Shining New Light on Useful Features for Network Intrusion Detection Algorithms
2022 IEEE 19th Annual Consumer Communications & Networking Conference (CCNC)(2022)
摘要
Network intrusion detection systems (NIDS) today must quickly provide visibility into anomalous behavior on a growing amount of data. Meanwhile different data models have evolved over time, each providing a different set of features to classify attacks. Defenders have limited time to retrain classifiers, while the scale of data and feature mismatch between data models can affect the ability to periodically retrain. Much work has focused on classification accuracy yet feature selection is a key part of machine learning that, when optimized, reduces the training time and can increase accuracy by removing poorly performing features that introduce noise. With a larger feature space, the pursuit of more features is not as valuable as selecting better features. In this paper, we use an ensemble approach of filter methods to rank features followed by a voting technique to select a subset of features. We evaluate our approach using three datasets to show that, across datasets and network topologies, similar features have a trivial effect on classifier accuracy after removal. Our approach identifies poorly performing features to remove in a classifier-agnostic manner that can significantly save time for periodic retraining of production NIDS.
更多查看译文
关键词
Network Intrusion Detection Systems,NIDS,Ensemble Feature Selection
AI 理解论文
溯源树
样例
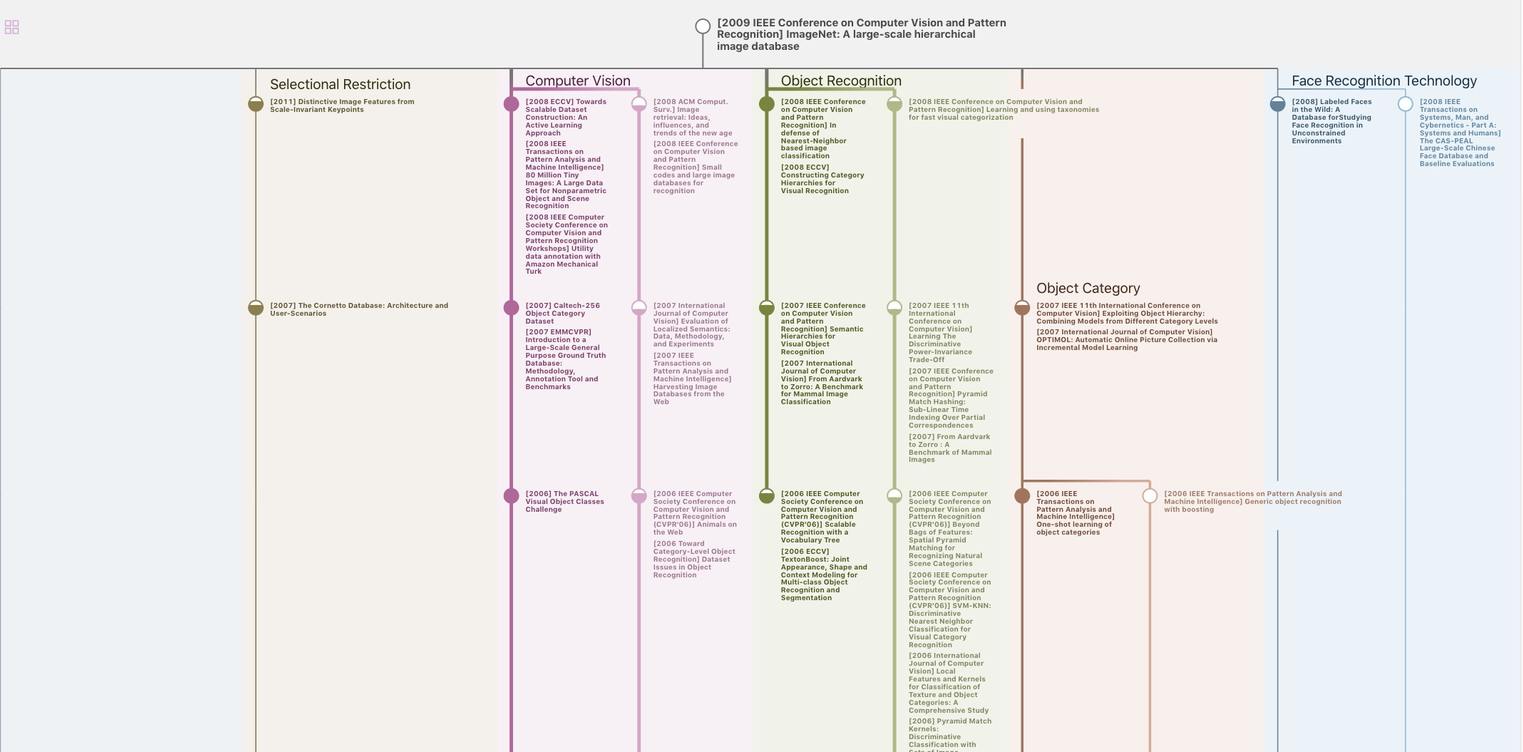
生成溯源树,研究论文发展脉络
Chat Paper
正在生成论文摘要