PyTorch Geometric Signed Directed: A Software Package on Graph Neural Networks for Signed and Directed Graphs
CoRR(2022)
摘要
Networks are ubiquitous in many real-world applications (e.g., social networks encoding trust/distrust relationships, correlation networks arising from time series data). While many networks are signed or directed, or both, there is a lack of unified software packages on graph neural networks (GNNs) specially designed for signed and directed networks. In this paper, we present PyTorch Geometric Signed Directed (PyGSD), a software package which fills this gap. Along the way, we also provide a brief review surveying typical tasks, loss functions and evaluation metrics in the analysis of signed and directed networks, discuss data used in related experiments, provide an overview of methods proposed, and evaluate the implemented methods with experiments. The deep learning framework consists of easy-to-use GNN models, synthetic and real-world data, as well as task-specific evaluation metrics and loss functions for signed and directed networks. As an extension library for PyG, our proposed software is maintained with open-source releases, detailed documentation, continuous integration, unit tests and code coverage checks. Our code is publicly available at \url{https://github.com/SherylHYX/pytorch_geometric_signed_directed}.
更多查看译文
关键词
graph neural
AI 理解论文
溯源树
样例
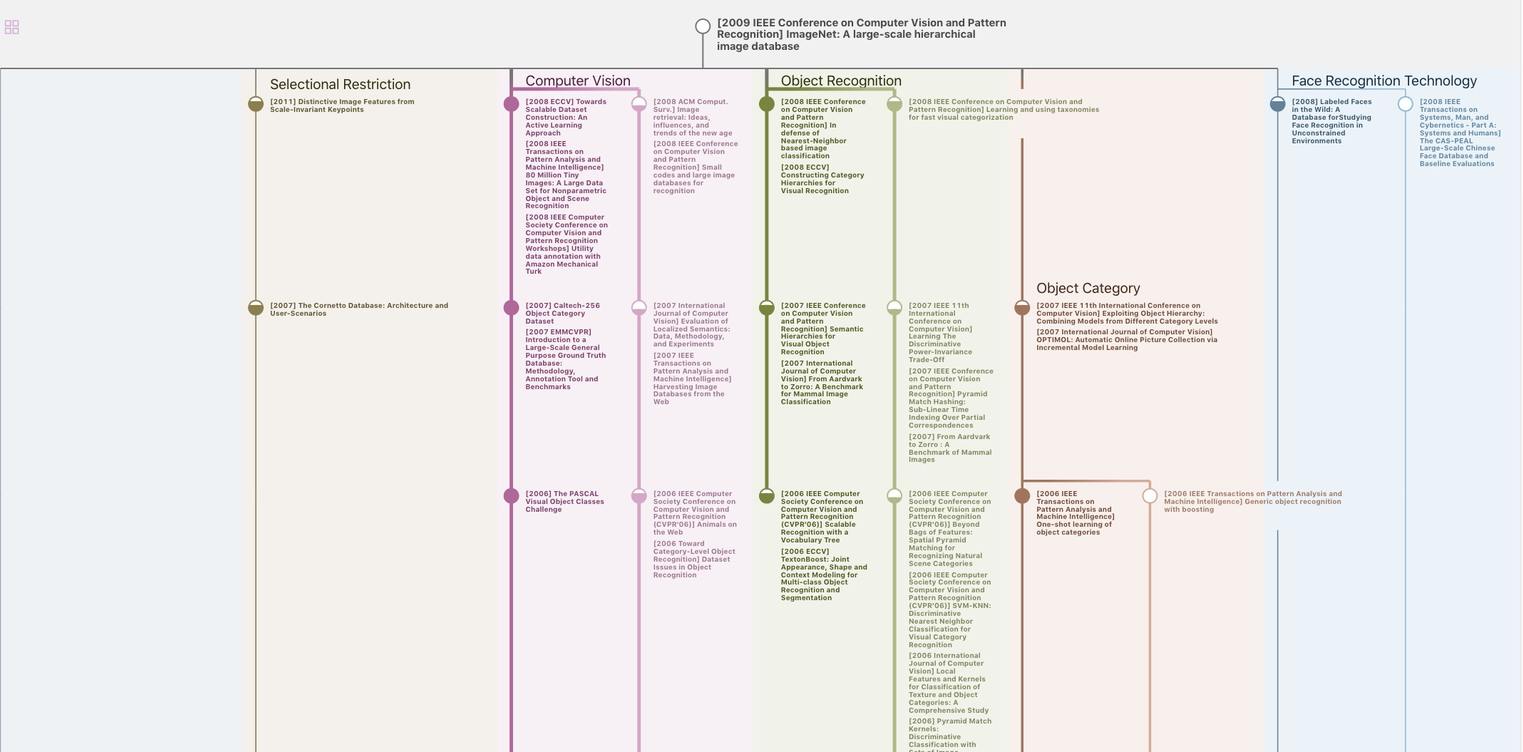
生成溯源树,研究论文发展脉络
Chat Paper
正在生成论文摘要