Efficient Two-stage Label Noise Reduction for Retrieval-based Tasks
WSDM(2022)
摘要
ABSTRACTThe existence of noisy labels in datasets has always been an essential dilemma in deep learning studies. Previous works detected noisy labels by analyzing the predicted probability distribution generated by the model trained on the same data and calculating the probabilities of each label to be regarded as noise. However, the predicted probability distribution from the whole dataset may introduce overfitting, and the overfitting on noisy labels may induce the probability distribution of clean and noisy items to be not conditional independent, making identification more challenging. Additionally, label noise reduction on image datasets has received much attention, while label noise reduction on text datasets has not. This paper proposes a noisy label reduction method for text datasets, which could be applied at retrieval-based tasks by getting a conditional independent probability distribution to identify noisy labels accurately. The method first generates a candidate set containing noisy labels, predicts the category probabilities by the model trained on the rest cleaner data, and then identifies noisy items by analyzing a confidence matrix. Moreover, we introduce a warm-up module and a sharpened cross-entropy loss function for efficiently training in the first stage. Empirical results on different rates of uniform and random label noise in five text datasets demonstrate that our method can improve the label noise reduction accuracy and end-to-end classification accuracy. Further, we find that the iteration of the label noise reduction method is efficient to high-rate label noise datasets, and our method will not hurt clean datasets too much.
更多查看译文
关键词
Data cleaning, noisy labels, text dataset, retrieval-based tasks
AI 理解论文
溯源树
样例
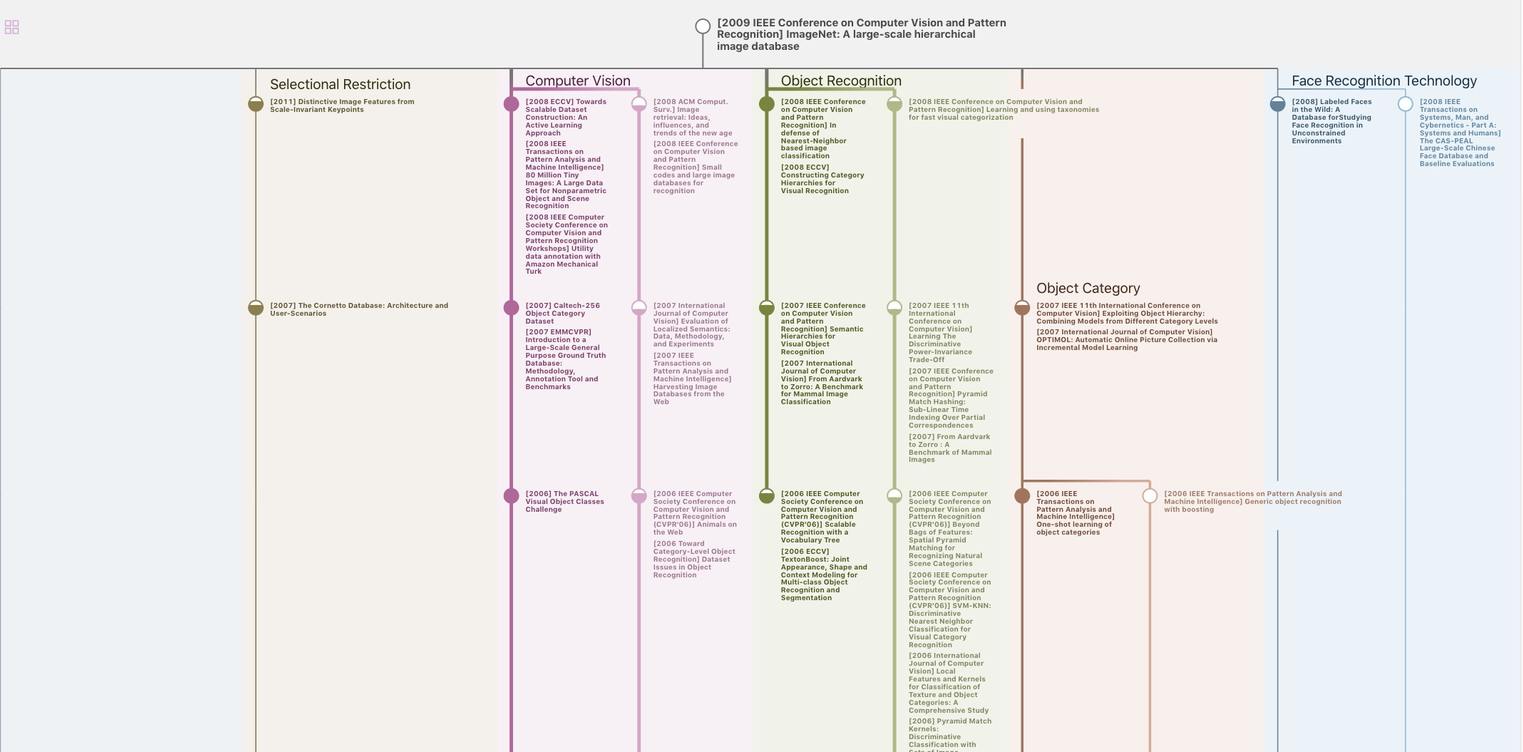
生成溯源树,研究论文发展脉络
Chat Paper
正在生成论文摘要