Self-Supervised Pre-training for Protein Embeddings Using Tertiary Structures.
AAAI Conference on Artificial Intelligence(2022)
摘要
The protein tertiary structure largely determines its interaction with other molecules. Despite its importance in various structure-related tasks, fully-supervised data are often time-consuming and costly to obtain. Existing pre-training models mostly focus on amino-acid sequences or multiple sequence alignments, while the structural information is not yet exploited. In this paper, we propose a self-supervised pre-training model for learning structure embeddings from protein tertiary structures. Native protein structures are perturbed with random noise, and the pre-training model aims at estimating gradients over perturbed 3D structures. Specifically, we adopt SE(3)-invariant features as model inputs and reconstruct gradients over 3D coordinates with SE(3)-equivariance preserved. Such paradigm avoids the usage of sophisticated SE(3)-equivariant models, and dramatically improves the computational efficiency of pre-training models. We demonstrate the effectiveness of our pre-training model on two downstream tasks, protein structure quality assessment (QA) and protein-protein interaction (PPI) site prediction. Hierarchical structure embeddings are extracted to enhance corresponding prediction models. Extensive experiments indicate that such structure embeddings consistently improve the prediction accuracy for both downstream tasks.
更多查看译文
关键词
Machine Learning (ML)
AI 理解论文
溯源树
样例
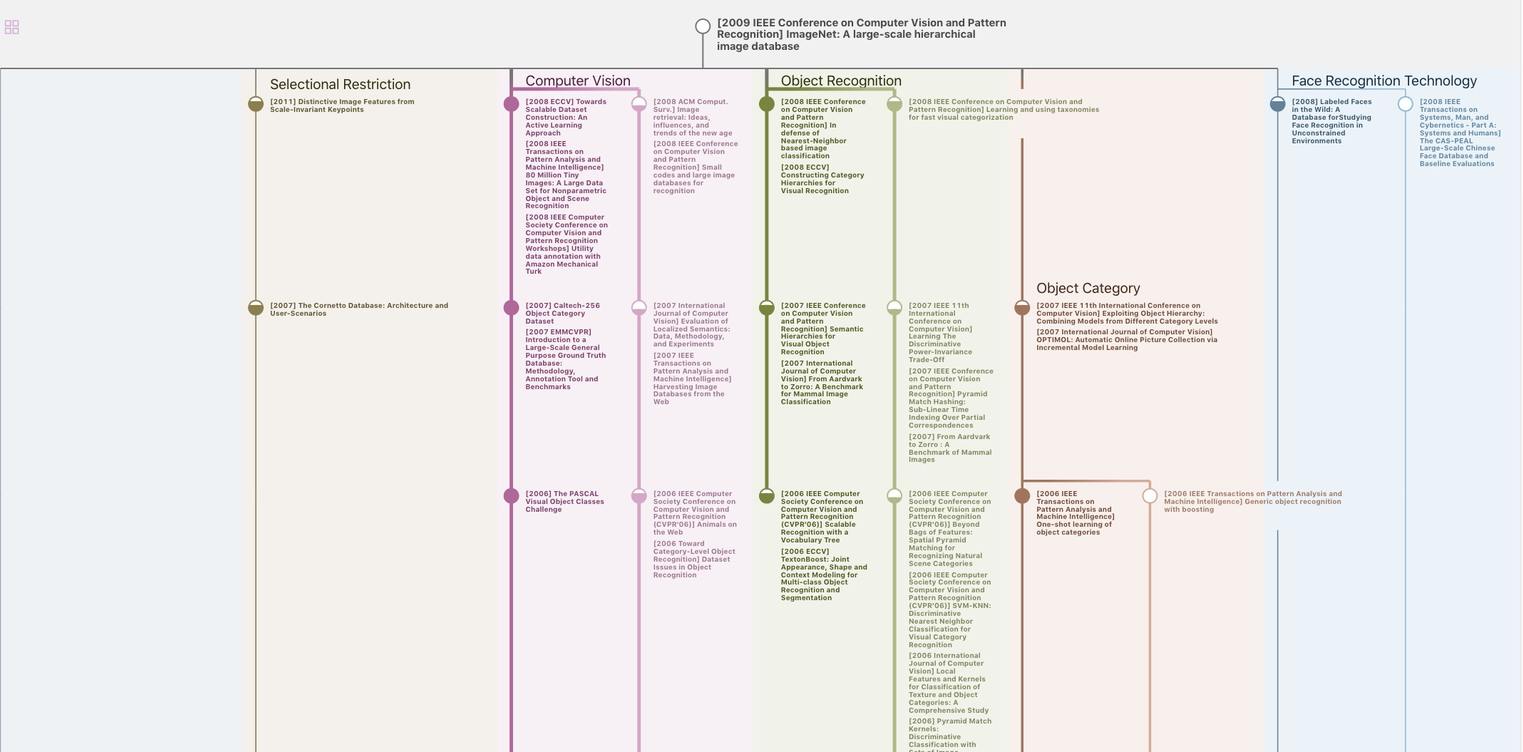
生成溯源树,研究论文发展脉络
Chat Paper
正在生成论文摘要