Track Segment Association With Dual Contrast Neural Network
IEEE Transactions on Aerospace and Electronic Systems(2022)
摘要
Track interruption will cause serious interference to target tracking, situation awareness, and information fusion. And it is often triggered by a variety of reasons, such as target maneuver, the blind spot of Doppler radar, and excessive long sampling interval, among other causes. All the existing algorithms are based on the hypothetical target motion models and adopt the theory of statistical estimation to measure the similarity between predicted tracks to associate, which have some disadvantages such as unreasonable hypotheses, inapplicable models, and uncertain thresholds. In order to address these problems, in this article, we propose a track segment association dual contrast neural network (TSADCNN) to complete track segment association tasks. First, based on deep metric learning, we propose a temporal and spatial information extraction module to extract track information, especially symmetric correlation information and raise dimension. Second, by constructing an appropriate optimization function, TSADCNN makes track segments belonging to the same target closer, track segments belonging to different targets farther in high-dimensional space. Finally, TSADCNN will pick the nearest neighbor vectors in high-dimensional space as associated tracks. Extensive experiments on simulation data are conducted to evaluate the effectiveness of TSADCNN, and the results demonstrate that this method has good adaptability and anti-noise ability. Furthermore, the contrast experiment shows that it can outperform other TSA methods in both quality and efficiency. Compared with the best performance, the average true association rate of TSADCNN can be increased by 1.3% in long interrupt intervals.
更多查看译文
关键词
Deep learning,metric learning,track interruption,track segment association (TSA)
AI 理解论文
溯源树
样例
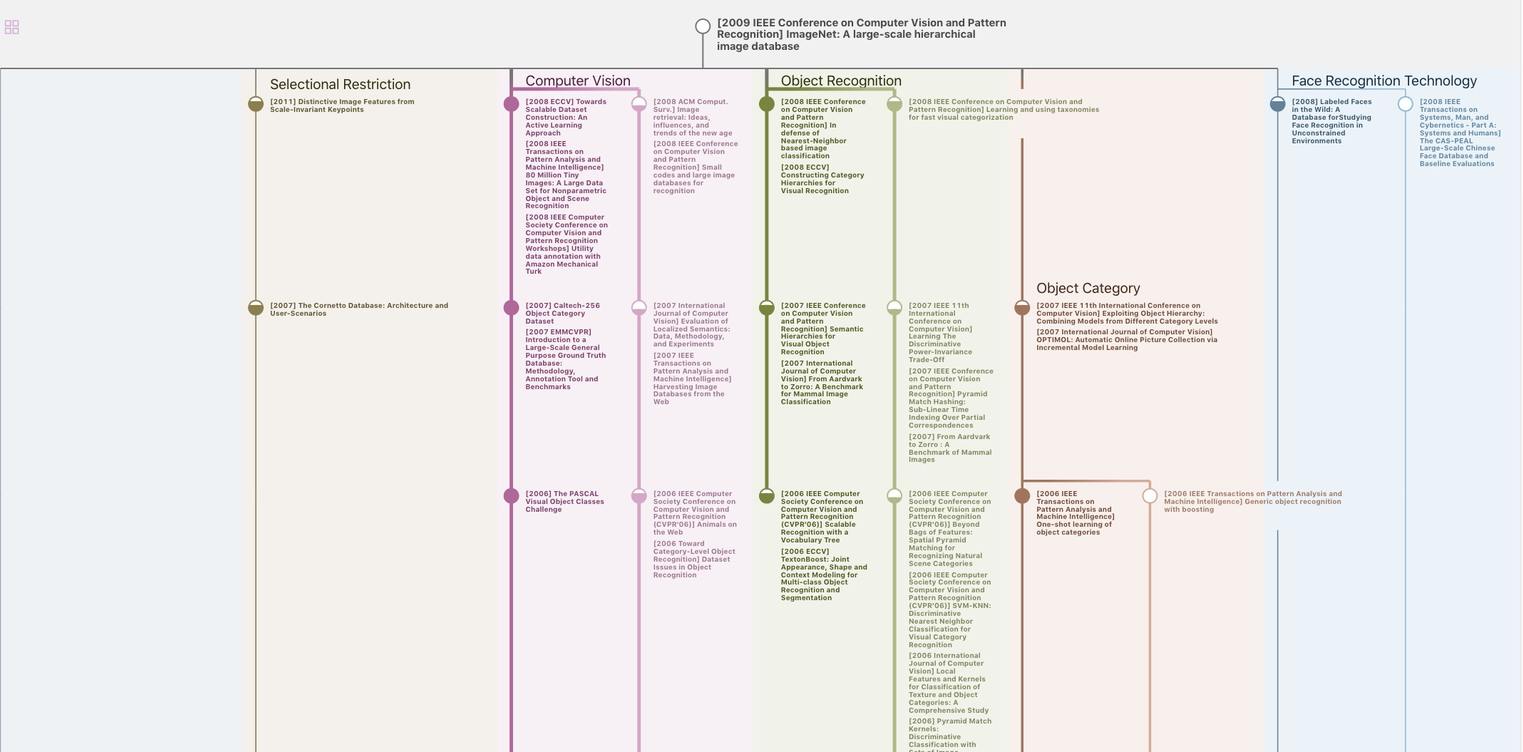
生成溯源树,研究论文发展脉络
Chat Paper
正在生成论文摘要