The Real-Time and Patient-Specific Prediction for Duration and Recovery Profile of Cisatracurium Based on Deep Learning Models
FRONTIERS IN PHARMACOLOGY(2022)
摘要
During general anesthesia, how to judge the patient's muscle relaxation state has always been one of the most significant issues for anesthesiologists. Train-of-four ratio (TOFR) monitoring is a standard method, which can only obtain static data to judge the current situation of muscle relaxation. Cisatracurium is a nondepolarizing benzylisoquinoline muscle relaxant. Real-time prediction of TOFR could help anesthesiologists to evaluate the duration and recovery profile of cisatracurium. TOFR of cisatracurium could be regarded as temporal sequence data, which could be processed and predicted using RNN based deep learning methods. In this work, we performed RNN, GRU, and LSTM models for TOFR prediction. We used transfer learning based on patient similarity derived from BMI and age to achieve real-time and patient-specific prediction. The GRU model achieved the best performance. In transfer learning, the model chosen based on patient similarity has significantly outperformed the model chosen randomly. Our work verified the feasibility of real-time prediction for TOFR of cisatracurium, which had practical significance in general anesthesia. Meanwhile, using the patient demographic data in transfer learning, our work could also achieve the patient-specific prediction, having theoretical value for the clinical research of precision medicine.
更多查看译文
关键词
deep learning-artificial neural network, Long Short-Term Memory, GRU (gated recurrent unit), temporal sequence analysis, general anesthesia
AI 理解论文
溯源树
样例
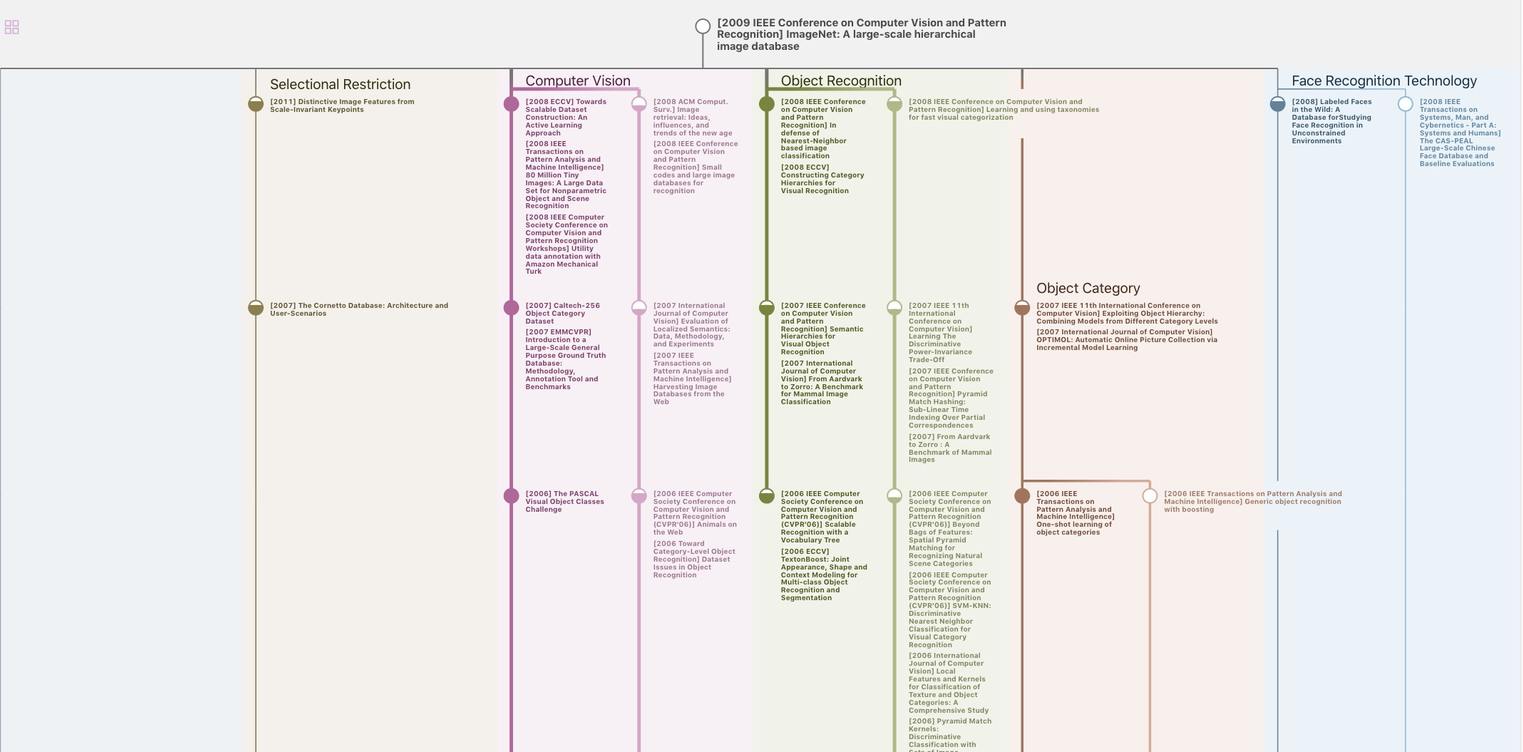
生成溯源树,研究论文发展脉络
Chat Paper
正在生成论文摘要