Parsimonious System Identification from Quantized Observations.
CDC(2021)
摘要
Quantization plays an important role as an interface between analog and digital environments. Since quantization is a many to few mapping, it is a non-linear irreversible process. This made, in addition of the quantization noise signal dependency, the traditional methods of system identification no longer applicable. In this work, we propose a method for parsimonious system identification when only quantized measurements of the output are observable. More precisely, we develop an algorithm that aims at identifying a low order system that is compatible with a priori information on the system and the collected quantized output information. Moreover, the proposed approach can be used even if only fragmented information on the quantized output is available. The proposed algorithm relies on an ADMM approach to l(p) quasi-norm optimization. Numerical results highlight the performance of the proposed approach when compared to the l(1) minimization in terms of the sparsity of the induced solution.
更多查看译文
关键词
parsimonious system identification,quantized observations,digital environments,nonlinear irreversible process,quantization noise signal dependency,quantized measurements,low order system,collected quantized output information,analog environments,ADMM approach,ℓ1 minimization
AI 理解论文
溯源树
样例
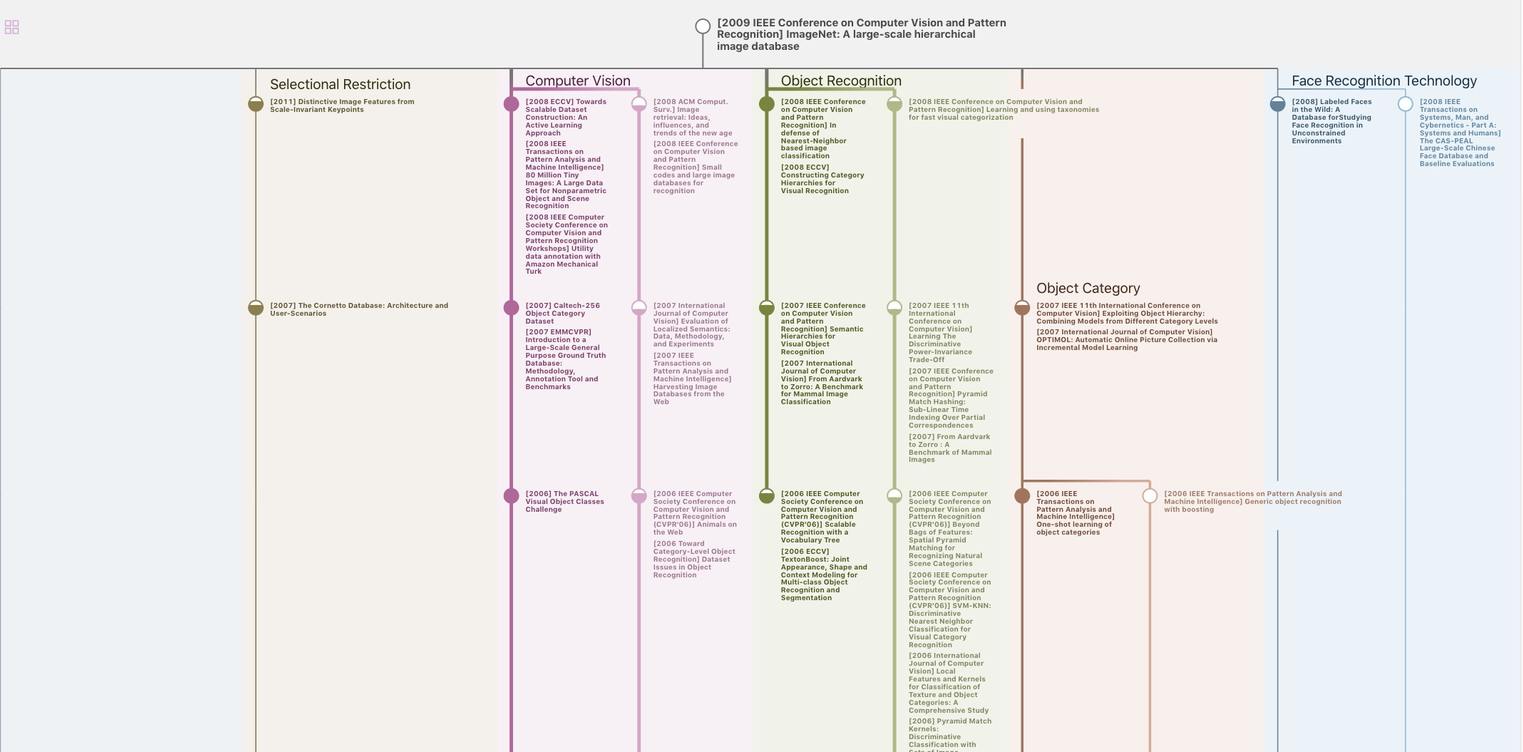
生成溯源树,研究论文发展脉络
Chat Paper
正在生成论文摘要