Global Solutions to Nonconvex Problems by Evolution of Hamilton-Jacobi PDEs
arxiv(2023)
摘要
Computing tasks may often be posed as optimization problems. The objective functions for real-world scenarios are often nonconvex and/or nondifferentiable. State-of-the-art methods for solving these problems typically only guarantee convergence to local minima. This work presents Hamilton-Jacobi-based Moreau adaptive descent (HJ-MAD), a zero-order algorithm with guaranteed convergence to global minima, assuming continuity of the objective function. The core idea is to compute gradients of the Moreau envelope of the objective (which is “piece-wise convex”) with adaptive smoothing parameters. Gradients of the Moreau envelope (i.e., proximal operators) are approximated via the Hopf-Lax formula for the viscous Hamilton-Jacobi equation. Our numerical examples illustrate global convergence.
更多查看译文
关键词
Global optimization,Moreau envelope,Hamilton-Jacobi,Hopf-Lax,Cole-Hopf,Proximals,Zero-order optimization
AI 理解论文
溯源树
样例
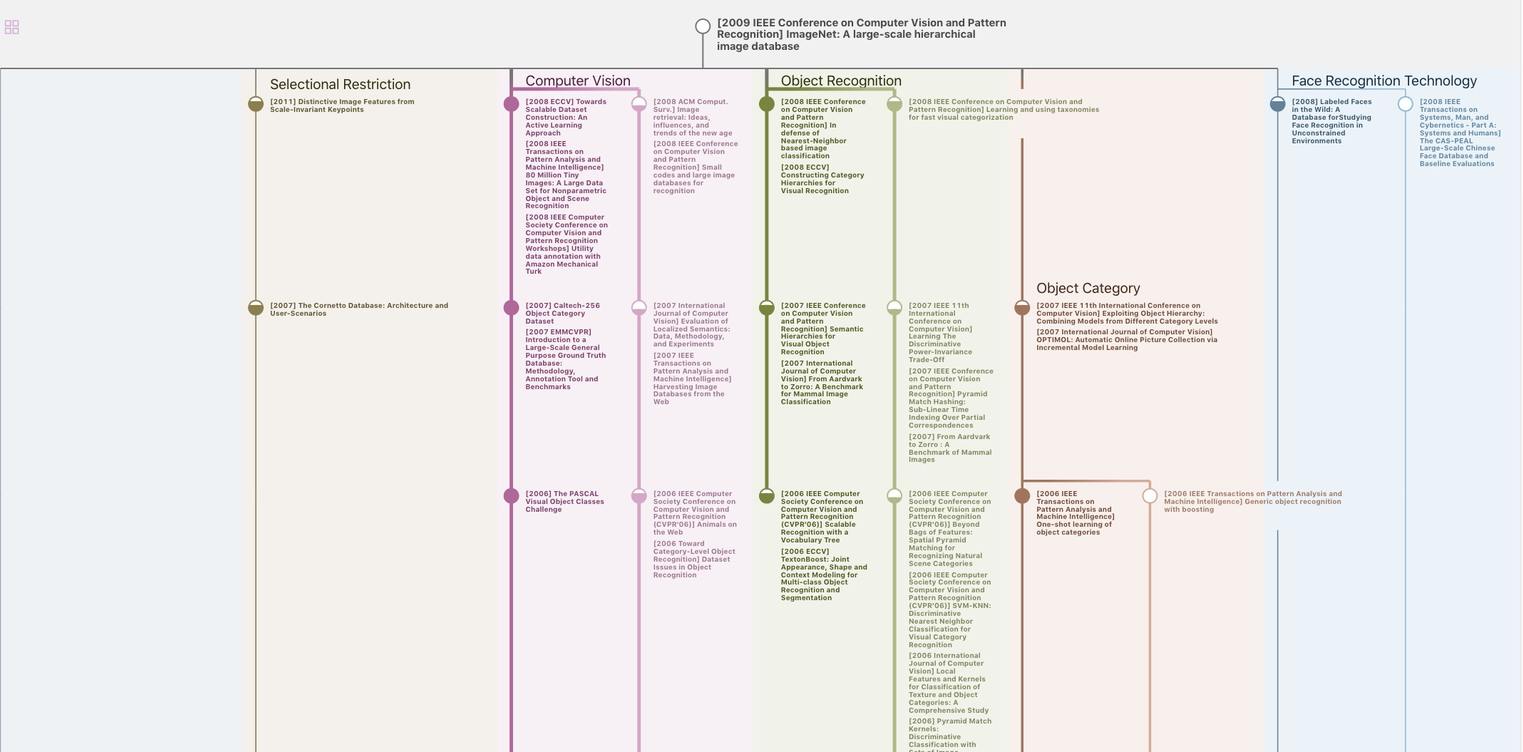
生成溯源树,研究论文发展脉络
Chat Paper
正在生成论文摘要