Path of Destruction: Learning an Iterative Level Generator Using a Small Dataset
2022 IEEE Symposium Series on Computational Intelligence (SSCI)(2022)
摘要
We propose a new procedural content generation method which learns iterative level generators from a dataset of existing levels. The Path of Destruction method, as we call it, views level generation as repair; levels are created by iteratively repairing from a random starting level. The first step is to generate an artificial dataset from the original set of levels by introducing many different sequences of mutations to existing levels. In the generated dataset, features are observations of destroyed levels and targets are the specific actions that repair the mutated tile in the middle of the observations. Using this dataset, a convolutional network is trained to map from observations to their respective appropriate repair actions. The trained network is then used to iteratively produce levels from random starting maps. We demonstrate this method by applying it to generate unique and playable tile-based levels for several 2D games (Zelda, Danger Dave, and Sokoban) and vary key hyperparameters.
更多查看译文
关键词
procedural content generation,neural networks,supervised learning,games,level generation,data augmentation
AI 理解论文
溯源树
样例
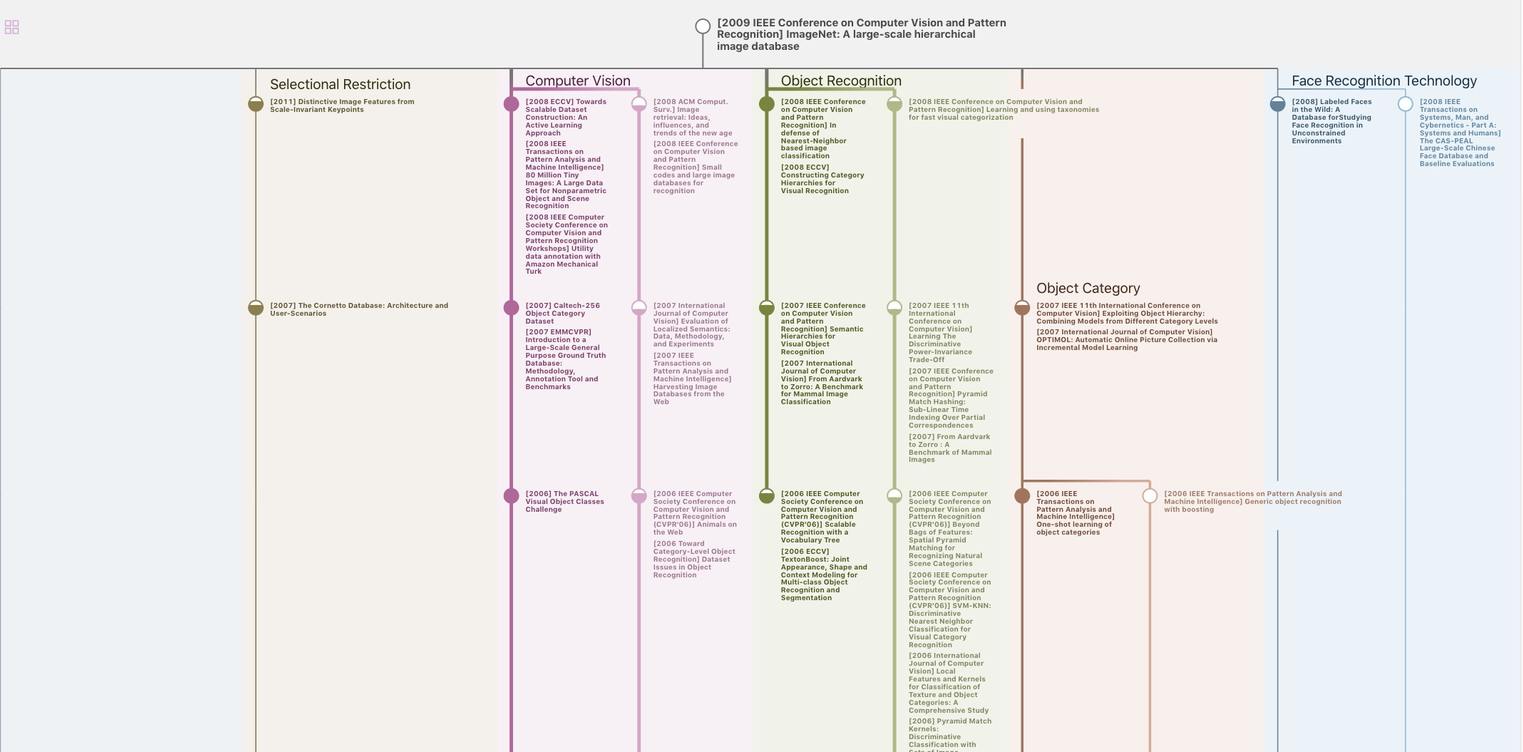
生成溯源树,研究论文发展脉络
Chat Paper
正在生成论文摘要