DualDE: Dually Distilling Knowledge Graph Embedding for Faster and Cheaper Reasoning
WSDM(2022)
摘要
ABSTRACTKnowledge Graph Embedding (KGE) is a popular method for KG reasoning and training KGEs with higher dimension are usually preferred since they have better reasoning capability. However, high-dimensional KGEs pose huge challenges to storage and computing resources and are not suitable for resource-limited or time-constrained applications, for which faster and cheaper reasoning is necessary. To address this problem, we propose DualDE, a knowledge distillation method to build low-dimensional student KGE from pre-trained high-dimensional teacher KGE. DualDE considers the dual-influence between the teacher and the student. In DualDE, we propose a soft label evaluation mechanism to adaptively assign different soft label and hard label weights to different triples, and a two-stage distillation approach to improve the student's acceptance of the teacher. Our DualDE is general enough to be applied to various KGEs. Experimental results show that our method can successfully reduce the embedding parameters of a high-dimensional KGE by 7× - 15× and increase the inference speed by 2× - 6× while retaining a high performance. We also experimentally prove the effectiveness of our soft label evaluation mechanism and two-stage distillation approach via ablation study.
更多查看译文
关键词
knowledge graph embedding, fast embedding, knowledge distillation
AI 理解论文
溯源树
样例
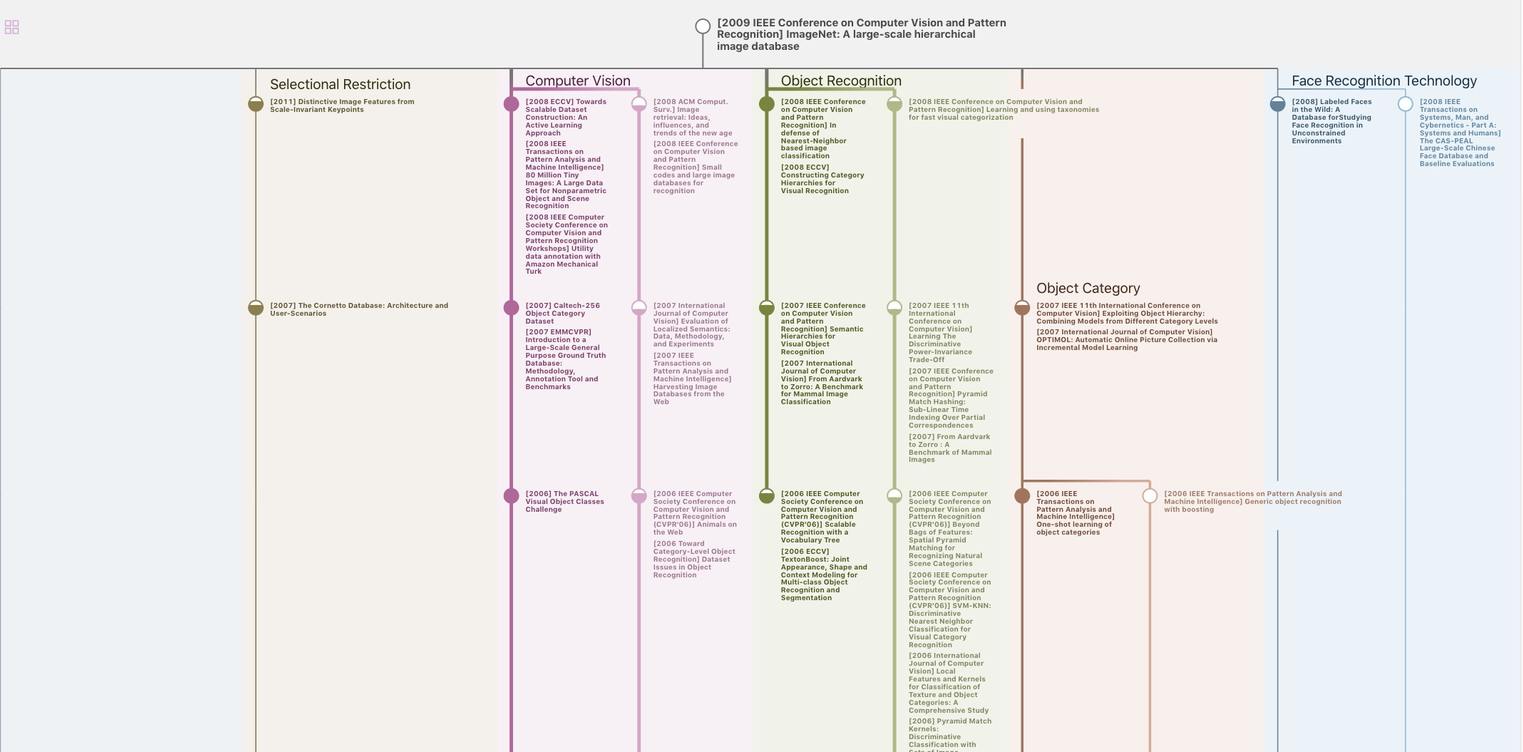
生成溯源树,研究论文发展脉络
Chat Paper
正在生成论文摘要