy and pattern stability in a large dataset pooled from five different sites. Our results show the impact of diversity on prediction performance. Our model can reduce diversity and improve the predictive and generalizing capability of the components. Finally, our results show that our proposed model can robustly identify clinically relevant patterns characteristic of MDD with high reproducibility.
更多查看译文
关键词
mdd patients,robust hierarchical patterns,identifying
AI 理解论文
溯源树
样例
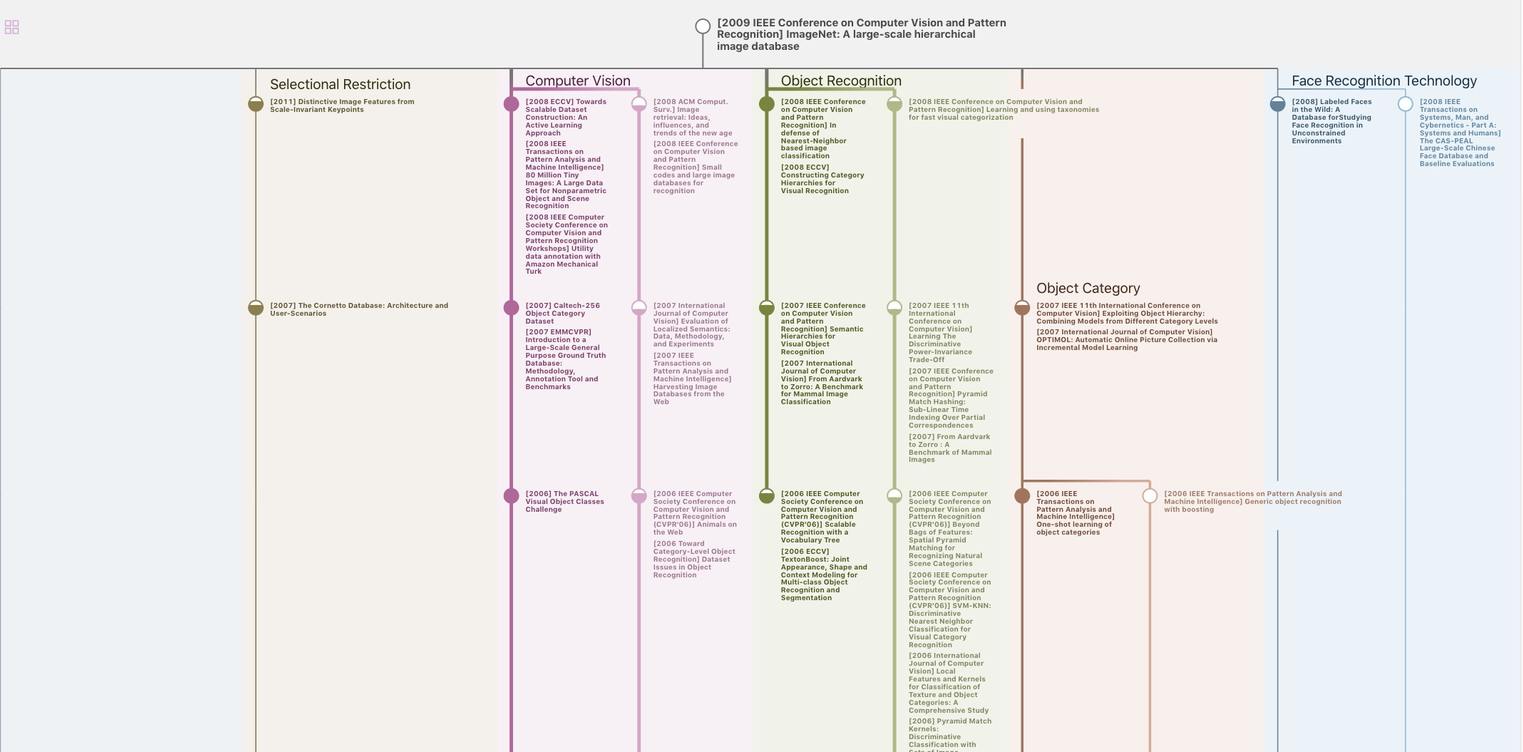
生成溯源树,研究论文发展脉络
Chat Paper
正在生成论文摘要