Hypergraph Modeling via Spectral Embedding Connection: Hypergraph Cut, Weighted Kernel k-means, and Heat Kernel
Proceedings of the ... AAAI Conference on Artificial Intelligence(2022)
Abstract
We propose a theoretical framework of multi-way similarity to model real-valued data into hypergraphs for clustering via spectral embedding. For graph cut based spectral clustering, it is common to model real-valued data into graph by modeling pairwise similarities using kernel function. This is because the kernel function has a theoretical connection to the graph cut. For problems where using multi-way similarities are more suitable than pairwise ones, it is natural to model as a hypergraph, which is generalization of a graph. However, although the hypergraph cut is well-studied, there is not yet established a hypergraph cut based framework to model multi-way similarity. In this paper, we formulate multi-way similarities by exploiting the theoretical foundation of kernel function. We show a theoretical connection between our formulation and hypergraph cut in two ways, generalizing both weighted kernel k-means and the heat kernel, by which we justify our formulation. We also provide a fast algorithm for spectral clustering. Our algorithm empirically shows better performance than existing graph and other heuristic modeling methods.
MoreTranslated text
Key words
Machine Learning (ML)
AI Read Science
Must-Reading Tree
Example
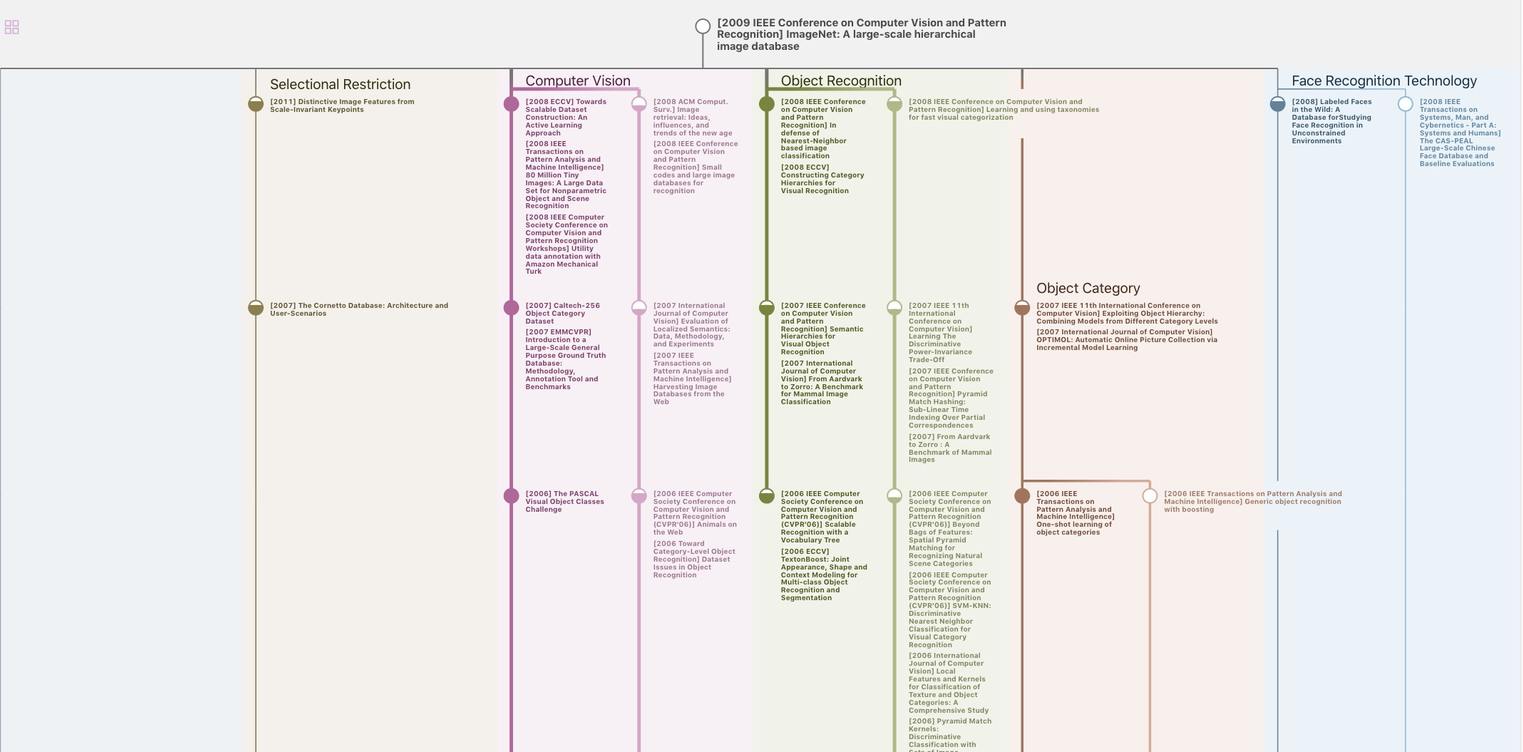
Generate MRT to find the research sequence of this paper
Chat Paper
Summary is being generated by the instructions you defined