How Can Our Tweets Go Viral? Point-Process Modelling of Brand Content
Information & Management(2022)
摘要
People create and share content via online social networks, which provide an unparalleled opportunity for brands to gain visibility, promote products or services and drive revenue growth. Much research has focused on why, how, or what social content is popular, trending and “hype”. One central challenge is to forecast the spread (cascades) of information that leads to the popularity of content throughout a social network. Online content tends to have bursts and spikes, experiencing a different cascading pattern depending on the viral propagation. In this paper, we propose and test a flexible framework capable of modelling such patterns and trends. We take temporal and network perspectives and develop a model based on the multivariate Hawkes processes that account for social behaviour and network elements such as follower counts, and activity variation observed in collective re-sharing behaviour. We focus on Twitter as the most widely used micro-blogging online social network and measure the popularity of a brand's tweet by analysing the time-series path of three types of subsequent activities (retweets (RTs), replies (REs) and likes (LKs)). The specific model that we propose in this paper is the multidimensional epidemic-type aftershock sequence (METAS) model, a particular case of the multivariate Hawkes process. It consists of a power-law relaxation governing the timing of activities. It also includes an exponential boost as a reinforcement mechanism for the response amplitude to model the impact of influential users on their followers. Earlier attempts to model online cascades have treated all online responses as one type of activity. Rather than aggregating all the activities into one stream, and therefore, ignoring exciting effects among different types of activities, we incorporate the activity variation into the predictive models of content popularity, explicitly accounting for such excitation effects. We develop epidemic-type mutually exciting Hawkes point processes models to quantify such effects and to predict more accurately the number of follow-up activities (i.e., RTs, REs and LKs) on a brand tweet after it is posted. Our results suggest that the proposed model outperforms the state-of-the-art models in terms of prediction accuracy, as it is able to account for mutual excitations and cross-interactions between sequences of users’ activities from one type to another. These results are relevant for developing and executing a plan for online activities by the brand owners.
更多查看译文
关键词
Social media,Analytics,Online social networks,Brand-generated content,Brand post popularity,Hawkes process
AI 理解论文
溯源树
样例
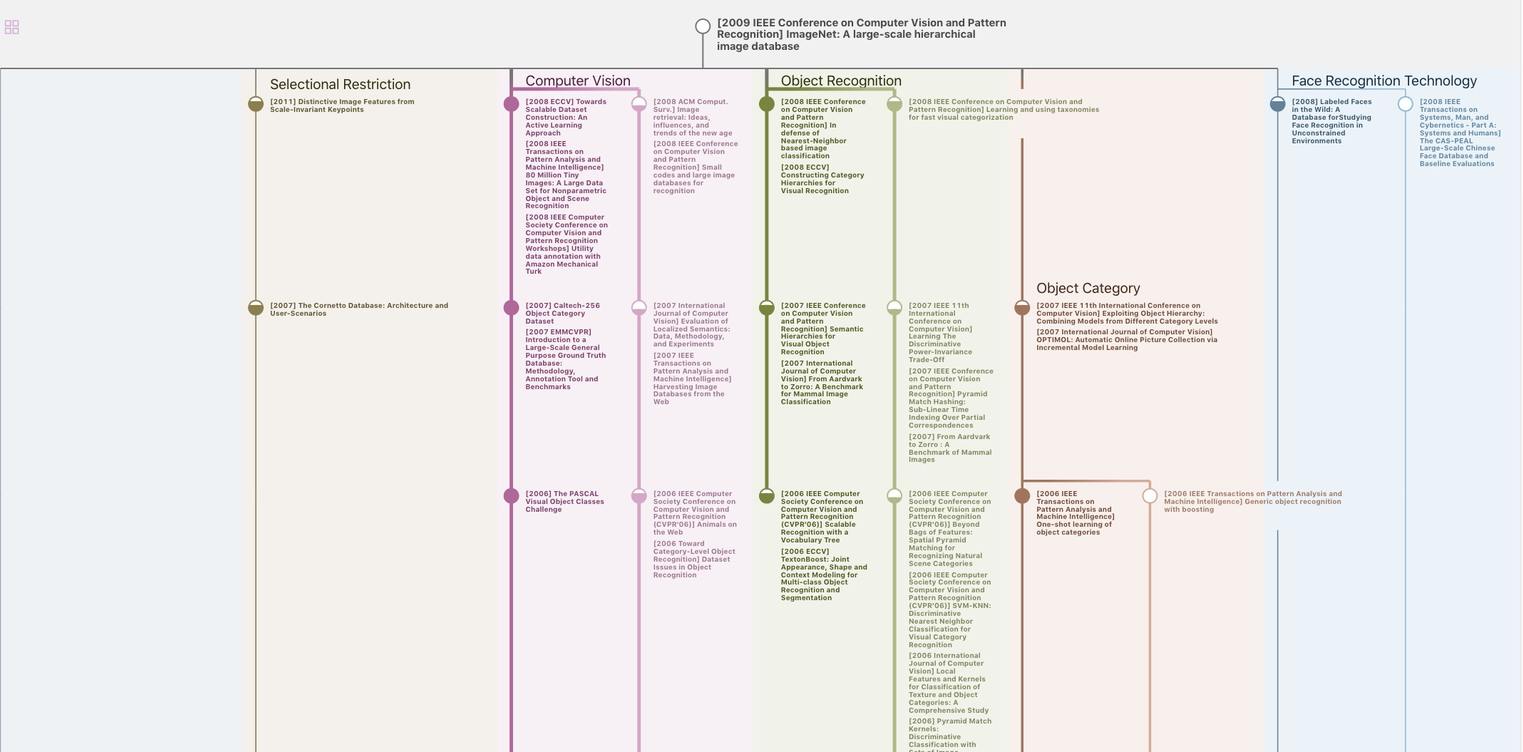
生成溯源树,研究论文发展脉络
Chat Paper
正在生成论文摘要