High-correlation 3D routability estimation for congestion-guided global routing
The Journal of Supercomputing(2023)
摘要
Routability estimation identifies potentially congested areas in advance to achieve high-quality routing solutions. To improve the routing quality, this paper presents a deep learning-based congestion estimation algorithm, which serves to guide the initial pattern routing of a global router to reduce unexpected overflows. Unlike existing methods based on traditional compressed 2D features for model training and prediction, our algorithm extracts appropriate 3D features from the placed netlists. Furthermore, an improved RUDY (Rectangular Uniform wire DensitY) method is developed to estimate 3D routing demands. For the model selection, we employ the U-net model with good image prediction ability as a routability estimator, and the prediction results are used for guiding the initial routing process. Since our training set is formed by cropping training designs, the structural information between two adjacent cropped designs may be missed. Hence, we divide experiments into two parts, experiments based on normal designs and experiments based on big designs. Compared with the state-of-the-art method, experimental results show that our routability estimator can significantly improve the Pearson Correlation Coefficient (PCC) index and reduce the Mean Absolute Normalized Error (MANE) and the Standard Deviation in the Normalized Error (SDNE). Furthermore, our congestion-guided global routing can reduce the routing overflows, wirelength, and via count both on normal designs and big designs, compared to CUGR.
更多查看译文
关键词
Deep learning,Machine learning,Physical design,Routing congestion,Very large-scale integration
AI 理解论文
溯源树
样例
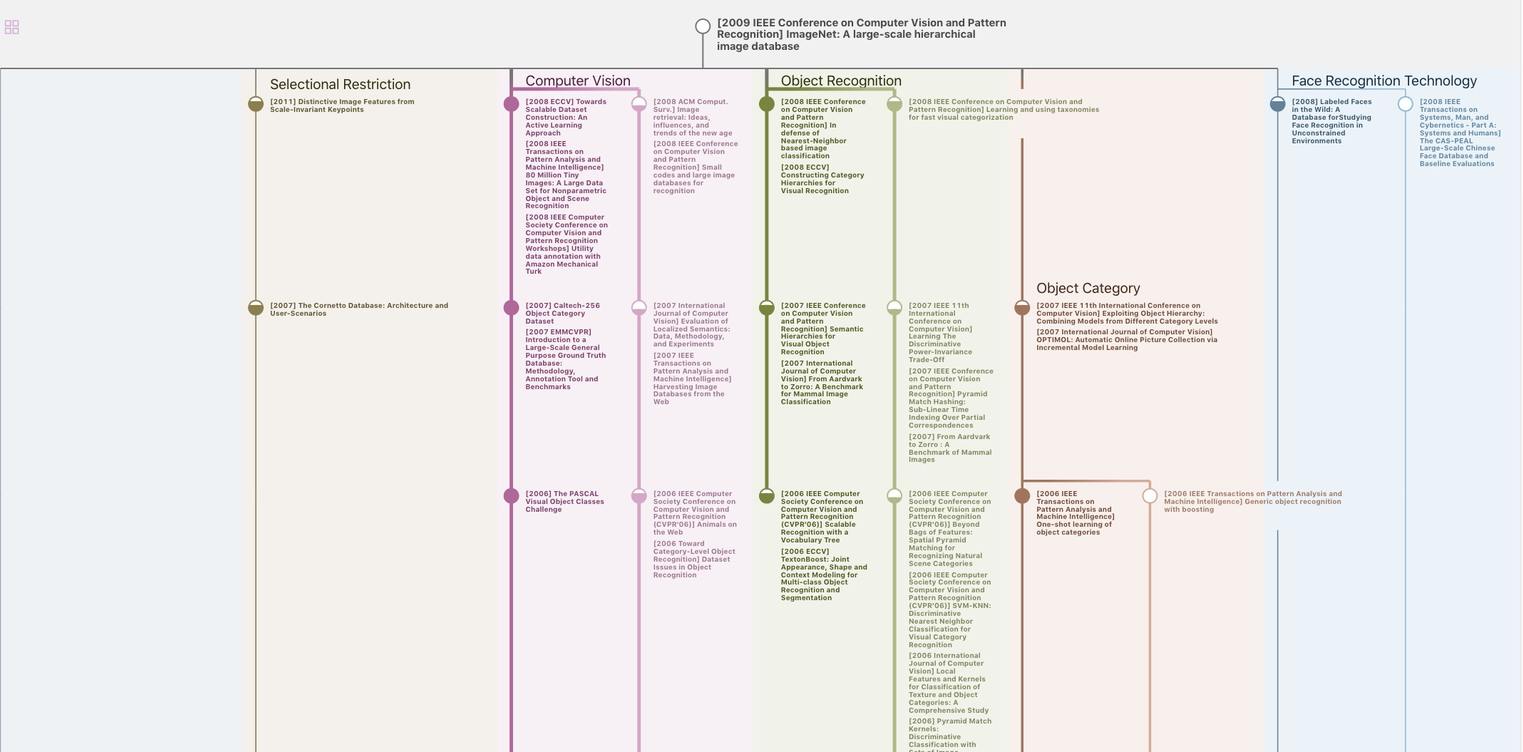
生成溯源树,研究论文发展脉络
Chat Paper
正在生成论文摘要