Attention EdgeConv For 3D Point Cloud Classification
2021 ASIA-PACIFIC SIGNAL AND INFORMATION PROCESSING ASSOCIATION ANNUAL SUMMIT AND CONFERENCE (APSIPA ASC)(2021)
摘要
Due to the irregular and unordered properties of 3D point cloud data, it is more challenging to extract geometric features between points. In this paper, we propose techniques to improve the ability of capturing point cloud features, so that higher accuracy and better stability of point cloud classification tasks can be achieved. First, we design two attention modules: the Point-wise Attention Module determines the correlation between points, and the Channel-wise Attention Module allows the model to focus on important features with limited resources. With these attention modules, we not only achieve the state-of-the-art accuracy of 93.7% on the ModelNet40 dataset but also reduce the error rates on the ScanObjectNN dataset. Secondly, we propose a guideline to dynamically adjust the size of the KNN. Using the proposed Dynamic-K method, we can significantly increase the accuracy of classification when dealing with low-resolution objects.
更多查看译文
关键词
3D Point Cloud Classification, Attention Mechanism, Deep Neural Network
AI 理解论文
溯源树
样例
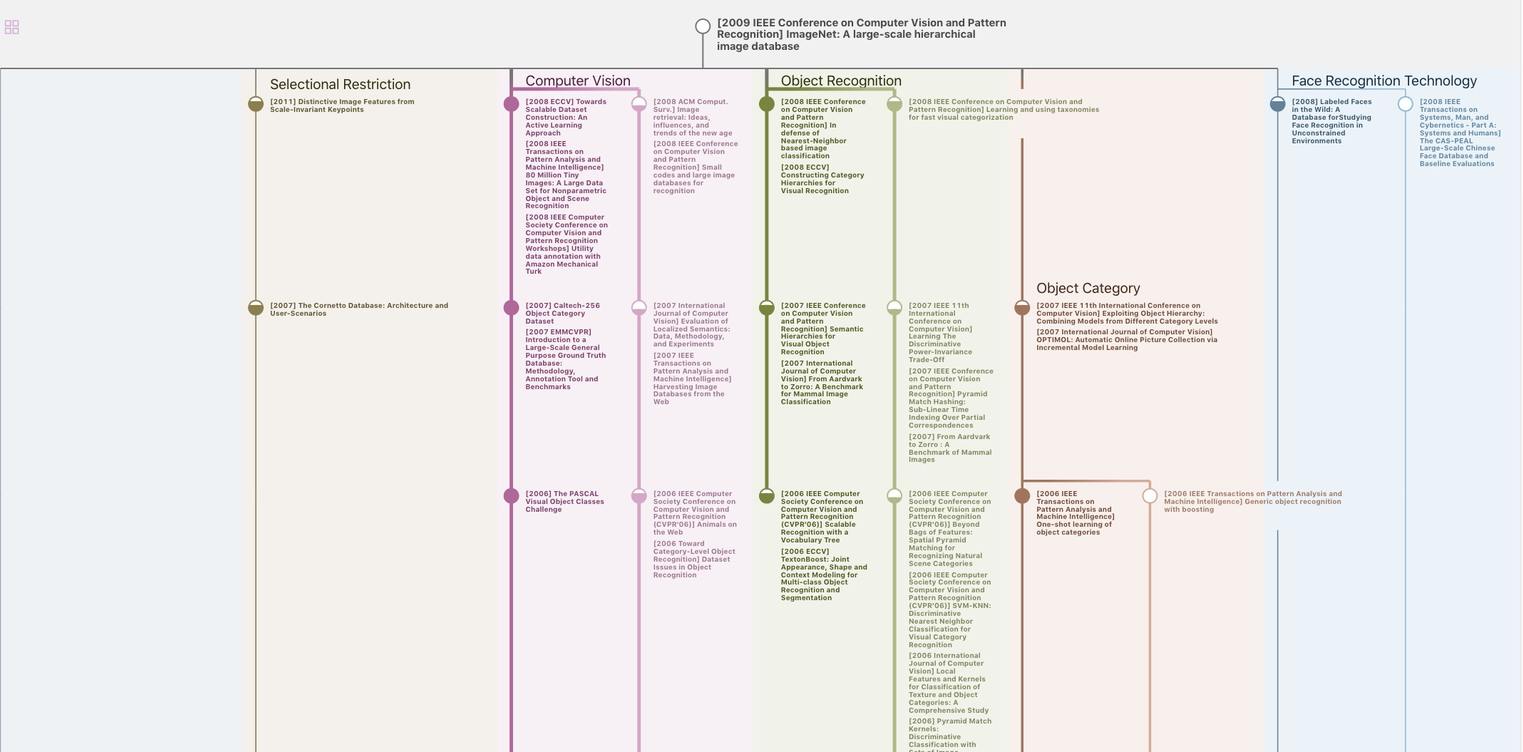
生成溯源树,研究论文发展脉络
Chat Paper
正在生成论文摘要