RHC: Cluster based Feature Reduction for Network Intrusion Detections
2022 IEEE 19th Annual Consumer Communications & Networking Conference (CCNC)(2022)
摘要
Intrusion Detection Systems (IDSs) play an important part in securing computer networks from different malicious threats and attacks. Modern IDSs leverage machine learning algorithms for effective intrusion detection. However, network traffic flows contain a large number of redundant features in a high-dimensional feature space which decreases the performance of such data-driven approaches drastically. Existing feature reduction methods lack to effectively remove redundant features as well as to retain features with additional information (if there is any). In this paper, we propose a Redundancy based Hierarchical Clustering (RHC) method that groups redundant features in the same cluster based on mutual information. We use both feature selection and extraction for finding the final feature set. We conduct rigorous experiments on three benchmark security datasets and our results demonstrate that the proposed methods outperform the state-of-the-art methods in terms of accuracy, f-score and false positive rate. We show the superiority of our proposed methods in both binary class (normal vs attack) and multi-class classification.
更多查看译文
关键词
Network Intrusion Detection System,Hierarchical Clustering,Robust Mahalanobis Distance,Mutual Information,Feature Reduction
AI 理解论文
溯源树
样例
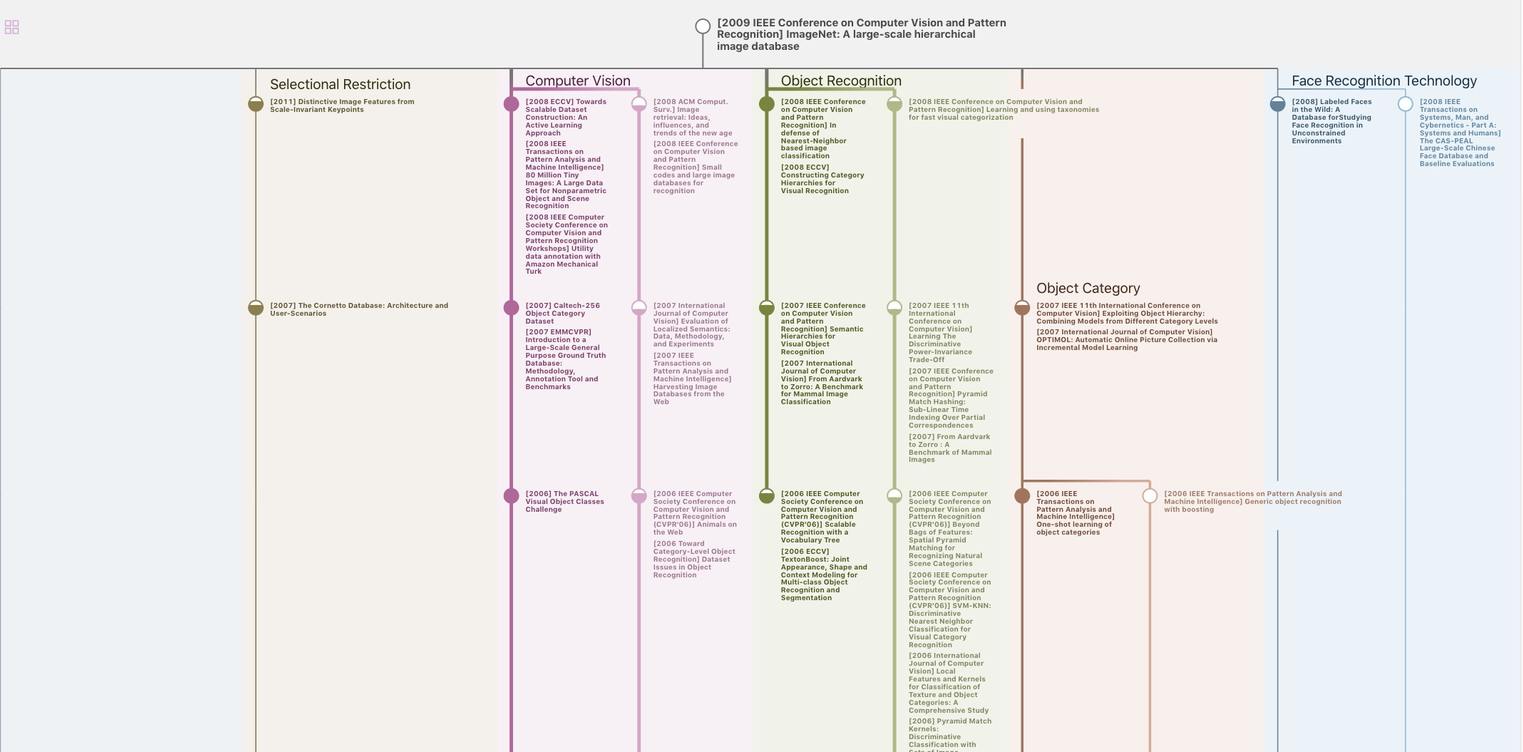
生成溯源树,研究论文发展脉络
Chat Paper
正在生成论文摘要