Data augmentation for asr using tts via a discrete representation
2021 IEEE Automatic Speech Recognition and Understanding Workshop (ASRU)(2021)
Abstract
While end-to-end automatic speech recognition (ASR) has achieved high performance, it requires a huge amount of paired speech and transcription data for training Recently, data augmentation methods have actively been investigated. One method is to use a text-to-speech (TTS) system to generate speech data from text-only data and use the generated speech for data augmentation, but it has been found that the synthesized log Mel-scale filterbank (lmfb) features could have a serious mismatch with the real speech features. In this study, we propose a data augmentation method via a discrete speech representation. The TTS model predicts discrete ID sequences instead of lmfb features, and the ASR also uses the ID sequences as training data. We expect that the use of a discrete representation based on vq-wav2vec not only makes TTS training easier but also mitigates the mismatch with real data. Experimental evaluations show that the proposed method outperforms the data augmentation method using the conventional TTS. We found that it reduces speaker dependency, and the generated features are distributed more closely to the real ones.
MoreTranslated text
Key words
Speech recognition,Sequence-to-sequence model,Data augmentation,Vq-wav2vec,Speech synthesis
AI Read Science
Must-Reading Tree
Example
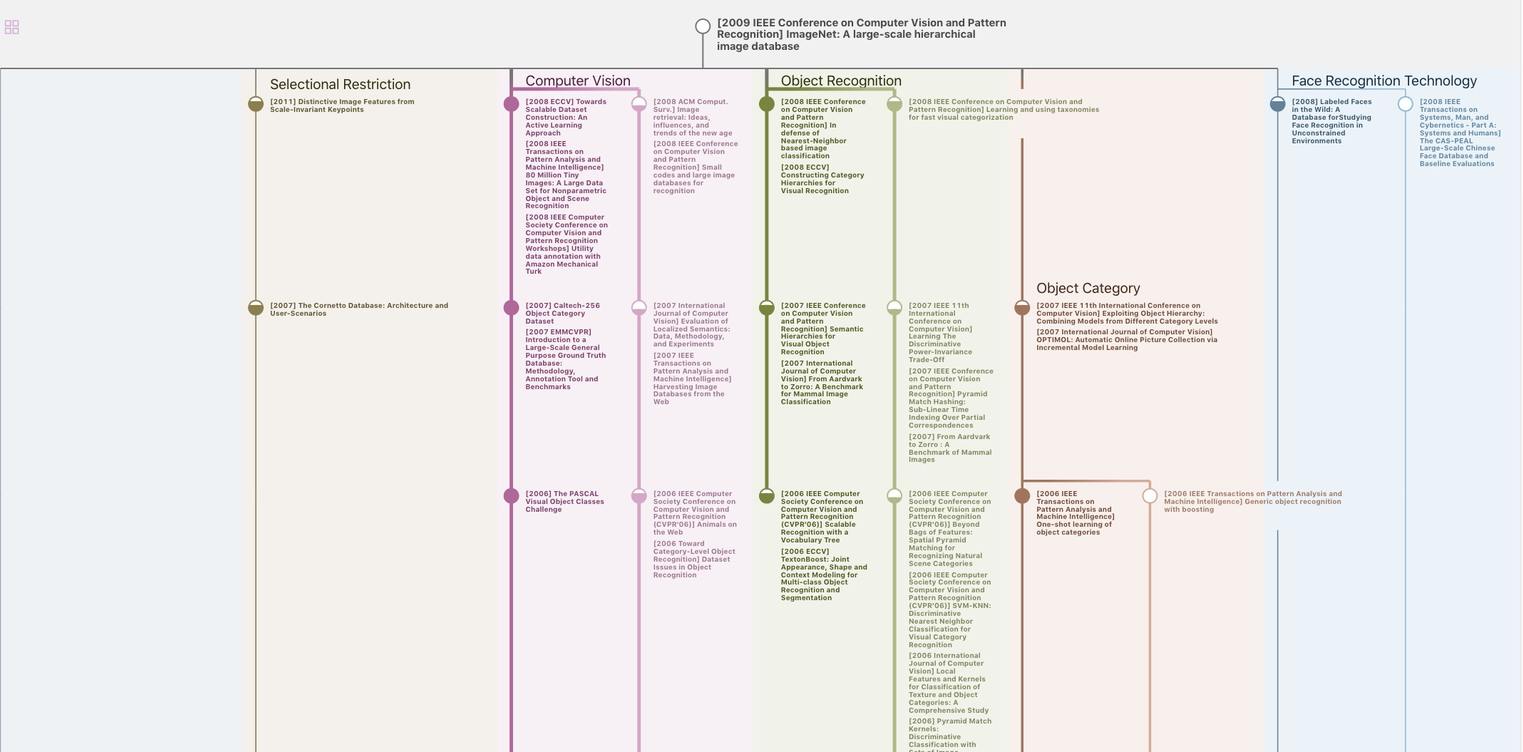
Generate MRT to find the research sequence of this paper
Chat Paper
Summary is being generated by the instructions you defined