Identifying Root-Cause Metrics for Incident Diagnosis in Online Service Systems
2021 IEEE 32nd International Symposium on Software Reliability Engineering (ISSRE)(2021)
摘要
Incidents in online service systems could incur poor user experience and tremendous economic loss. To reduce the influence of incidents and guarantee service reliability, it is critical to identify root-cause metrics for engineers with clues to assist incident diagnosis. However, it is a challenging task due to the complicated dependencies and huge volume of various metrics in large-scale systems. Existing approaches are based on either anomaly detection or correlation analysis, performing not well in terms of accuracy or efficiency. To better understand the problem of root-cause metric identification, we conduct a preliminary study based on real-world data analysis and interactions with engineers. The key observation is that root-cause metrics should satisfy two requirements. One is that the metric is expected to behave abnormally during the incident; the other is that the anomaly pattern should meet physical meaning and engineers' demand. Motivated by the findings obtained from the study, we propose an effective approach named PatternMatcher to identifying root-cause metrics accurately. Specifically, PatternMatcher contains three steps, where coarse-grained anomaly detection aiming to filter out normal metrics, anomaly pattern classification aiming to filter out unimportant anomaly patterns, and root-cause metric ranking. An extensive study on four real-world datasets including 113 incident cases from a large commercial bank demonstrates that PatternMatcher outperforms all baseline approaches, achieving top-3 average accuracy of 0.91. Moreover, we have deployed PatternMatcher in practice and shared some successful cases from real deployment.
更多查看译文
关键词
Root-cause metric,incident diagnosis,anomaly pattern classification
AI 理解论文
溯源树
样例
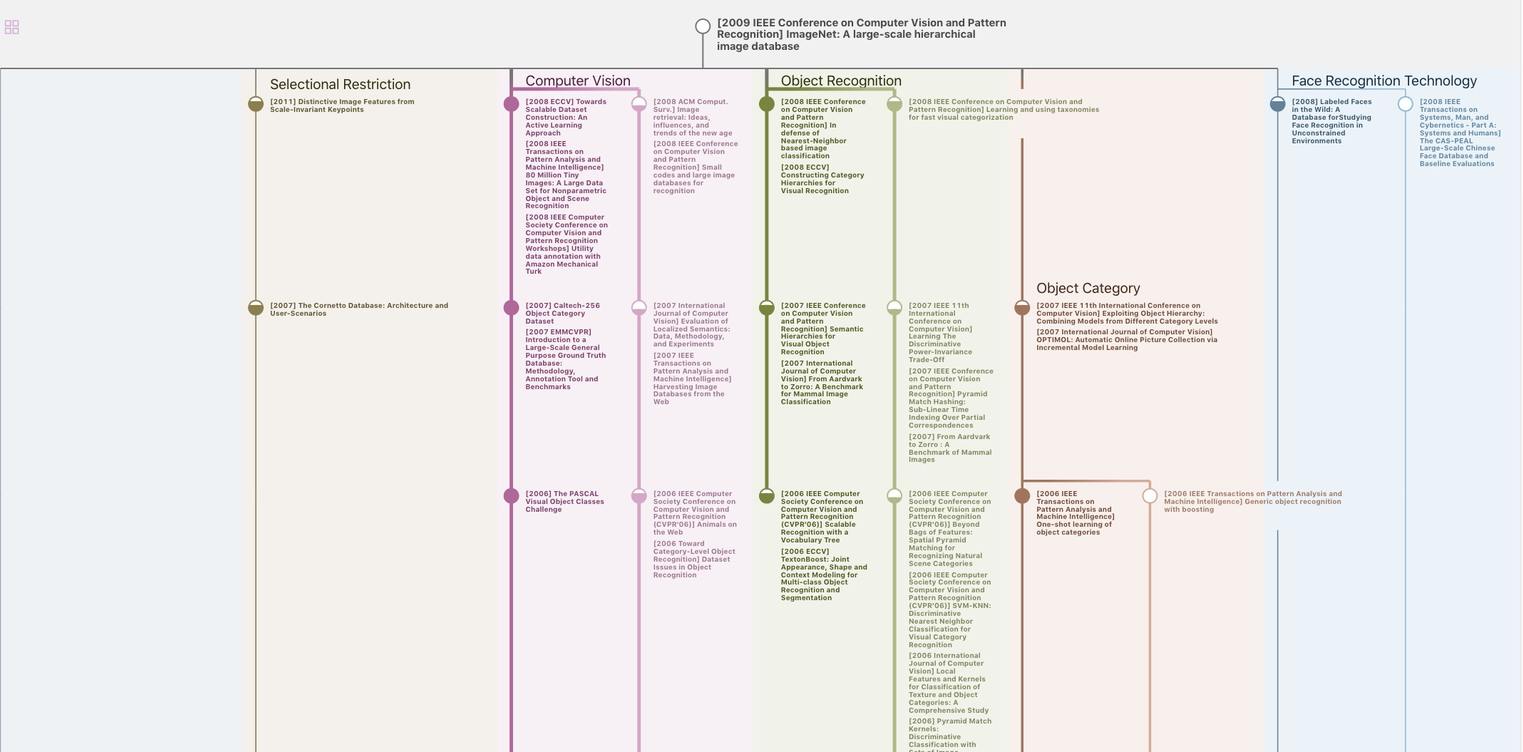
生成溯源树,研究论文发展脉络
Chat Paper
正在生成论文摘要