QueryNet: Querying neural networks for lightweight specialized models
Information Sciences(2022)
摘要
Despite the active research on deep learning these days, no existing works attempt to query neural networks for a specific task so that we can quickly obtain a model specialized for the queried task. This paper presents the first study on the problem of querying neural networks, aiming to efficiently find a lightweight model for any on-demand sub-task supported by a large and generic neural network. This problem is well motivated by the fact that such a lightweight model is particularly suitable for deployment in commodity mobile devices with limited computing resources. In this paper, we propose QueryNet, a framework of queriable neural networks, which is based on our class-aware channel pruning technique and the proposed method of merging multiple tiny networks for producing a specialized model for the task. Through the extensive experiments, we show that QueryNet can generate a lightweight neural network for a given task up to 3.25 times faster than learning a specialized model from scratch, and the resulting specialized neural network carries up to 37 times less parameters than a pretrained network, and even shows a slightly better accuracy than that of the pretrained model.
更多查看译文
关键词
Querying Neural Networks,Model Specialization,Lightweight Models
AI 理解论文
溯源树
样例
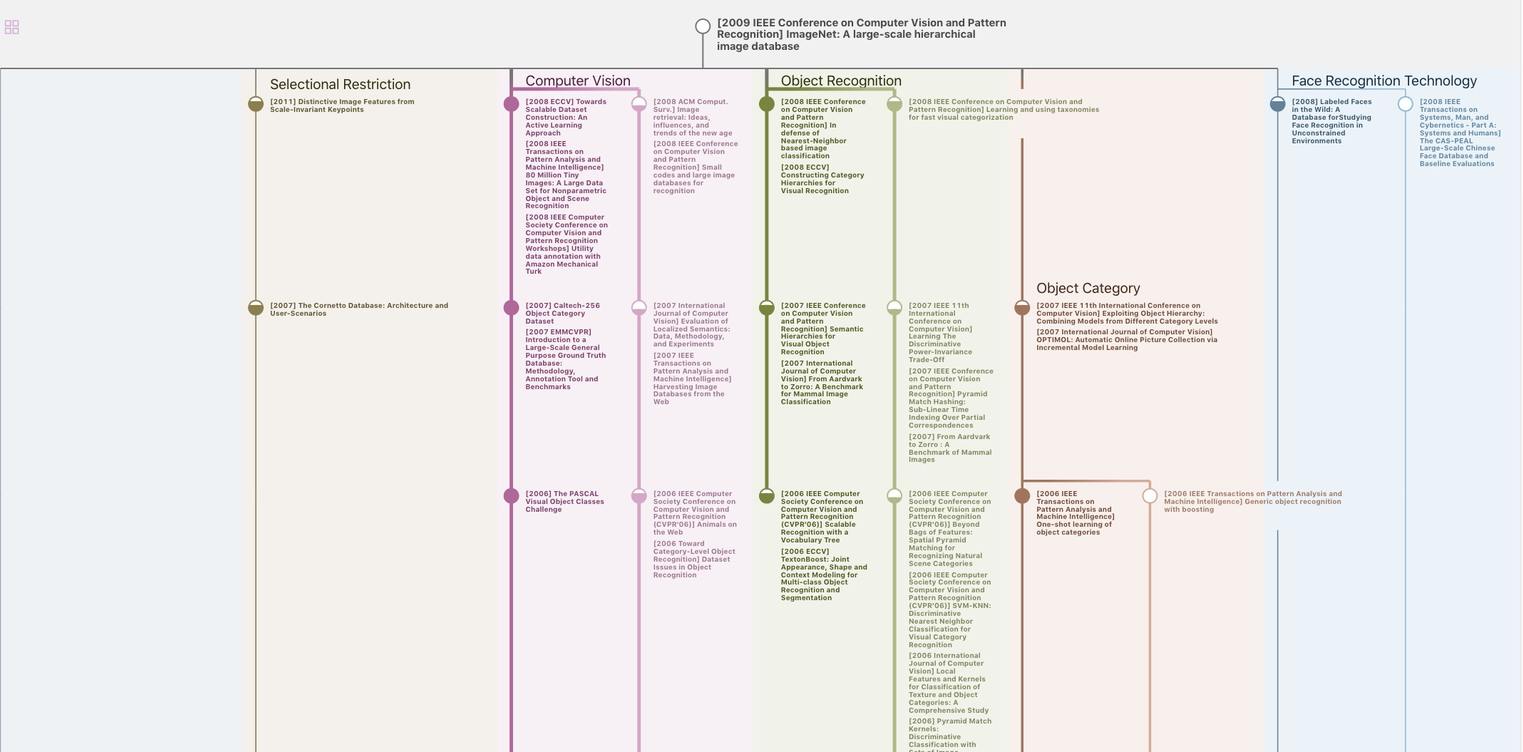
生成溯源树,研究论文发展脉络
Chat Paper
正在生成论文摘要