A lifting approach to learning-based self-triggered control with Gaussian processes
arxiv(2024)
摘要
This paper investigates the design of self-triggered control for networked control systems (NCS), where the dynamics of the plant are initially unknown. Given the nature of the self-triggered control where state measurements are sent to the controller a-periodically, our proposal involves augmenting the continuous-time dynamics to a novel dynamical model that incorporates inter-event time as a supplemental input. Then, this new model is studied through Gaussian processes (GP) regression. Additionally, we propose a learning-based approach where a self-triggered controller is formulated by minimizing a cost function for the newly learned model, ensuring consideration of inter-sample behavior. The usage of the lifting approach facilitates the application of gradient-based policy updates, allowing for the effective optimization of both control and communication policies. Finally, we provide a numerical simulation to illustrate the effectiveness of the proposed approach.
更多查看译文
关键词
Event-triggered and self-triggered control,Gaussian process regression,optimal control
AI 理解论文
溯源树
样例
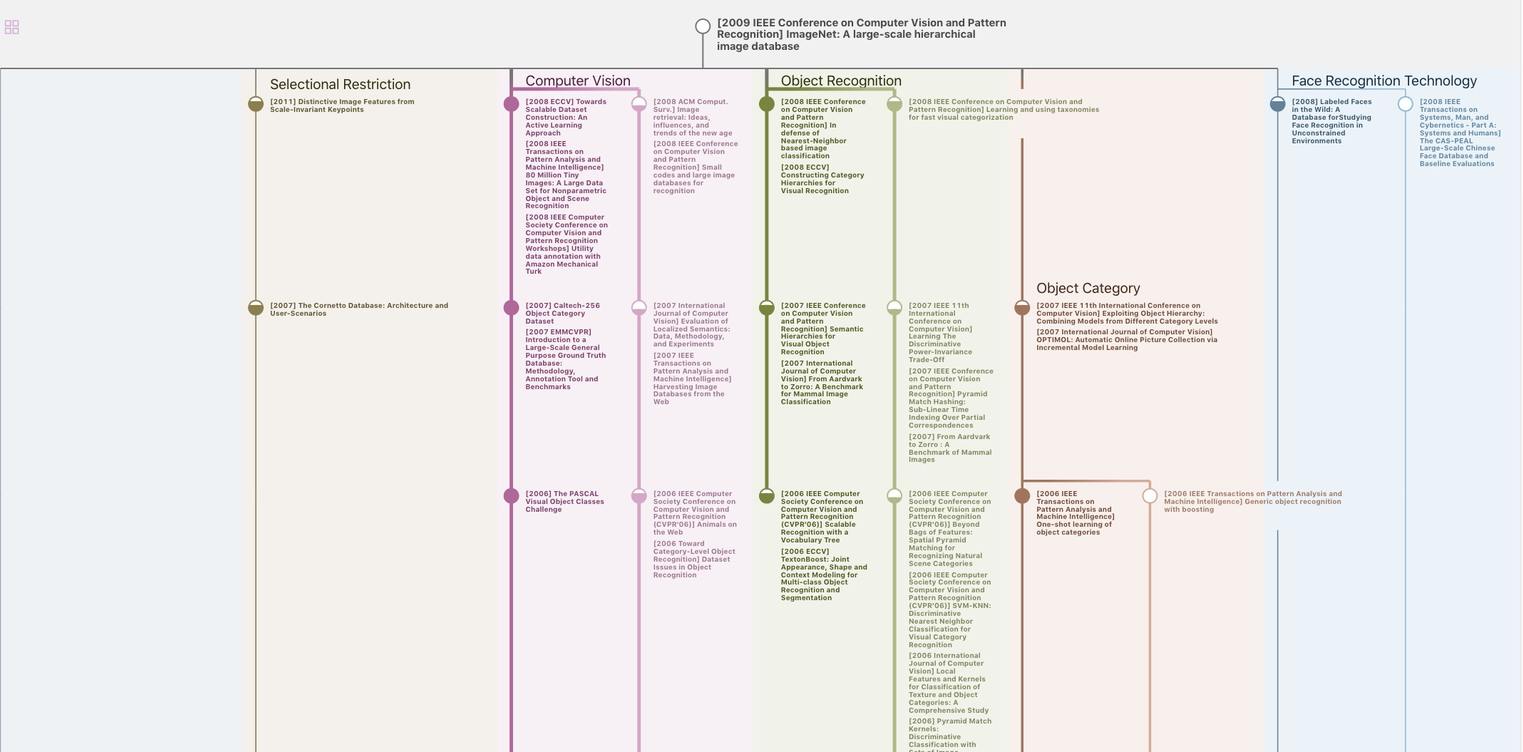
生成溯源树,研究论文发展脉络
Chat Paper
正在生成论文摘要