Multi-scale feature aggregation network for Image super-resolution
Applied Intelligence(2021)
摘要
For single image super-resolution technology, Convolutional Neural Network (CNN) has an excellent capability to improve reconstruction results. However, the existing CNN-based SR methods maintain high-quality reconstruction with excessive amounts of parameters and very deep network structures, which not only leads to higher requirements for computational resource and memory storage but also makes it difficult to apply resource-constrained devices. To solve these problems, a multi-scale feature aggregation network (MFAN) is proposed in this paper. In the MFAN, global dual-path is put into use to forward the feature information in low resolution space and medium resolution space respectively. For the sake of enhancing the ability of MFAN feature extraction, we come up a with multi-scale feature extraction module (MSFE), which also contains a multi-path structure. This module is embedded in each global path for image feature extraction. Through the effective combination of global path and MSFE, multi-scale information is extracted and enhanced step by step. Moreover, we design an inter-scale feature projection module (ISFP) based on the back-projection mechanism to effectively integrate the multi-scale feature information from MSFE. Extensive experiments show that the proposed MFAN has achieved competitive results in the qualitative and quantitative evaluation.
更多查看译文
关键词
Super-resolution,Multi-path learning,Multi-scale,Convolutional neural network
AI 理解论文
溯源树
样例
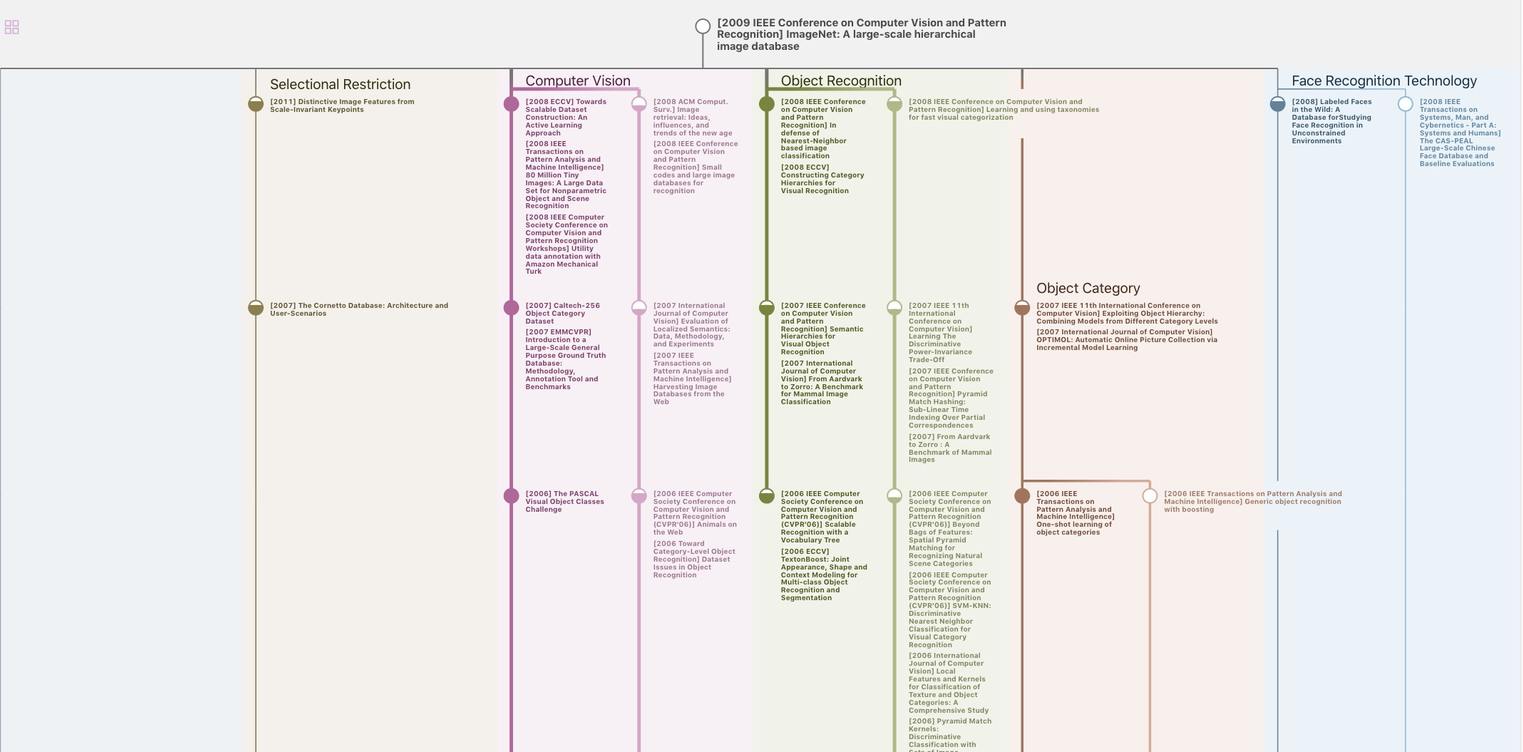
生成溯源树,研究论文发展脉络
Chat Paper
正在生成论文摘要