Learning a Stability Filter for Uncertain Differentially Flat Systems using Gaussian Processes.
CDC(2021)
摘要
Many physical system models exhibit a structural property known as differential flatness. Intuitively, differential flatness allows us to separate the system's nonlinear dynamics into a linear dynamics component and a nonlinear term. In this work, we exploit this structure and propose using a nonparametric Gaussian Process (GP) to learn the unknown nonlinear term. We use this GP in an optimization problem to optimize for an input that is most likely to feedback linearize the system (i.e., cancel this nonlinear term). This optimization is subject to input constraints and a stability filter, described by an uncertain Control Lyapunov Function (CLF), which probabilistically guarantees exponential trajectory tracking when possible. Furthermore, for systems that are control-affine, we choose to express this structure in the selection of the kernel for the GP. By exploiting this selection, we show that the optimization problem is not only convex but can be efficiently solved as a second-order cone program. We compare our approach to related works in simulation and show that we can achieve similar performance at much lower computational cost.
更多查看译文
关键词
differential flatness,linear dynamics component,nonparametric Gaussian Process,GP,unknown nonlinear term,optimization problem,input constraints,stability filter,uncertain Control Lyapunov Function,exponential trajectory tracking,uncertain differentially flat systems,Gaussian processes,physical system models,structural property
AI 理解论文
溯源树
样例
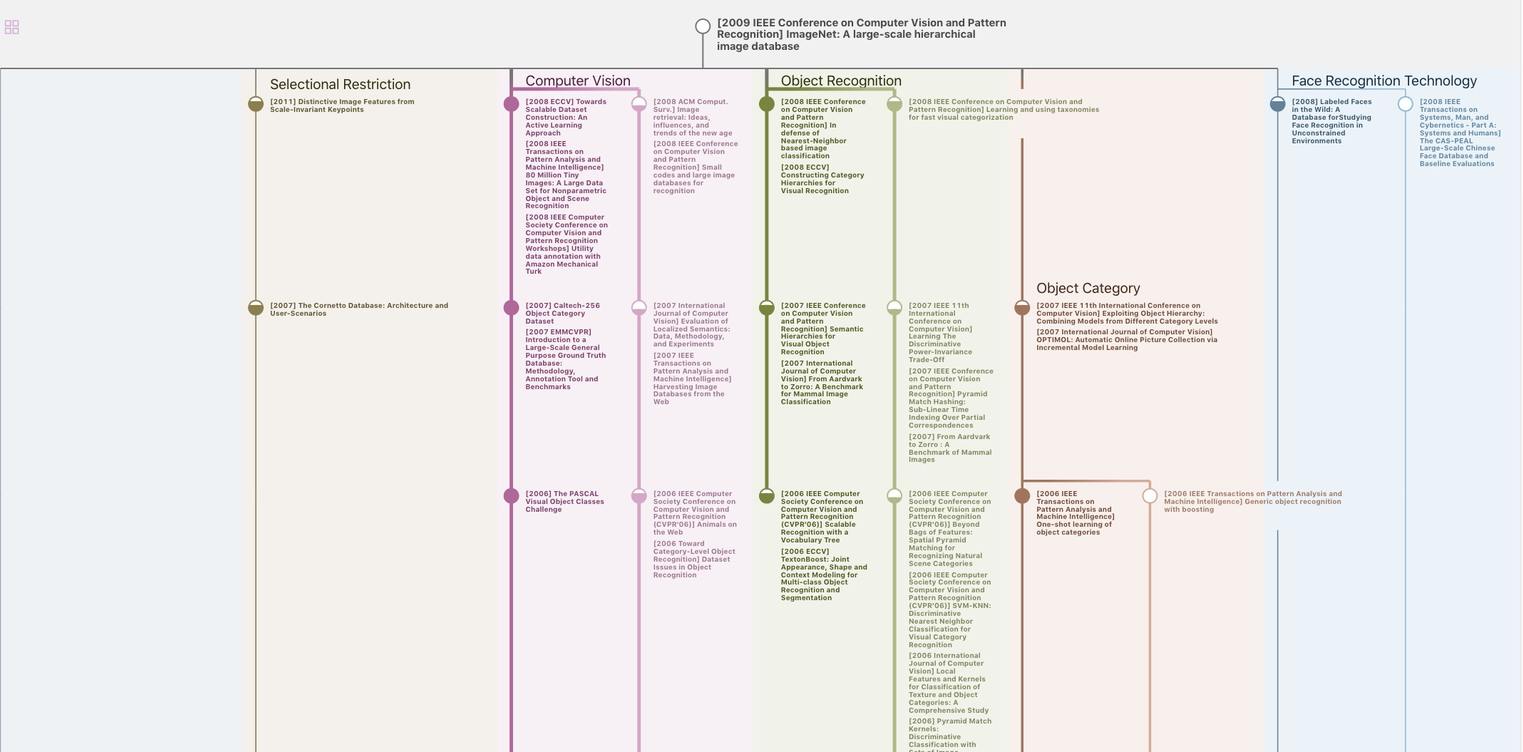
生成溯源树,研究论文发展脉络
Chat Paper
正在生成论文摘要