Correction: Spatio-Temporal Outdoor Lighting Aggregation on Image Sequences Using Transformer Networks
arxiv(2023)
摘要
In this work, we focus on outdoor lighting estimation by aggregating individual noisy estimates from images, exploiting the rich image information from wide-angle cameras and/or temporal image sequences. Photographs inherently encode information about the lighting of the scene in the form of shading and shadows. Recovering the lighting is an inverse rendering problem and as that ill-posed. Recent research based on deep neural networks has shown promising results for estimating light from a single image, but with shortcomings in robustness. We tackle this problem by combining lighting estimates from several image views sampled in the angular and temporal domains of an image sequence. For this task, we introduce a transformer architecture that is trained in an end-2-end fashion without any statistical post-processing as required by previous work. Thereby, we propose a positional encoding that takes into account camera alignment and ego-motion estimation to globally register the individual estimates when computing attention between visual words. We show that our method leads to improved lighting estimation while requiring fewer hyperparameters compared to the state of the art.
更多查看译文
关键词
Lighting estimation,Spatio-temporal filtering,Positional encoding,Transformer
AI 理解论文
溯源树
样例
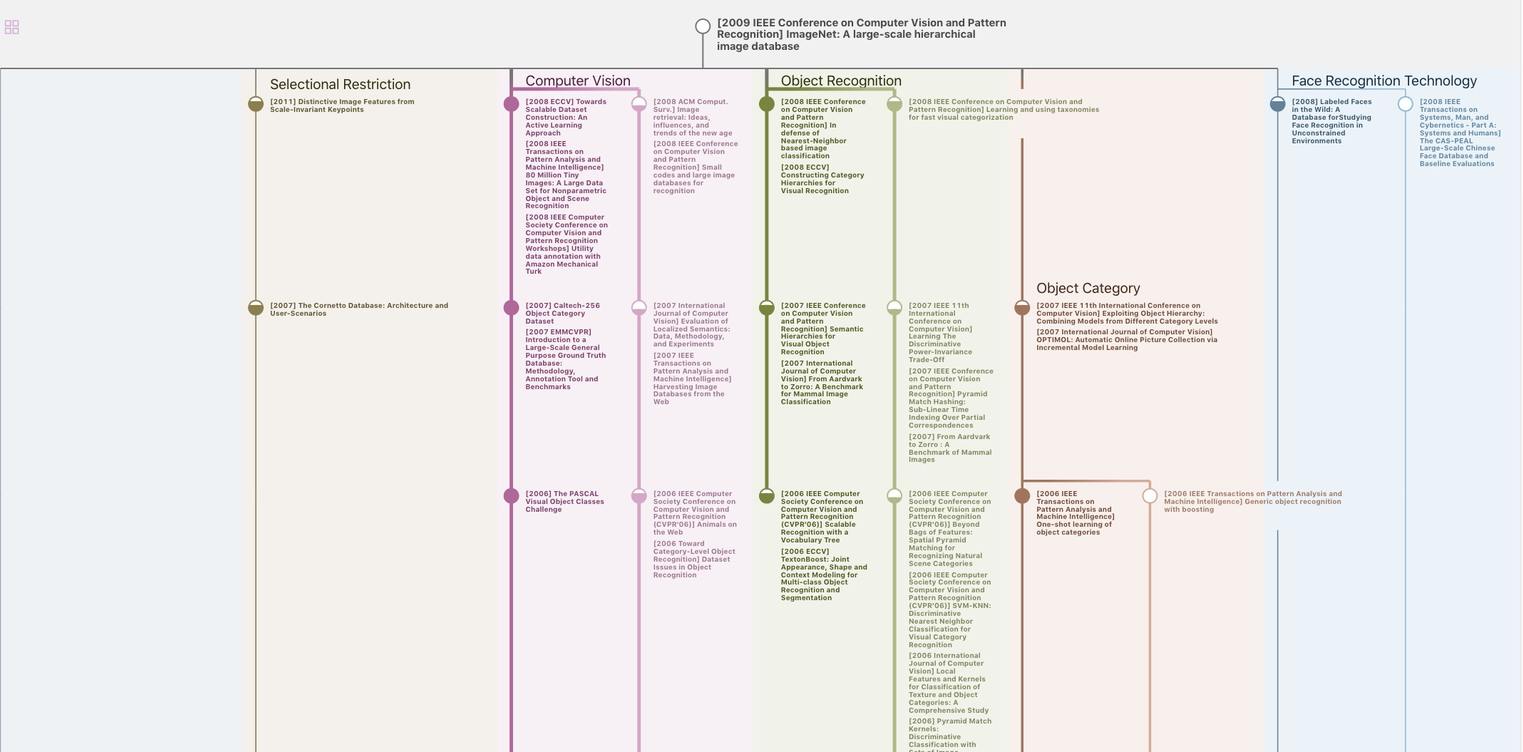
生成溯源树,研究论文发展脉络
Chat Paper
正在生成论文摘要