Excogitating marine predators algorithm based on random opposition-based learning for feature selection
CONCURRENCY AND COMPUTATION-PRACTICE & EXPERIENCE(2022)
Abstract
Obtaining precise information from a high-dimensional dataset is one of the most difficult tasks as datasets contain more features and fewer samples. The high-dimensionality of the dataset reduces predictive capability and increases the computational complexity of the analytical model. The widespread employment of meta-heuristic methods to handle the challenge of high-dimensional datasets has been exceptional in recent years. The marine predators algorithm (MPA) is a recently developed meta-heuristic algorithm based on the "survival-of-the-fittest" notion. This research critique overcomes the drawbacks of the existing MPA and proposes a feature selection model using random opposition-based learning (ROBL). The searching for the optimum solution in a single direction of the traditional MPA reduces its performance. The incorporation of ROBL in the MPA enhances its ability to reconnoiter bigger search space. The proposed algorithm generates a new population based on the initial and random opposite population. The performance of ROBL-MPA is inspected on six high-dimensional microarray datasets. The results of the proposed ROBL-MPA are compared to traditional MPA and opposition based MPA (OBL-MPA). The proposed ROBL-MPA outperforms traditional MPA based on several benchmark performance analysis tests.
MoreTranslated text
Key words
feature selection, marine predators algorithm, meta-heuristic optimization, random opposition-based learning
AI Read Science
Must-Reading Tree
Example
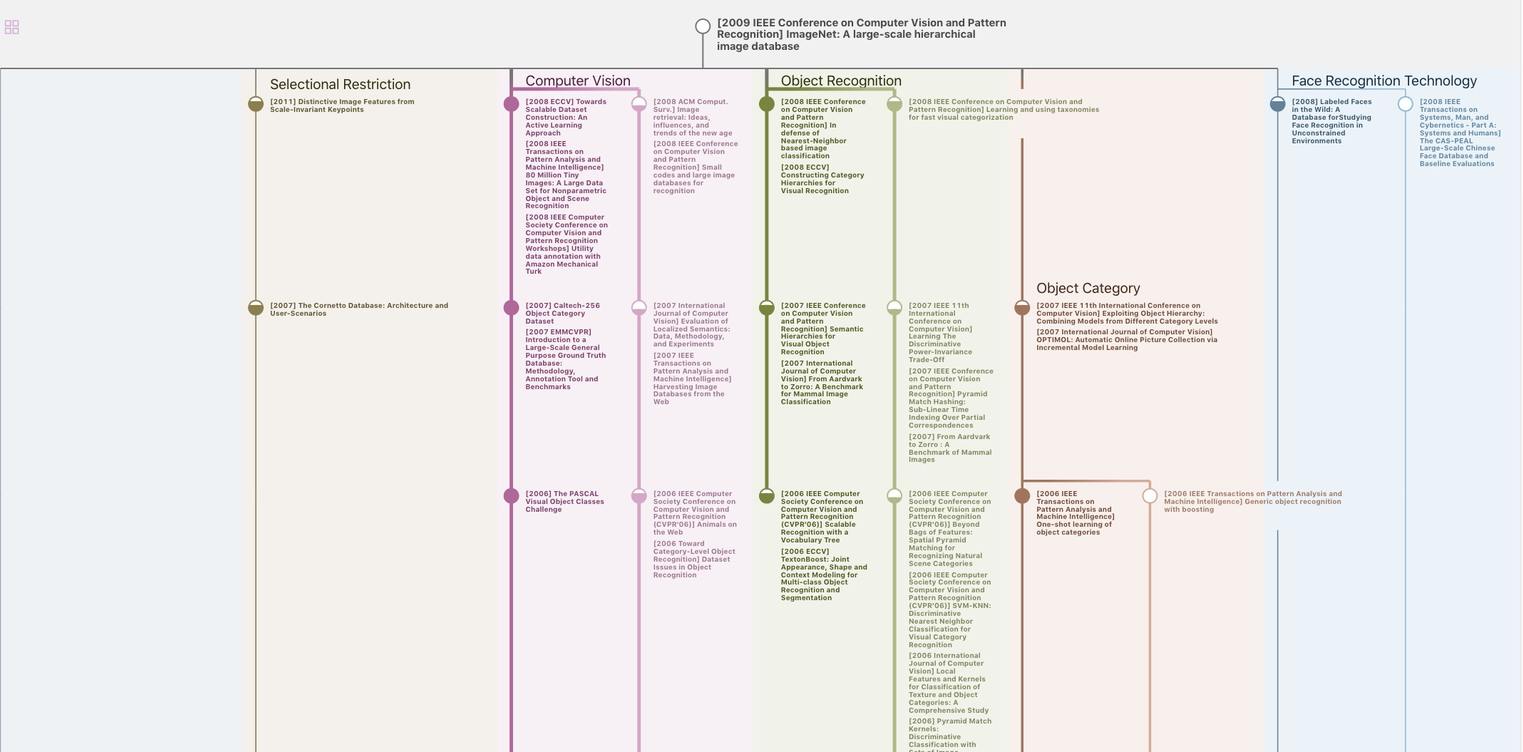
Generate MRT to find the research sequence of this paper
Chat Paper
Summary is being generated by the instructions you defined