An Efficient Smoothing and Thresholding Image Segmentation Framework with Weighted Anisotropic-Isotropic Total Variation
Communications on Applied Mathematics and Computation(2024)
摘要
In this paper, we design an efficient, multi-stage image segmentation framework that incorporates a weighted difference of anisotropic and isotropic total variation (AITV). The segmentation framework generally consists of two stages: smoothing and thresholding, thus referred to as smoothing-and-thresholding (SaT). In the first stage, a smoothed image is obtained by an AITV-regularized Mumford-Shah (MS) model, which can be solved efficiently by the alternating direction method of multipliers (ADMMs) with a closed-form solution of a proximal operator of the ℓ _1 -αℓ _2 regularizer. The convergence of the ADMM algorithm is analyzed. In the second stage, we threshold the smoothed image by K -means clustering to obtain the final segmentation result. Numerical experiments demonstrate that the proposed segmentation framework is versatile for both grayscale and color images, efficient in producing high-quality segmentation results within a few seconds, and robust to input images that are corrupted with noise, blur, or both. We compare the AITV method with its original convex TV and nonconvex TV ^p (0
更多
查看译文
关键词
Image segmentation,Non-convex optimization,Mumford-Shah (MS) model,Alternating direction method of multipliers (ADMMs),Proximal operator
AI 理解论文
溯源树
样例
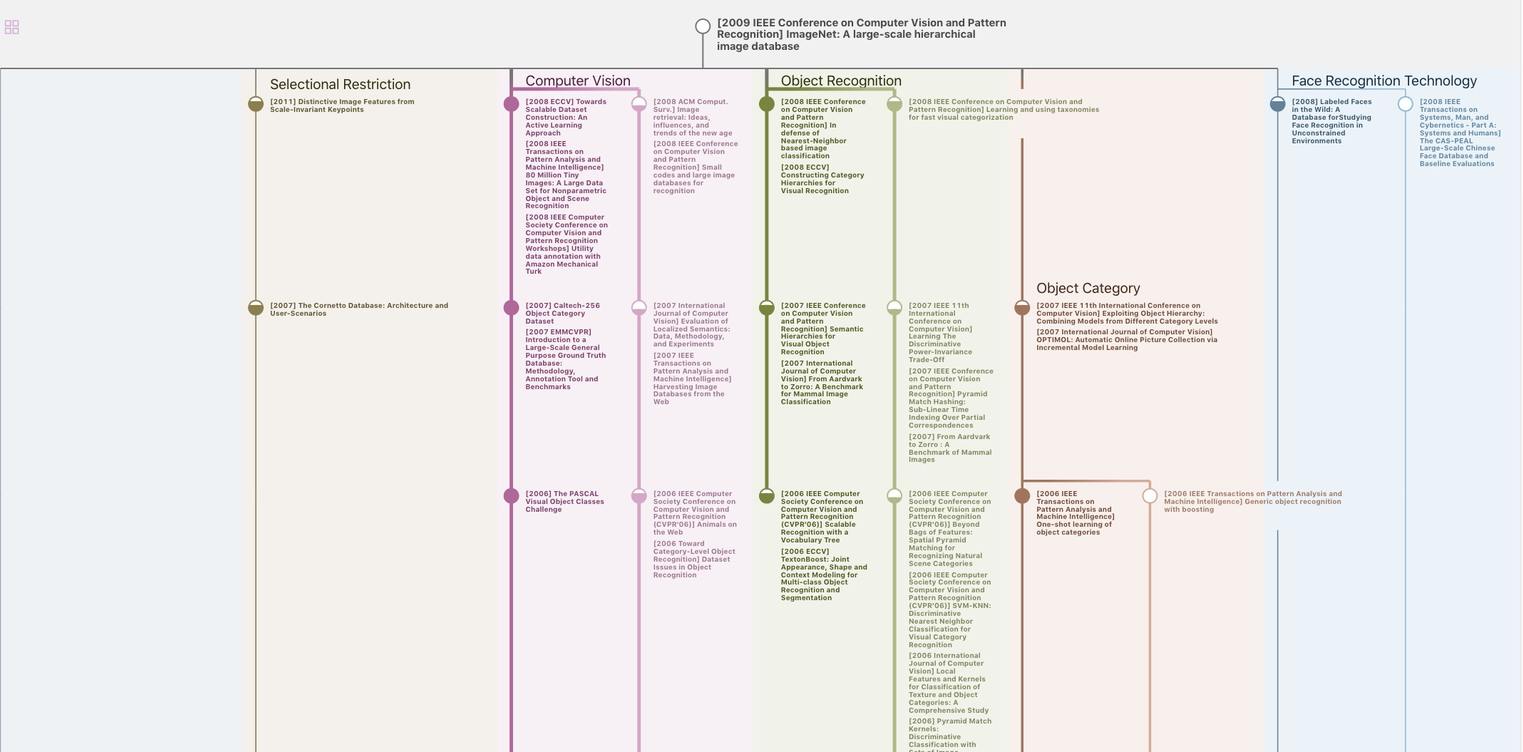
生成溯源树,研究论文发展脉络
Chat Paper
正在生成论文摘要