Malware Detection and Classification along with Trade-off Analysis for Number of Features, Feature Types, and Speed
2021 International Conference on Frontiers of Information Technology (FIT)(2021)
摘要
Existing research in malware detection and classification has focused on the higher accuracy of the results. Generally, high accuracy is achieved through a large set of entropy features in most of the existing frameworks. However, detection and classification time (which also includes the feature extraction time) have largely been neglected in past studies. In this paper, we propose a neural network-based model for Windows-based malware detection and classification. We also study the impact of reducing the number of features, feature types, and feature extraction time on the accuracy of malware detection and classification. We compared our model with Gaussian Naive Bayes (gNB), Logistic Regression (LR), K-Nearest Neighbor (KNN), Classification and Regression Trees (CART), Random Forest (RF), and Extreme Gradient Boosting (XGB). Our proposed model achieves the highest accuracy of 92.17% for detection and 98.41% for classification by using only 100 features of entropy. Results demonstrate that by replacing entropy features with the GINI-index features and by adjusting feature sets, we can get 21.93% faster detection and 15.6% faster classification at the cost of less than 3% and 2% reduction in accuracy respectively.
更多查看译文
关键词
Cyber-security,Entropy analysis,GINI-Index analysis,malware detection,malware classification,supervised learning
AI 理解论文
溯源树
样例
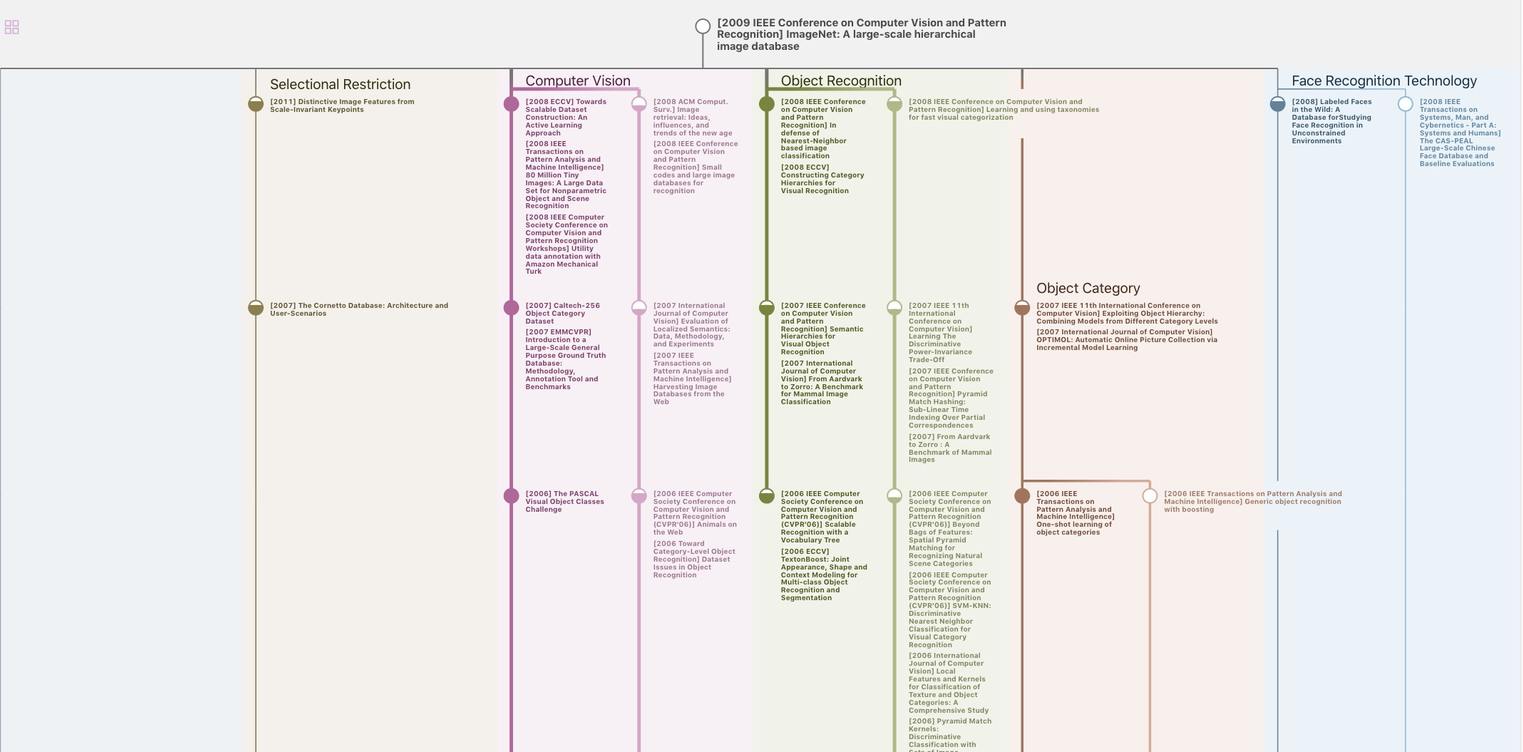
生成溯源树,研究论文发展脉络
Chat Paper
正在生成论文摘要