Multi-task safe reinforcement learning for navigating intersections in dense traffic
JOURNAL OF THE FRANKLIN INSTITUTE-ENGINEERING AND APPLIED MATHEMATICS(2023)
摘要
Multi-task intersection navigation, which includes unprotected turning left, turning right, and going straight in heavy traffic, remains a difficult task for autonomous vehicles. For the human driver, negotiation skills with other interactive vehicles are the key to guaranteeing safety and efficiency. However, it is hard to balance the safety and efficiency of the autonomous vehicle for multi-task intersection navigation. In this paper, we formulate a multi-task safe reinforcement learning framework with social attention to improve the safety and efficiency when interacting with other traffic participants. Specifically, the social attention module is used to focus on the states of negotiation vehicles. In addition, a safety layer is added to the multi-task reinforcement learning framework to guarantee safe negotiation. We deploy experiments in the simulators SUMO, which has abundant traffic flows, and CARLA, which has high-fidelity vehicle models. Both show that the proposed algorithm improves safety while maintaining stable traffic efficiency for the multi-task intersection navigation problem. More details and demonstrations are available at https:// github.com/ liuyuqi123/ SAT.(c) 2022 The Franklin Institute. Published by Elsevier Ltd. All rights reserved.
更多查看译文
关键词
traffic,intersections,multi-task
AI 理解论文
溯源树
样例
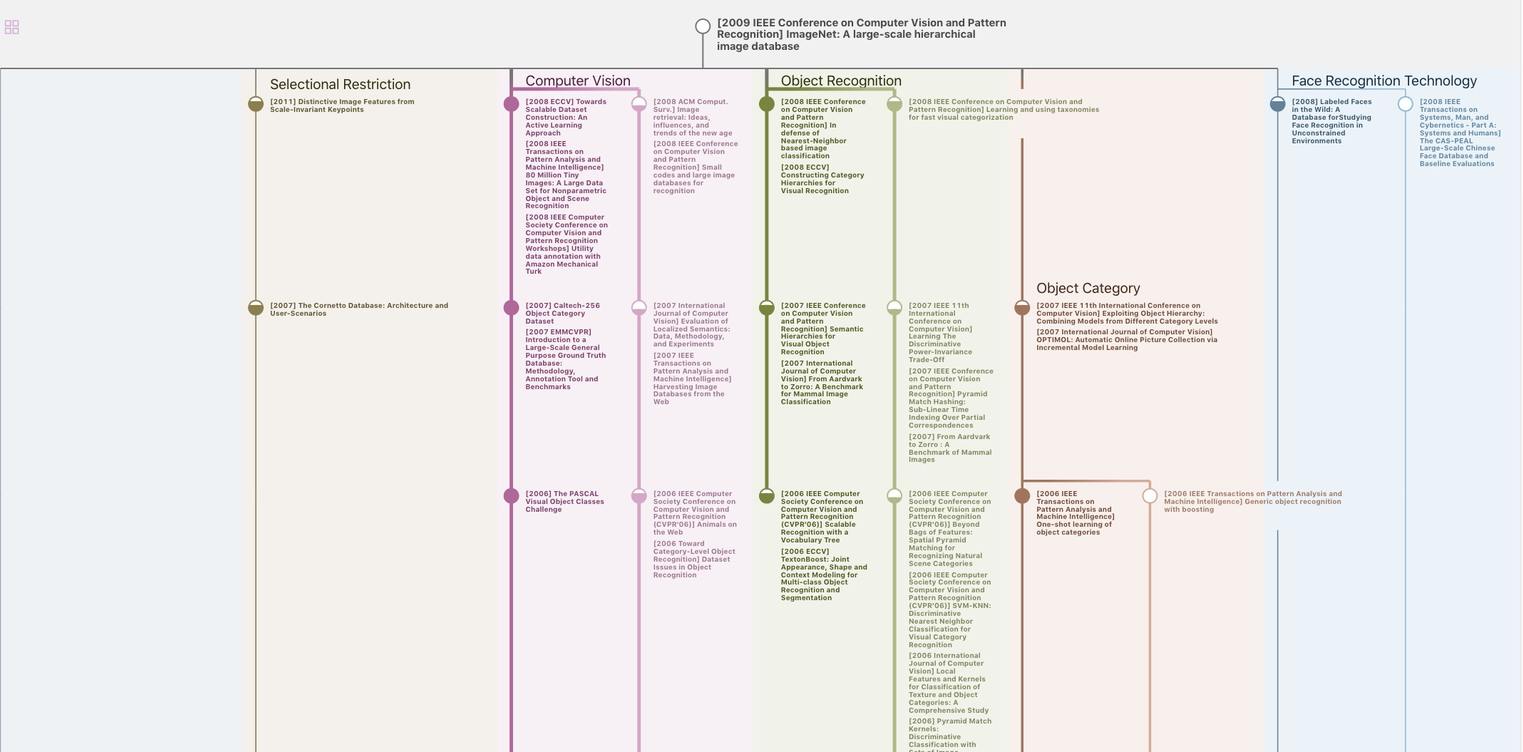
生成溯源树,研究论文发展脉络
Chat Paper
正在生成论文摘要