Multi-task unscented Kalman inversion (MUKI): a derivative-free joint inversion framework and its application to joint inversion of geophysical data
arXiv (Cornell University)(2022)
摘要
In the geophysical joint inversion, the gradient and Bayesian Markov Chain Monte Carlo (MCMC) sampling-based methods are widely used owing to their fast convergences or global optimality. However, these methods either require the computation of gradients and easily fall into local optimal solutions, or cost much time to carry out the millions of forward calculations in a huge sampling space. Different from these two methods, taking advantage of the recently developed unscented Kalman method in computational mathematics, we extend an iterative gradient-free Bayesian joint inversion framework, i.e., Multi-task unscented Kalman inversion (MUKI). In this new framework, information from various observations is incorporated, the model is iteratively updated in a derivative-free way, and a Gaussian approximation to the posterior distribution of the model parameters is obtained. We apply the MUKI to the joint inversion of receiver functions and surface wave dispersion, which is well-established and widely used to construct the crustal and upper mantle structure of the earth. Based on synthesized and real data, the tests demonstrate that MUKI can recover the model more efficiently than the gradient-based method and the Markov Chain Monte Carlo method, and it would be a promising approach to resolve the geophysical joint inversion problems.
更多查看译文
关键词
joint
AI 理解论文
溯源树
样例
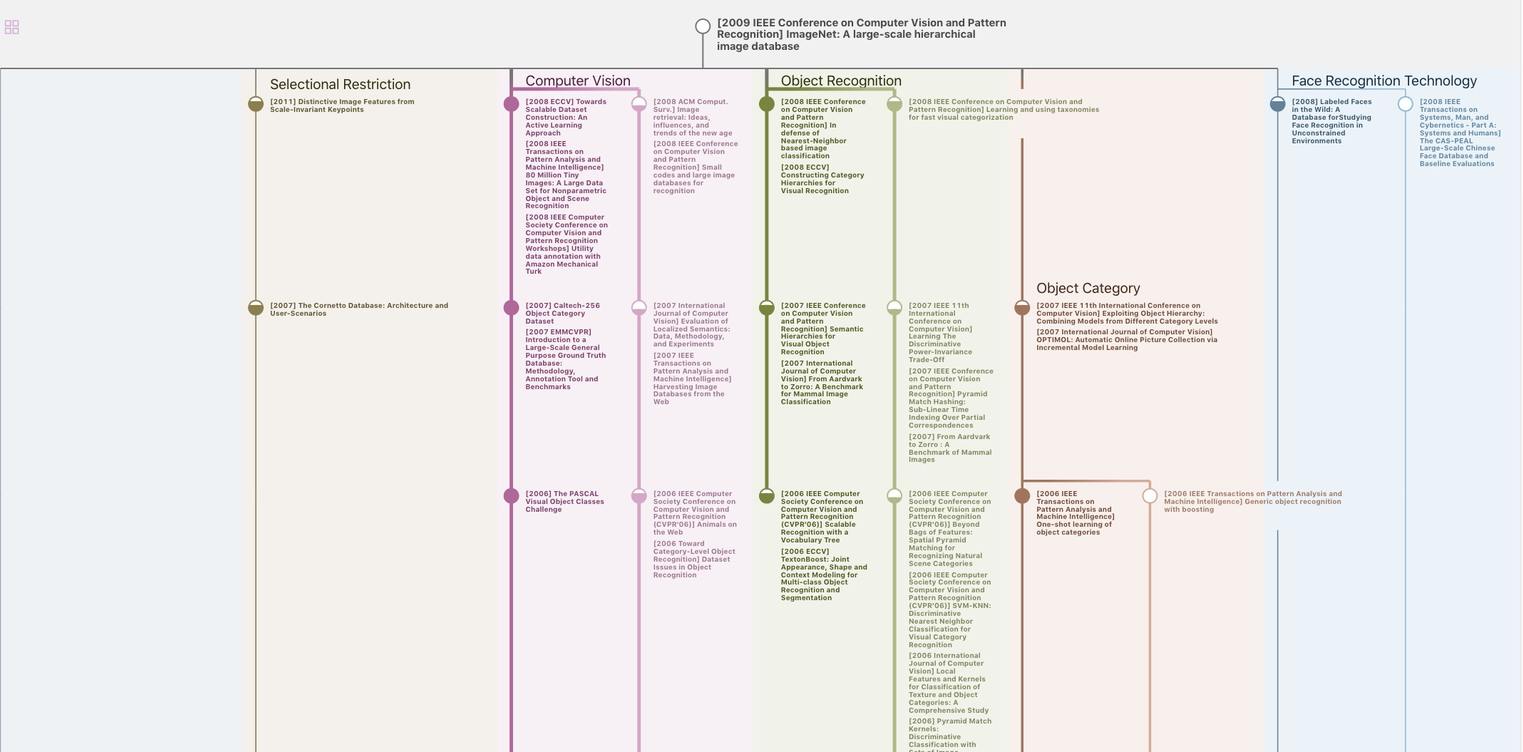
生成溯源树,研究论文发展脉络
Chat Paper
正在生成论文摘要