Model Selection-inspired Coefficients Optimization for Polynomial-Kernel Graph Learning
2021 Asia-Pacific Signal and Information Processing Association Annual Summit and Conference (APSIPA ASC)(2021)
摘要
Graph learning has been extensively investigated for over a decade, in which the graph structure can be learnt from multiple graph signals (e.g., graphical Lasso) or node features (e.g., graph metric learning). Given partial graph signals, existing node feature-based graph learning approaches learn a pair-wise distance metric with gradient descent, where the number of opti-mization variables dramatically scale with the node feature size. To address this issue, in this paper, we propose a low-complexity model selection-inspired graph learning (MSGL) method with very few optimization variables independent with feature size, via leveraging on recent advances in graph spectral signal processing (GSP). We achieve this by 1) interpreting a finite-degree polynomial function of the graph Laplacian as a positive-definite precision matrix, 2) formulating a convex optimization problem with variables being the polynomial coefficients, 3) replacing the positive-definite cone constraint for the precision matrix with a set of linear constraints, and 4) solving efficiently the objective using the Frank-Wolfe algorithm. Using binary classification as an application example, our simulation results show that our proposed MSGL method achieves competitive performance with significant speed gains against existing node feature-based graph learning methods.
更多查看译文
关键词
Graph signal processing,graph learning,convex optimization
AI 理解论文
溯源树
样例
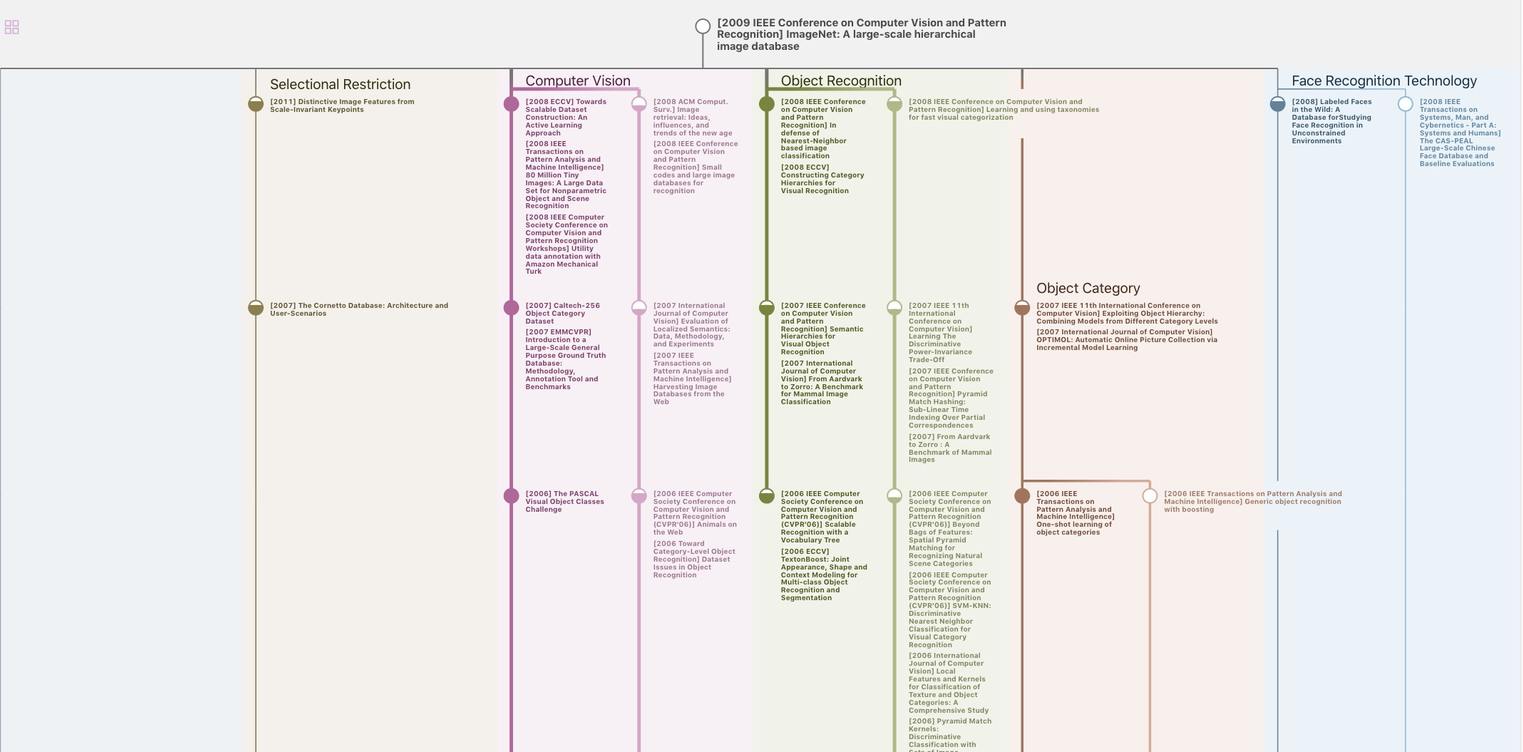
生成溯源树,研究论文发展脉络
Chat Paper
正在生成论文摘要