CHANNELAUGMENT: IMPROVING GENERALIZATION OF MULTI-CHANNEL ASR BY TRAINING WITH INPUT CHANNEL RANDOMIZATION
2021 IEEE AUTOMATIC SPEECH RECOGNITION AND UNDERSTANDING WORKSHOP (ASRU)(2021)
摘要
End-to-end (E2E) multi-channel ASR systems show state-of-the-art performance in far-field ASR tasks by joint training of a multi-channel front-end along with the ASR model. The main limitation of such systems is that they are usually trained with data from a fixed array geometry, which can lead to degradation in accuracy when a different array is used in testing. This makes it challenging to deploy these systems in practice, as it is costly to retrain and deploy different models for various array configurations. To address this, we present a simple and effective data augmentation technique, which is based on randomly dropping channels in the multi-channel audio input during training, in order to improve the robustness to various array configurations at test time. We call this technique ChannelAugment, in contrast to SpecAugment (SA) which drops time and/or frequency components of a single channel input audio. We apply ChannelAugment to the Spatial Filtering (SF) and Minimum Variance Distortionless Response (MVDR) neural beamforming approaches. For SF, we observe 10.6 % WER improvement across various array configurations employing different numbers of microphones. For MVDR, we achieve a 74% reduction in training time without causing degradation of recognition accuracy.
更多查看译文
关键词
automatic speech recognition, data augmentation, multi-channel, end-to-end training
AI 理解论文
溯源树
样例
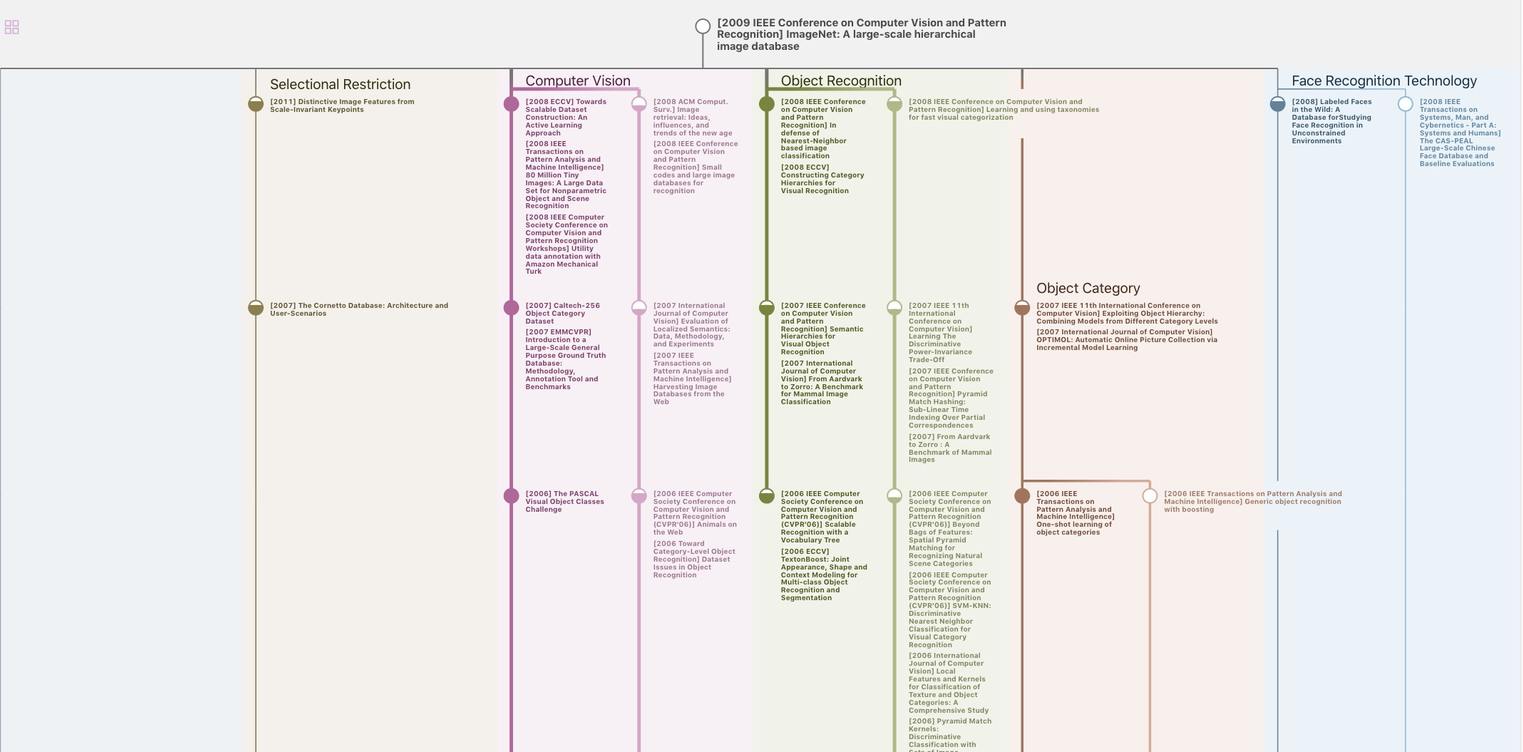
生成溯源树,研究论文发展脉络
Chat Paper
正在生成论文摘要