SIGNAV: Semantically-Informed GPS-Denied Navigation and Mapping in Visually-Degraded Environments
2022 IEEE WINTER CONFERENCE ON APPLICATIONS OF COMPUTER VISION (WACV 2022)(2022)
摘要
Understanding the perceived scene during navigation enables intelligent robot behaviors. Current vision-based semantic SLAM (Simultaneous Localization and Mapping) systems provide these capabilities. However, their performance decreases in visually-degraded environments, that are common places for critical robotic applications, such as search and rescue missions. In this paper, we present SIGNAV, a real-time semantic SLAM system to operate in perceptually-challenging situations. To improve the robustness for navigation in dark environments, SIGNAV leverages a multi-sensor navigation architecture to fuse vision with additional sensing modalities, including an inertial measurement unit (IMU), LiDAR, and wheel odometry. A new 2.5D semantic segmentation method is also developed to combine both images and LiDAR depth maps to generate semantic labels of 3D mapped points in real time. We demonstrate that the navigation accuracy from SIGNAV in a variety of indoor environments under both normal lighting and dark conditions. SIGNAV also provides semantic scene understanding capabilities in visually-degraded environments. We also show the benefits of semantic information to SIGNAV's performance.
更多查看译文
关键词
Vision for Robotics Vision for Aerial/Drone/Underwater/Ground Vehicles,Vision Systems and Applications
AI 理解论文
溯源树
样例
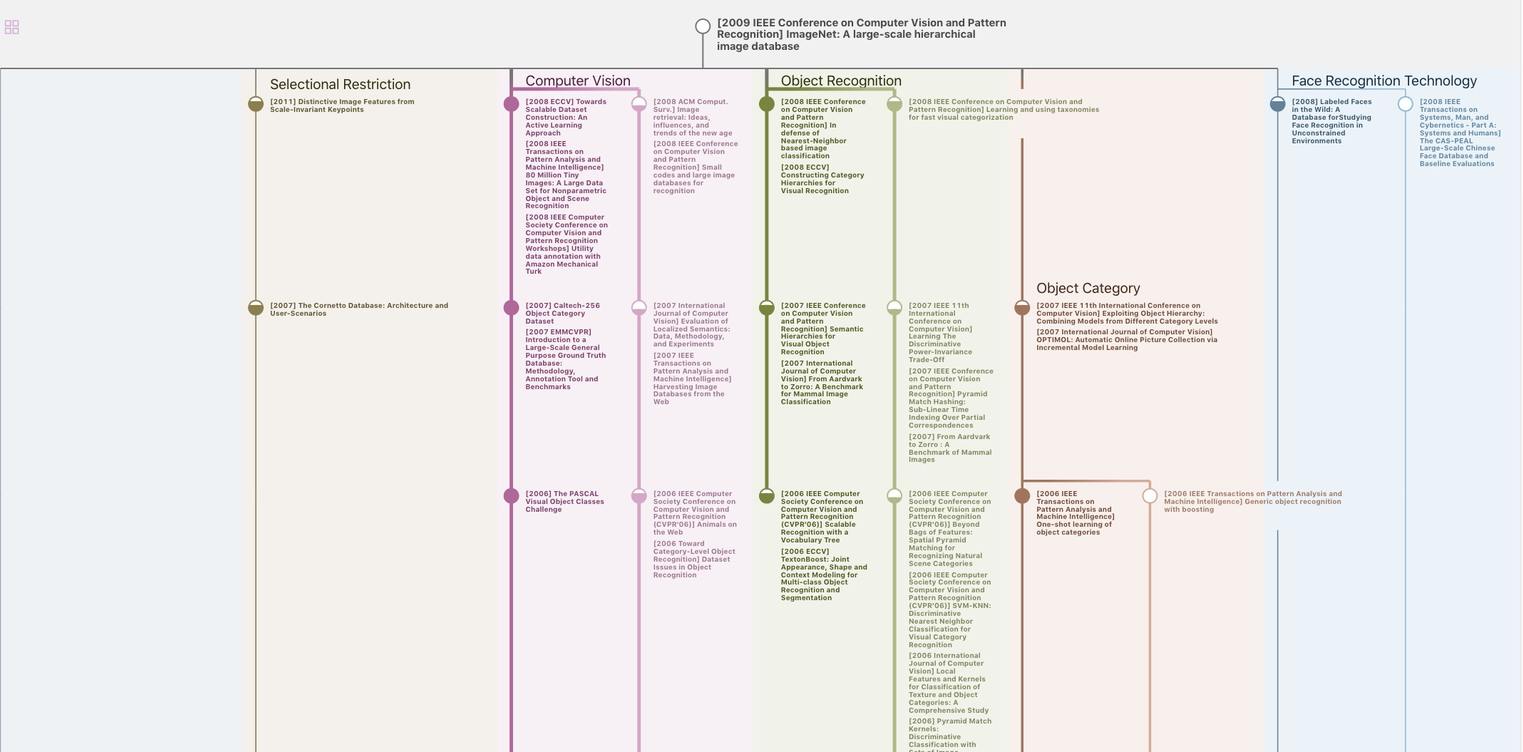
生成溯源树,研究论文发展脉络
Chat Paper
正在生成论文摘要