Fully Adaptive Framework: Neural Computerized Adaptive Testing for Online Education.
AAAI Conference on Artificial Intelligence(2022)
摘要
Computerized Adaptive Testing (CAT) refers to an efficient and personalized test mode in online education, aiming to accurately measure student proficiency level on the required subject/domain. The key component of CAT is the "adaptive" question selection algorithm, which automatically selects the best suited question for student based on his/her current estimated proficiency, reducing test length. Existing algorithms rely on some manually designed and pre-fixed informativeness/uncertainty metrics of question for selections, which is labor-intensive and not sufficient for capturing complex relations between students and questions. In this paper, we propose a fully adaptive framework named Neural Computerized Adaptive Testing (NCAT), which formally redefines CAT as a reinforcement learning problem and directly learns selection algorithm from real-world data. Specifically, a bilevel optimization is defined and simplified under CAT's application scenarios to make the algorithm learnable. Furthermore, to address the CAT task effectively, we tackle it as an equivalent reinforcement learning problem and propose an attentive neural policy to model complex non-linear interactions. Extensive experiments on real-world datasets demonstrate the effectiveness and robustness of NCAT compared with several state-of-the-art methods.
更多查看译文
关键词
Domain(s) Of Application (APP),Data Mining & Knowledge Management (DMKM),Cognitive Modeling & Cognitive Systems (CMS)
AI 理解论文
溯源树
样例
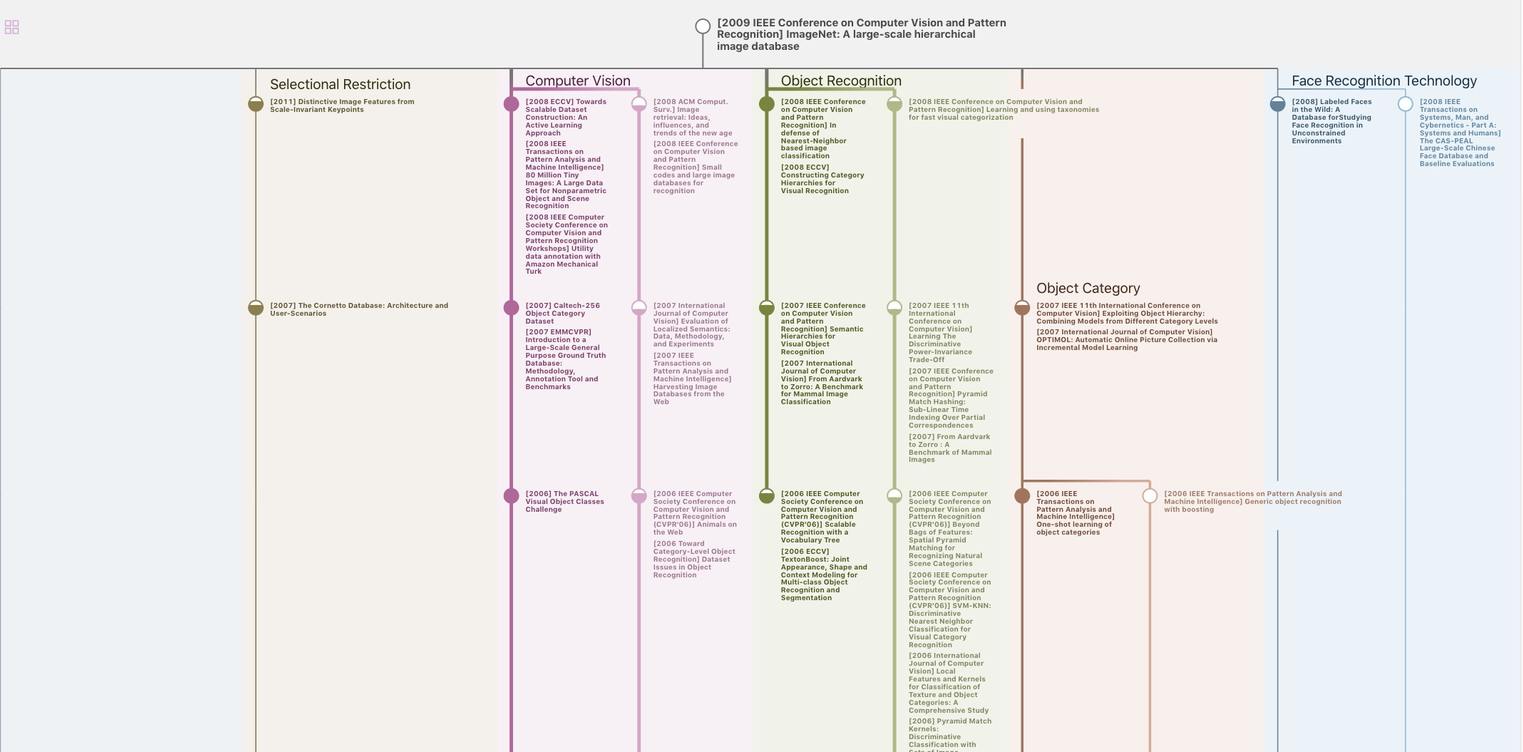
生成溯源树,研究论文发展脉络
Chat Paper
正在生成论文摘要