An Adaptive Exploratory Q-Learning Algorithm for Multiple Target Path Planning
2021 17th International Conference on Computational Intelligence and Security (CIS)(2021)
摘要
Inspired by the adaptive parameter adjustment of meta-heuristic algorithms, we propose here an adaptive exploratory mechanism with dynamic ε selection probability for the original Q-learning algorithm to enhance its convergence ability and alleviate falling into local optimum. In the original Q-learning algorithm, the ε value with greedy selection method is fixed in the whole exploratory process, and it seriously affects the search efficiency the agents. Firstly, this paper proposes a new exploratory which defines a particular value for each state-action pair and creates a dynamic state transition Table to control the exploratory probability of each state transition. Secondly, to better regulate its exploratory ability, this paper introduces an adaptive exploratory mechanism to dynamically control state transition value according to the overall distribution of agents. Finally, the proposed adaptive exploratory Q-learning (AE-Q-learning) algorithm is simulated in well-known grid map for multiple target path planning problem. The experimental results demonstrate that the AE-Q-learning algorithm is effective and feasible, and it also exhibits better convergence accuracy and exploratory ability compared the original Q-learning algorithm and other state-of-the-art improved Q-learning algorithms.
更多查看译文
关键词
Adaptive parameter,adaptive mutation strategy,Q-learning,reinforcement learning
AI 理解论文
溯源树
样例
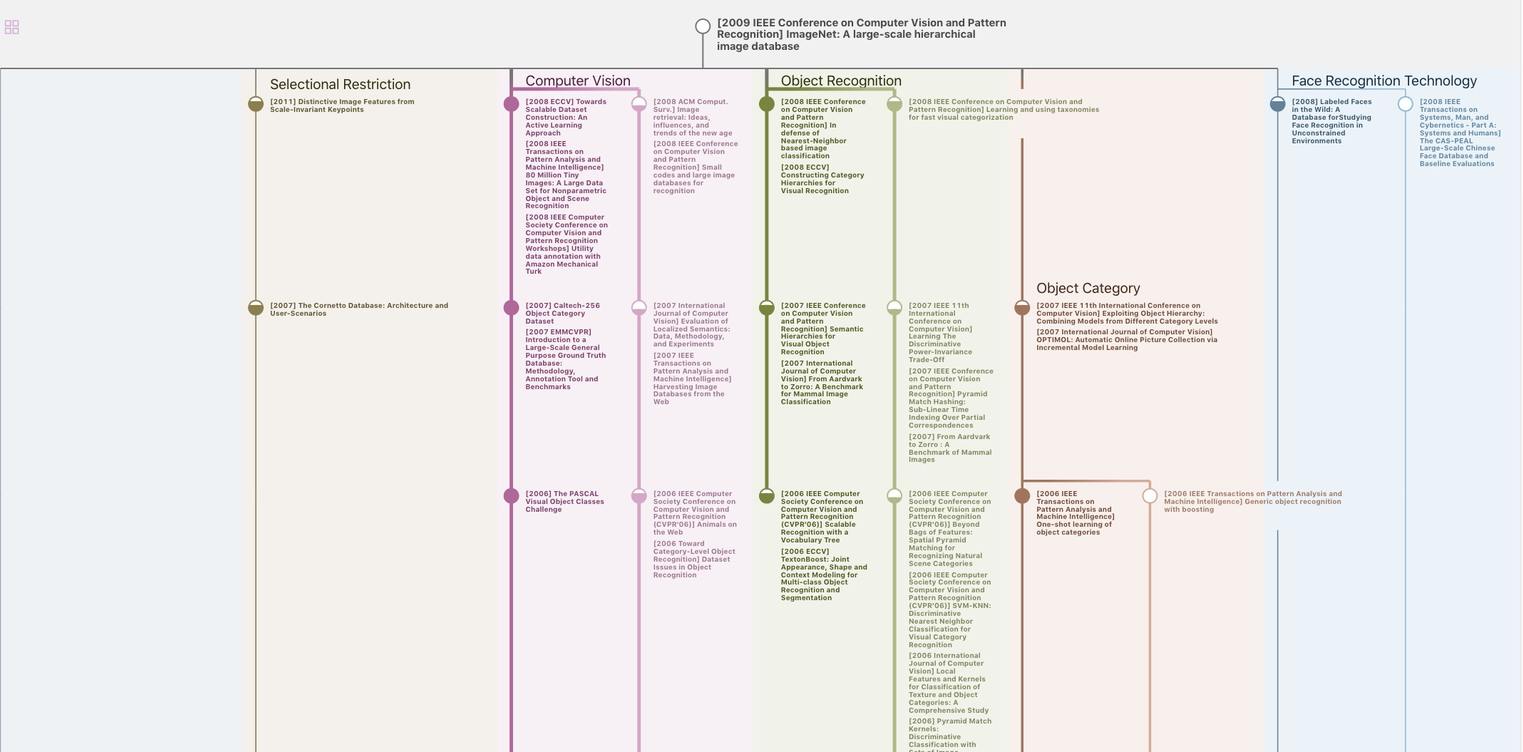
生成溯源树,研究论文发展脉络
Chat Paper
正在生成论文摘要