Self-Supervised Bulk Motion Artifact Removal in Optical Coherence Tomography Angiography
IEEE Conference on Computer Vision and Pattern Recognition(2022)
摘要
Optical coherence tomography angiography (OCTA) is an important imaging modality in many bioengineering tasks. The image quality of OCTA, however, is often degraded by Bulk Motion Artifacts (BMA), which are due to micromotion of subjects and typically appear as bright stripes surrounded by blurred areas. State-of-the-art methods usually treat BMA removal as a learning-based image inpainting problem, but require numerous training samples with nontrivial annotation. In addition, these methods discard the rich structural and appearance information carried in the BMA stripe region. To address these issues, in this paper we propose a self-supervised content-aware BMA removal model. First, the gradient-based structural information and appearance feature are extracted from the BMA area and injected into the model to capture more connectivity. Second, with easily collected defective masks, the model is trained in a self-supervised manner, in which only the clear areas are used for training while the BMA areas for inference. With the structural information and appearance feature from noisy image as references, our model can remove larger BMA and produce better visualizing result. In addition, only 2D images with defective masks are involved, hence improving the efficiency of our method. Experiments on OCTA of mouse cortex demonstrate that our model can remove most BMA with extremely large sizes and inconsistent intensities while previous methods fail.
更多查看译文
关键词
Medical,biological and cell microscopy
AI 理解论文
溯源树
样例
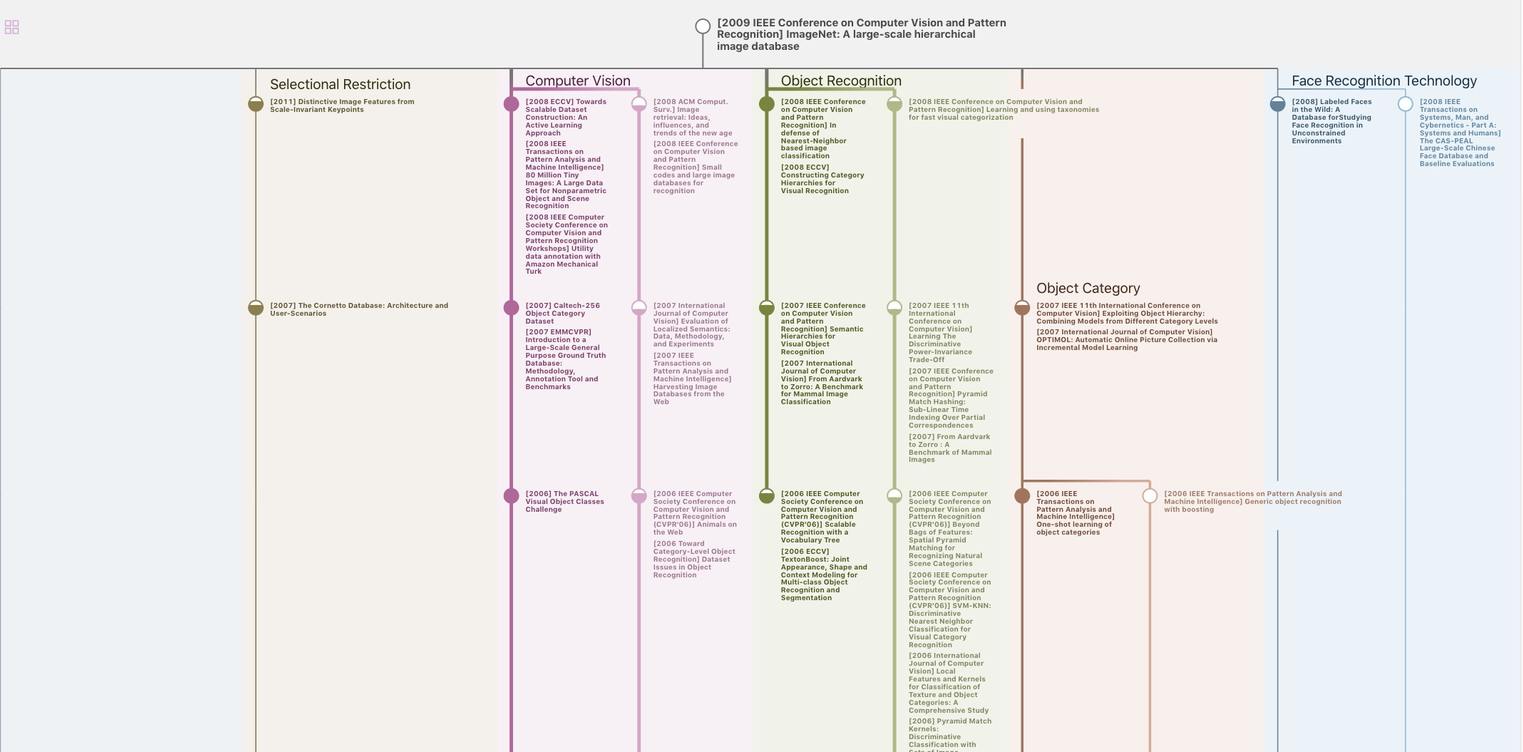
生成溯源树,研究论文发展脉络
Chat Paper
正在生成论文摘要