Automated galaxy-galaxy strong lens modelling: no lens left behind
arxiv(2023)
摘要
The distribution of dark and luminous matter can be mapped around galaxies that gravitationally lens background objects into arcs or Einstein rings. New surveys will soon observe hundreds of thousands of galaxy lenses, and current, labour-intensive analysis methods will not scale up to this challenge. We instead develop a fully automatic, Bayesian method which we use to fit a sample of 59 lenses imaged by the Hubble Space Telescope in uniform conditions. We set out to \textit{leave no lens behind} and focus on ways in which automated fits fail in a small handful of lenses, describing adjustments to the pipeline that allows us to infer accurate lens models. Our pipeline ultimately fits {\em all} 59 lenses in our sample, with a high success rate key because catastrophic outliers would bias large samples with small statistical errors. Machine Learning techniques might further improve the two most difficult steps: subtracting foreground lens light and initialising a first, approximate lens model. After that, increasing model complexity is straightforward. We find a mean $\sim1\%$ measurement precision on the measurement of the Einstein radius across the lens sample which {\em does not degrade with redshift} up to at least $z=0.7$ -- in stark contrast to other techniques used to study galaxy evolution, like stellar dynamics. Our \texttt{PyAutoLens} software is open source, and is also installed in the Science Data Centres of the ESA Euclid mission.
更多查看译文
关键词
gravitational lensing: strong,software: data analysis,galaxies: fundamental parameters,dark matter
AI 理解论文
溯源树
样例
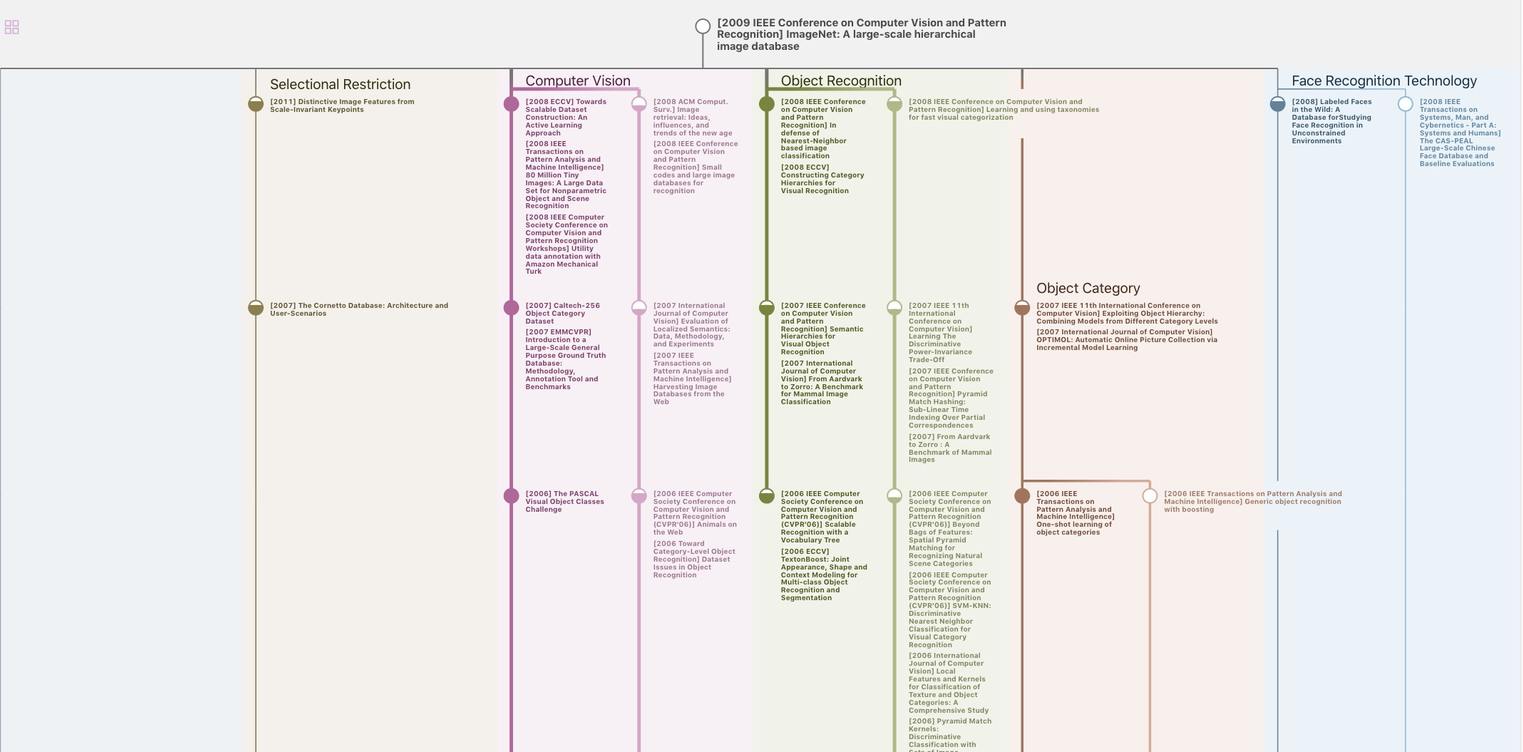
生成溯源树,研究论文发展脉络
Chat Paper
正在生成论文摘要