Combating Sampling Bias: A Self-Training Method in Credit Risk Models.
AAAI Conference on Artificial Intelligence(2022)
Abstract
A significant challenge in credit risk models for underwriting is the presence of bias in model training data. When most credit risk models are built using only applicants who had been funded for credit, such non-random sampling predominantly influenced by credit policymakers and previous loan performances may introduce sampling bias to the models, and thus alter their prediction of default on loan repayment when screening applications from prospective borrowers. In this paper, we propose a novel data augmentation method that aims to identify and pseudo-label parts of the historically declined loan applications to mitigate sampling bias in the training data. We also introduce a new measure to assess the performance from the business perspective, loan application approval rates at various loan default rate levels. Our proposed methods were compared to the original supervised learning model and the traditional sampling issue remedy techniques in the industry. The experiment and early production results from deployed model show that self-training method with calibrated probability as data augmentation selection criteria improved the ability of credit scoring to differentiate default loan applications and, more importantly, can increase loan approval rate up to 8.8\%, while keeping similar default rate comparing to baselines. The results demonstrate practical implications on how future underwriting model development processes should follow.
MoreTranslated text
Key words
Credit Risk,Self-training,Reject Inference
AI Read Science
Must-Reading Tree
Example
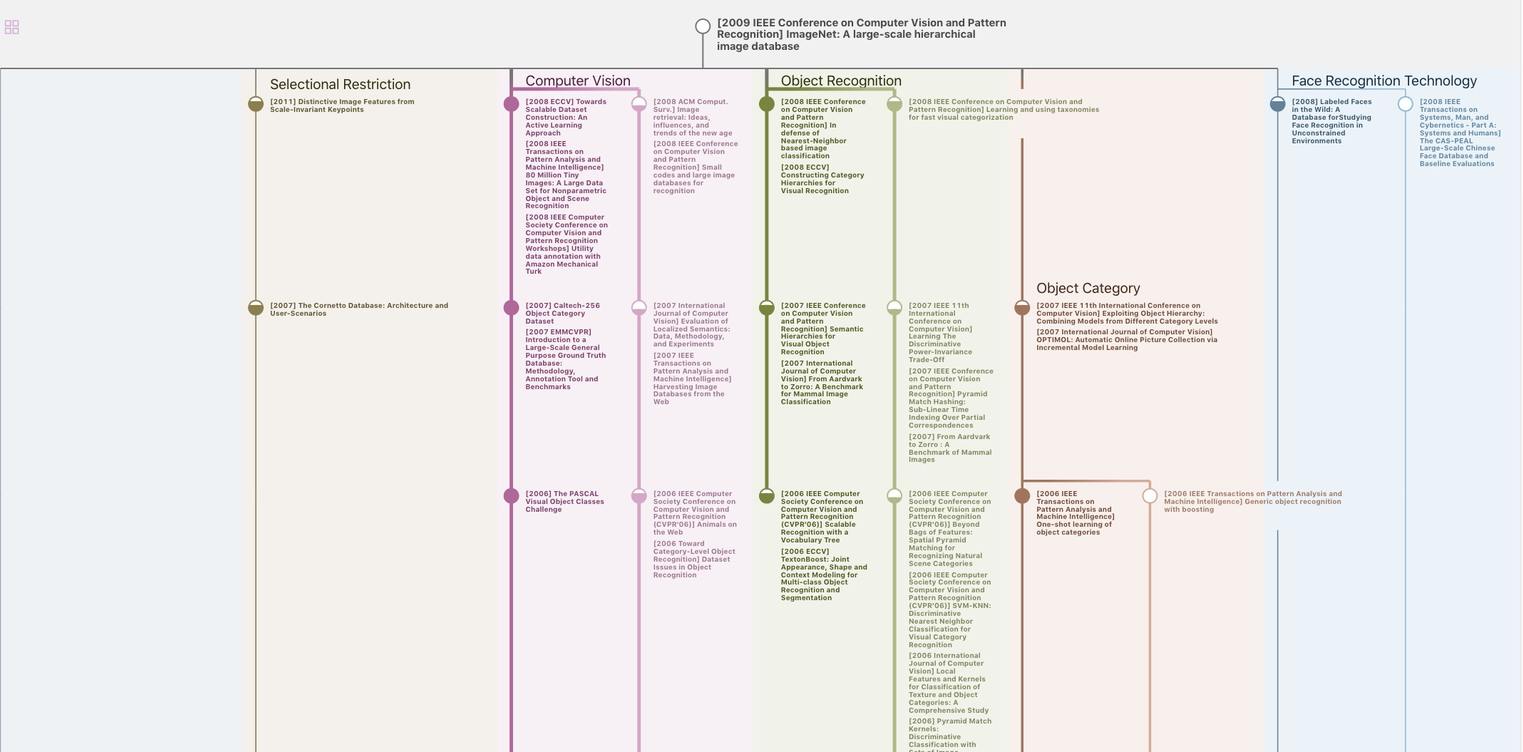
Generate MRT to find the research sequence of this paper
Chat Paper
Summary is being generated by the instructions you defined