MoESR: Blind Super-Resolution using Kernel-Aware Mixture of Experts
2022 IEEE WINTER CONFERENCE ON APPLICATIONS OF COMPUTER VISION (WACV 2022)(2022)
摘要
Modern deep learning super-resolution approaches have achieved remarkable performance where the low-resolution (LR) input is a degraded high-resolution (HR) image by a fixed known kernel i.e. kernel-specific super-resolution (SR). However, real images often vary in their degradation kernels, thus a single kernel-specific SR approach does not often produce accurate HR results. Recently, degradationaware networks are introduced to generate blind SR results for unknown kernel conditions. They can restore images for multiple blur kernels. However, they have to compromise in quality compared to their kernel-specific counterparts. To address this issue, we propose a novel blind SR method called Mixture of Experts Super-Resolution (MoESR), which uses different experts for different degradation kernels. A broad space of degradation kernels is covered by kernel-specific SR networks (experts). We present an accurate kernel prediction method (gating mechanism) by evaluating the sharpness of images generated by experts. Based on the predicted kernel, our most suited expert network is selected for the input image. Finally, we fine-tune the selected network on the test image itself to leverage the advantage of internal learning. Our experimental results on standard synthetic datasets and real images demonstrate that MoESR outperforms state-of-the-art methods both quantitatively and qualitatively. Especially for the challenging x4 SR task, our PSNR improvement of 0.93 dB on the DIV2KRK dataset is substantial(1).
更多查看译文
关键词
Image Processing -> Image Restoration Super-resolution
AI 理解论文
溯源树
样例
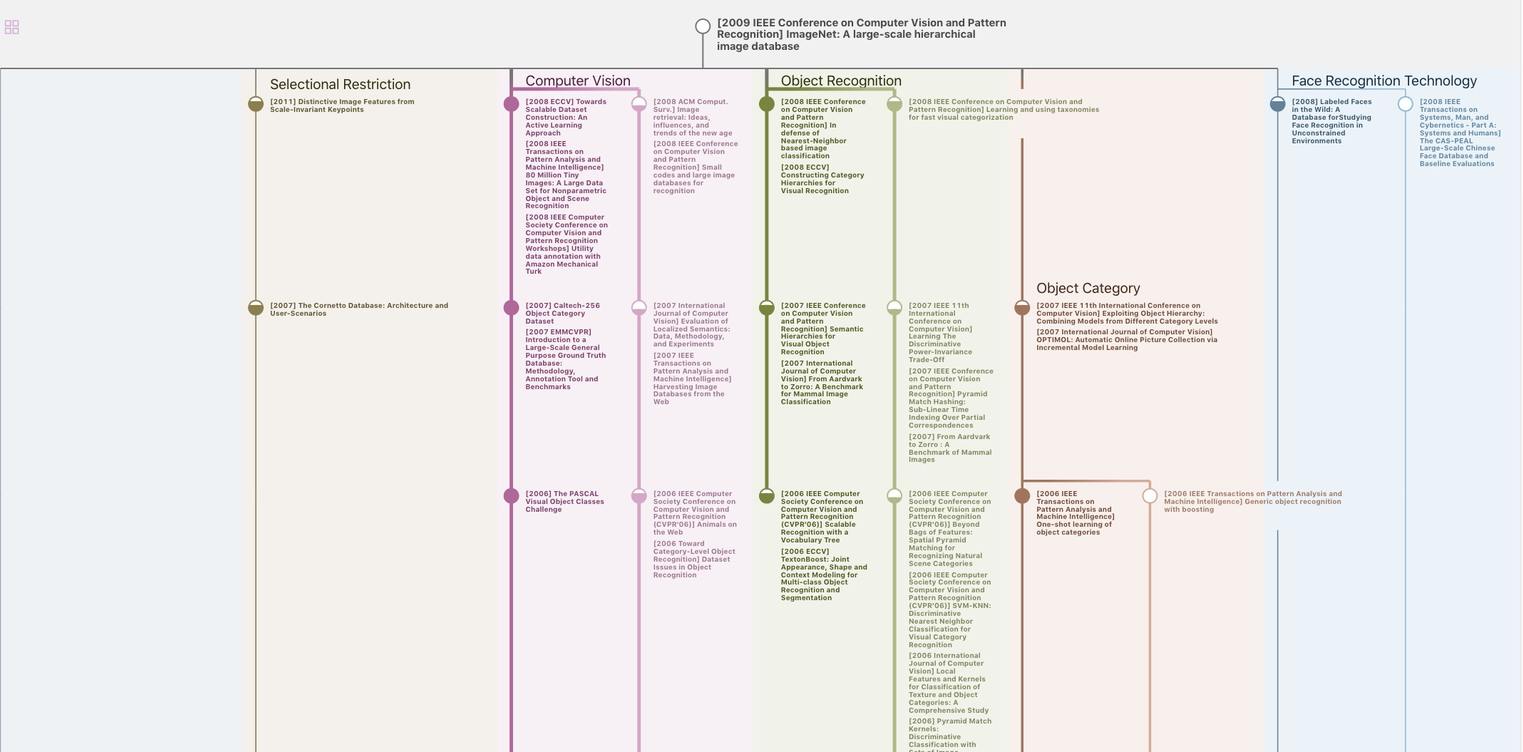
生成溯源树,研究论文发展脉络
Chat Paper
正在生成论文摘要