Comparative Study of Different Tokenization Strategies for Streaming End-to-End ASR
2021 IEEE Automatic Speech Recognition and Understanding Workshop (ASRU)(2021)
Abstract
Most End-to-End Automatic Speech Recognition (ASR) models use character-based vocabularies: characters, sub-words (BPE), or words. While these work well for training a monolingual model, there are certain limitations when ap-plying these to a multilingual model. Rare characters from character-rich languages like Korean can easily result in large vocabulary size, limiting the model's compactness. Repre-senting text at the level of bytes has also been proposed. However, a byte sequence representation of text is often much longer, which increases the decoding time and makes it computationally expensive for on-device use. Byte-based sub-words (BBPE) are proposed in neural machine translation for word representation but are still unexplored in the ASR domain. In this work, we conduct an empirical study comparing the above three tokenization strategies across three metrics: Word Error Rate (WER), model size, and the decoding time, which are critical for an on-device ASR. We did extensive experiments for both monolingual and bilingual, with languages belonging to same (English and Spanish) and different (English and Korean) language families. Our exper-iments show that BBPE and BPE models yield a similar WER for English and Spanish. While for a character-rich language like Korean, we get 26% and 14% relative WER improvement with BBPE monolingual and bilingual models, respectively. In contrast, the byte models trade-off small model size and a fixed vocabulary at the cost of high xRT. Among all three, we found the BBPE strategy to be the most flexible and optimal for most cases.
MoreTranslated text
Key words
end-to-end speech recognition,multilin-gual,RNN-Transducer
AI Read Science
Must-Reading Tree
Example
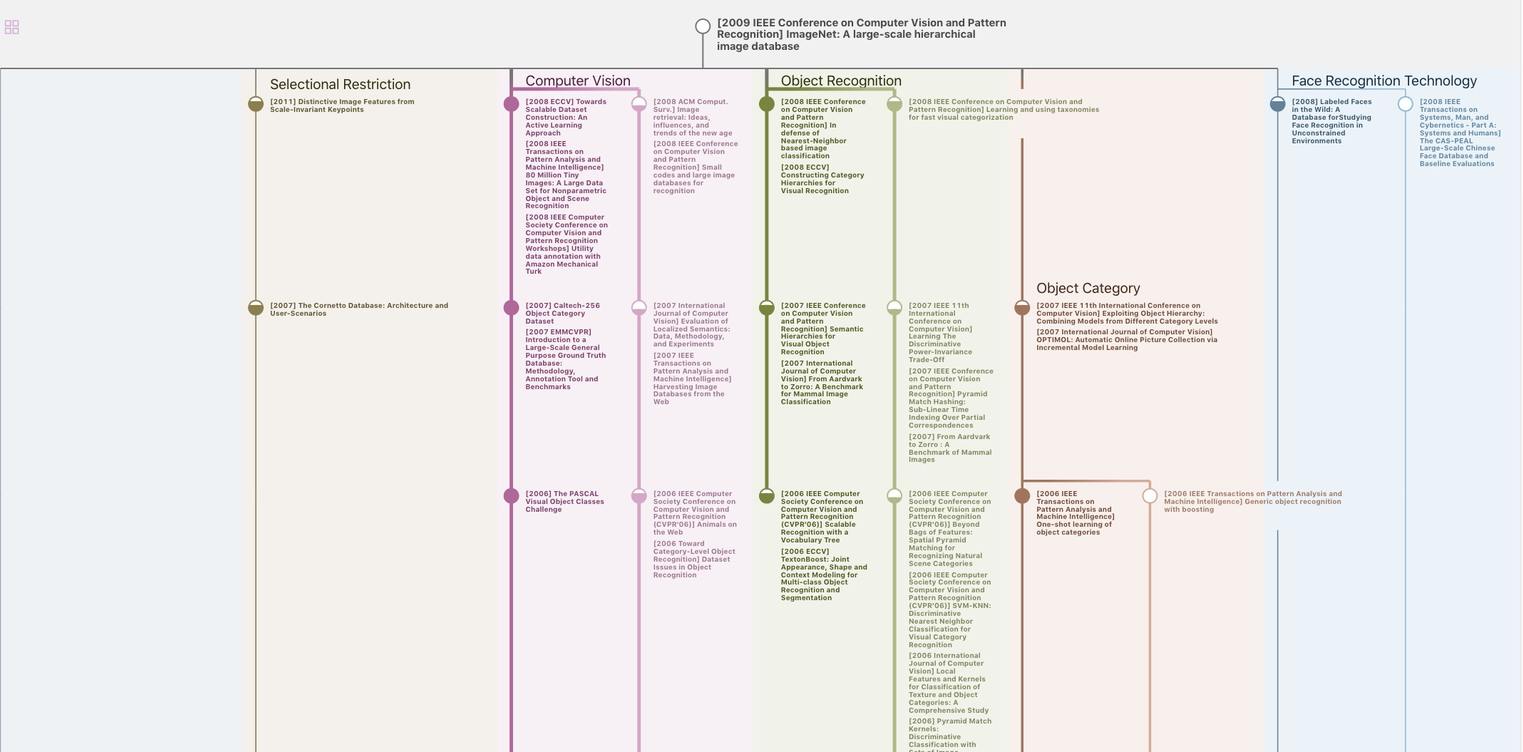
Generate MRT to find the research sequence of this paper
Chat Paper
Summary is being generated by the instructions you defined